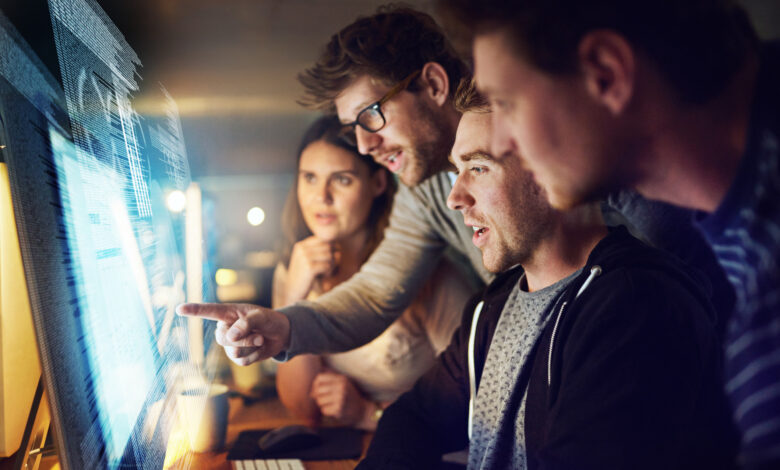
AI everywhere! Artificial intelligence (AI) is increasingly becoming an integral component of business operations, weaving itself into the fabric of every industry. Its influence is broad and deep, with almost choreographed adoption from companies of all shapes, sizes, and sectors across the globe. In fact, Gartner predicts that over three-quarters of organizations will move from testing AI technologies to embedding them into their daily operations, business processes, and customer workflows by the end of 2024.
Amid this rush to adopt, I often find myself navigating conversations between optimists who might underestimate the hurdles and critics who need to hear real-world success stories before entertaining future benefits. Striking a balance in perspectives is key. My emphasis is on uncovering meaningful, Enterprise Value-generating opportunities, and tackling the real challenges that AI introduces to the business landscape.
As part of that focus, we must broaden our view of AI beyond a mechanism for reducing labor costs through automation. AI lowers the cost of prediction, so it’s important to view this technology as a tool that amplifies human skills and boosts overall workforce capabilities. This perspective steers the conversation towards continuous improvement, growth, and forward movement, which in turn energizes the organization.
Critical Considerations in Selecting AI Offerings
Many firms struggle to deploy AI in production. An example we see time and time again is in customer experience (CX) and the customer onboarding journey. In these workflows, real-time responsiveness is critical.
Let’s say you’re on Amazon.com, for instance, and you’re about to check out with five items in your cart when suddenly, a buffering icon pops up on your screen and a sixth item is presented as a recommendation 30 seconds later—you’ve already lost the customer. Things need to happen in the blink of an eye, and the experience needs to feel part of a natural flow.
In other words, understanding the response time of your GenAI workflow is key, and this is something that can often materialize as you ramp up the amount of data feeding your decision engine or the number of consumers of your AI-ready context.
Gartner predicts that 90% of enterprise GenAI deployments will slow down as costs exceed value – this suggests two major points: enterprises aren’t understanding Return on Investment (ROI,) and they’re not controlling Total Cost of Ownership (TCO.) There’s a growing faction of startup companies who’ve openly sourced technology in the GenAI ecosystem that works incredibly well when dealing with a couple hundred unstructured documents.
But when you ramp this up to hundreds of thousands of documents, the open-source system becomes so bloated that the running costs are prohibitive. It’s a similar story on the usage side—you may insert GenAI into a workflow that works for a small number of users, but what happens when you unleash the workflow to thousands or tens of thousands of users? Spoiler alert: it does not end well.
Failure to effectively transition AI workflows into production can have profound repercussions for a business. Beyond the clear losses in time, money, and effort, such failures can result in competitive disadvantages, and reputational damage and hinder future innovation initiatives. My recommended approach is a classic case of going slow to go fast—take your time to pick vendors and technologies that will scale with your ambition.
The Key to Successful AI Adoption
Successful AI adoption hinges on a multifaceted approach that addresses critical organizational, cultural, and technological considerations. Success with GenAI is a three-legged race between data, tools, and people, navigating hurdles, like policies, privacy, and regulation, to deliver outcomes that move the needle for the enterprise:
- Cross-Departmental Collaboration: The urgency for AI strategic integration has led to increased pressures on CEOs from their boards, creating a complex hierarchy involving CFOs, CTOs, CDOs, and CIOs, each exploring how to weave AI into their operations effectively. Successful companies realize that AI is a team sport. They’ve either created a virtual team that runs AI projects across each of those C-Suite personas or a Chief AI Officer (CAIO) role, which is something we’re seeing pop up more frequently.
- Technological Alignment: In ensuring AI technologies align with corporate infrastructure, it’s crucial to integrate seamlessly with existing systems, ensure scalability, uphold security and compliance standards, and provide customization for unique business needs. Emphasizing future-proofing, vendor support, and the ecosystem, alongside regular performance monitoring, ensures AI solutions remain relevant, secure, and efficient, adapting to evolving business landscapes and technological advancements.
- Data Readiness: Data readiness also tends to be a big blocker, so your data must be in AI consumable format. This typically means you have a data fabric, mesh, or lake in place so that your data is ready to access. You may have a messaging layer sitting between each of the data assets, but ultimately, the data is in a format that can be consumed by your AI products.
High performers in AI adoption – true AI-first companies – have already embraced these elements and see increased revenue by radically improving their existing products and services, along with creating new offerings in record time.
For those just starting their journey, I encourage them to leverage these core practices. In time, you’ll notice the value that AI is generating across the entire organization, enabling you to avoid both the risk and high costs of poorly architectured technology and further investments in continued training and education for your employees to manage their AI applications.
Charting the Course Ahead
There’s a common expression that comes to mind when I think about the key to successful AI adoption: “If you want to go fast, go alone. If you want to go far, go together.”
A lot of enterprises are trying to take baby steps, leveraging open-source technology and small scripts they find on the Internet for free. It’s very developer-led, and they’re using that to validate some use cases, but they’re not thinking about the future state – they aren’t asking the hard questions about what success will demand in terms of technology.
They’re not talking about the day-zero considerations regarding data, data governance, and data policy. They’re not thinking about future applications and how to scale these use cases for greater volumes.
If you take away one thing from this, please let it be that going alone is not the answer – lean on experienced industry practitioners to give you a boost— they’ll help you avoid the chutes and find the ladders along the GenAI board game. The landscape is shifting at unprecedented rates, so being able to partner with a company that will be as nimble and malleable as the landscape is key.