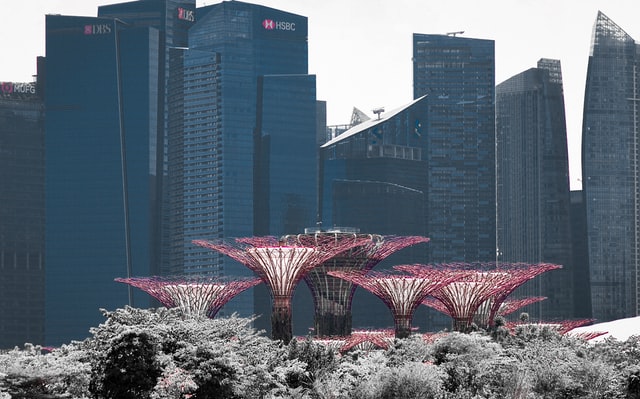
The problem: lack of data excellence
AI in banking is becoming a bigger issue and banks are facing multiple challenges when investing in AI. Some of these challenges include:
-> Legacy IT
-> Data Silos
-> ‘Spaghetti’ Software
-> Complex Privacy Regulations
-> Stringent Compliance Requirements
-> Cybersecurity
-> Shortage of Available AI Talent
-> Outdated User Interfaces
-> Heritage of Complexity as Opposed to Simplicity
-> Relatively Older Clientele
-> Legacy Channels
-> Lack of Data Ecosystems and Open Platformification
As a result of all this, banks are typically near the ‘no go zone’ of the matrix of data excellence:
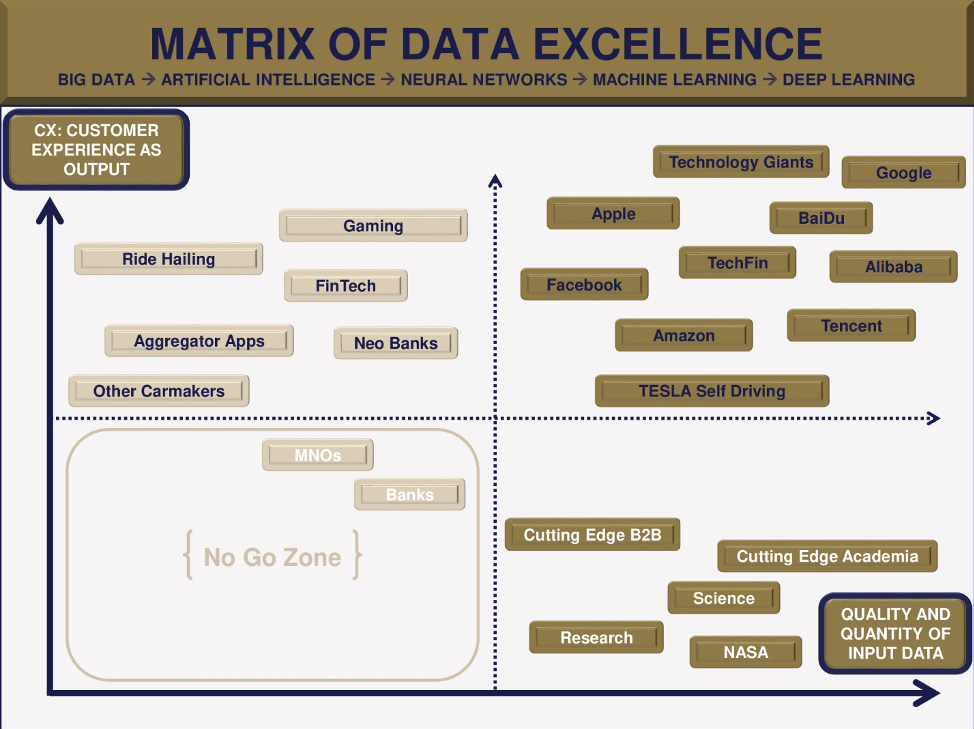
Being near the ‘no go zone’ of the matrix of data excellence is a great problem because data is the input of AI.
There is a cap limiting AI in banking success and this cap is exactly the quantity and quality of input data multiplied by the business value of customer experience as an output.
In the case of large, traditional, incumbent, bricks and mortar banks both components of the multiplication are typically weak.
Solutions: 20 ways banks can get AI right
1. Design data-focused products for your AI in banking strategy
The traditional process of product design in banking is focused on risks.
Data is collected in a way that primarily serves risk-management purposes.
While risk-management has to remain intact products have to be designed with smart, valuable, rich, structured, real-time data in mind.
Products have to be designed in a way that they provide the legal access to data from day 1 which later down the road can be turned into customer insights and superior customer experience.
2. Explicit, constantly visible client consent
Data privacy is a constant issue.
Clients have to be asked for permissions constantly and explicitly.
When you ask for data permissions from your clients, offer something in return: explain how your client will get something tangible and valuable in return.
Always link additional data consent to additional value, functionalities, insight, and services for the client.
3. Quest for hyper-personalisation
Try to create ‘segments of one’ through the collection of the volume and variety of data that can empower you to pursue automated hyper-personalisation.
When clients feel that your service is sensitive and responsive to their individual preferences, they will be happy to share more and more information with you.
Think about a situation where you offer the client the chance to offer money to a charity through ‘rounding prices up’.
For example, the client purchases a coffee for £1.79 and you offer to have the remaining £0.21 put into a charity pot, which, after the client has collected £20, this pot can be given to a charity, which you, as a bank, will match with an equal donation.
Let’s say the client is a paediatrician.
In this case, the three potential charities the client can choose from should be about health, children, and medical research.
Another client is a music teacher, in which case the three choices can be related to classical music, early talent, and education.
These elements of hyper-personalisation have to be fully automated and ideally be propelled for AI in banking to be a success.
4. Blend data and design
Think about the difference between a 22 years old female nurse living in a village and a 55 years old male bus driver living in a large city.
Is there a potential difference in the ideal colours and patterns they prefer to see as the design of an app or an online banking interface?
Chances are, yes.
Now, start to add purchase data to the equation.
Think about the potential blending of data and design!
This is a way that using AI in banking can give you an edge while improving customer experience.
5. Use geolocation in a smart way to improve chances of AI in banking working for you
Most mobile banking apps ask for geolocational data and most users do share this information with their banks.
Think about a client standing within 2 meters of the distance of an ATM for more than one minute!
Send an account balance automatically.
Think about a client walking inside the premises of a large car dealership for more than 20 minutes.
Send a leasing and car loan value proposition automatically.
And these are just the humble first steps.
There is a great potential in turning your client’s geolocational data into added value for them.
6. For AI in banking to work you need to cooperate with data-rich external partners
Design, build, and offer as many opportunities and incentives for your clients to share third party data with you as you can.
The more data from the more sources you can channel into your apps and interfaces the more value you can create for your clients.
Think about clients sharing e-commerce data, travel data, ride-sharing data, social media data, or chat data with you.
In any case, if you have ways to turn this data into the immediate value for your clients they (at least a significant chunk of them) will be happy to share their data with you.
You just need to establish safe, formal, and legally safeguarded cooperation ‘with the other end of the API’.
On the flip side of the coin, also actively encourage your clients to share external data via APIs with you and grab every opportunity to run superior social media plugins.
Clients love data-synergies when an ecosystem already holds their data and they can just automatically share this with ‘one-click’ and thereby gain quality access to something else.
7. Heavily incentivise your clients to write reviews, testimonials, and participate in your online community
Reviews and testimonials are primary sources of key data, if and when used well.
But there is a second reason to incentivise your clients heavily to be active this way: a video testimonial is nowadays more valuable among Gen-Z customers than almost any paid media.
Earn data, feedback, and publicity this way and let your active clients enjoy heavy benefits in return.
Research shows that when reacted to professionally and positively, a negative and critical review is even more valuable than a positive one.
8. Develop processes for ‘data dripping’
You need access to good data and a lot of data from your clients for AI in banking to be effective.
Yet, Millennials and Gen-Z are sometimes dubbed as ‘the instant generation’: they want things instantly and with one click.
How can you get a lot of detailed client data and information if your clients want to onboard and access your services instantaneously?
The answer is that you have to establish fine, smooth, conscious, and well-designed processes of ‘data dripping’: asking for a little drip of new data, a little crumb of new information from time to time.
This makes your hunger and quest for ‘a lot of information’ compatible with your new target group of Millennials and Gen-Z.
The technique of ‘data dripping’ is a good example to demonstrate the fact that taking traditional products and processes and creating an exact digital version of them is a mistake.
When you ask for information and data from your client in a physical environment, it is better to ask for as much as possible at once.
In a mobile online paradigm, the opposite is true.
9. Data input gamification
As we have discussed under solution #8, you have to make sure that the digitally savvy, young ‘instant generation’ takes the effort and patience to share a lot of information with you.
One way to motivate this is to gamify the data input process.
To incentivise additional data sharing from the client.
To offer goals and to provide metrics and benchmarks about how the client is doing in terms of giving you as much data and information as possible.
Think about the typical social media gig of ‘your profile is 59 percent complete’, please now share this and this with us to make your profile a ‘pro’ from a ‘light’ one.
People with a ‘pro’ profile receive a badge, etc.
Obviously the data input gamification has to be fully compliant with banking regulations and follow the strongest data privacy laws.
Think about fun ways to ask for data and give something (for example a gamified experience) in return.
10. AI output design-gamification
‘CX is the new product in banking’ the bon mot goes.
What does this mean?
CX (Customer Experience) in a digital environment is defined by the quality of UI (User Interface).
The quality of a digital Used Interface is defined by ‘design’.
This is why Steve Jobs orchestrated (20 years before others realized the same) that ‘design is not just what it looks like, the design is how it works’.
Millennials and Gen-Z have a preferred overall logic and style of design: it originates from gaming.
The colours, the gauges, the screen dashboards, the data visualisation… it is all ideally using the visual language of computer as well as smartphone games.
A good example of this is Moven starting from New York, serving young people by providing superior Personal Finance Management (PFM) dashboard through smartphones and even smartwatch (Moven accounts have recently been taken on by Varo).
You can have the best deep NN (Neural Network) design working in the background, but the clients will judge the output primarily based on CX, defined (as explained above) by design.
11. Constant digital client-satisfaction review for successful AI in banking results
Everything your client does in any of your available channels has to end by feedback.
One of the global best practice examples is the feedback system of Booking.com.
They are collecting first an overall, simplified ‘5 different emoticon’ based set of feedbacks and as a second additional layer of engagement, the client can turn the interaction into a full-fledged written review.
Negative feedback is even more valuable than positive: it signals, you need to improve something and it also makes the positive interactions more credible.
Negative feedback also ‘everything unchanged’ strengthens loyalty, because it provides an easy way for the dissatisfied clients to vent.
Therefore: have the five emoticons come up at the end of every micro-process your clients are performing digitally!
12. Turn data into habit-forming products
Habit forming products are microservices which make you open them on your phone multiple times per day.
Think about checking your email, looking for text messages, peeking at your account balance, looking at the number of your YouTube subscribers, checking Facebook notifications, looking at WhatsApp if you have new messages, popping in and looking at your LinkedIn news wall, looking at your calendar, Tweeting, looking at a news site (or a news aggregator site), searching for things on Amazon, checking flights and ticket prices, looking for an Uber ride or how much your recent ride cost, looking for real estate or cars, etc.
Habits differ individually and across generations, for example, Gen-Z preferences are Instagram and TikTok over Facebook¸ looking at your stocks, online brokerage, Robo-advisor, or eToro (copy trading app), etc.
Anyway, the quest is to tap into these habits by creating habitual micro products.
The best tool for this is data and presumably AI: to anticipate and proactively support the next move of your client.
13. Complex inputs translated into simple outputs
‘The AI Excellence Paradox’ is described by the paradox juxtaposition between the ideal complexity of inputs being translated to simple outputs.
For retail banking as well as Small and Midsize Enterprise clients the simpler the outputs are, the better, and more acceptable the product is, and banks should consider this when implementing AI in banking processes.
Steve Jobs had a quick test of sufficient simplicity: ‘First time I see it, I can use it; the second time I see it, I can teach it’.
Simplicity (from the user’s point of view) is a general characteristic of disruptive digital products, with exponential uptake.
This is a paradox surrounding B2C AI: to quest for complex and extensive sets of inputs but to provide simple outputs.
14. Turn the expression ‘AI’ into a client-oriented concept
What exactly powers a banking product in the background?
Where exactly is the fine line between smart software and AI?
These questions are typically irrelevant for 99.99 percent of the core retail clientele.
Therefore expressing explicitly that a product is powered by AI is irrelevant, or may sometimes even be a factor or alienation.
Find creative and meaningful ways to express what the client in fact gets as added value.
Choose one expression from the left column of the chart and combine it with one from the right. For example Additional Insights, Accessible Intelligence, or Awareness of Intents.
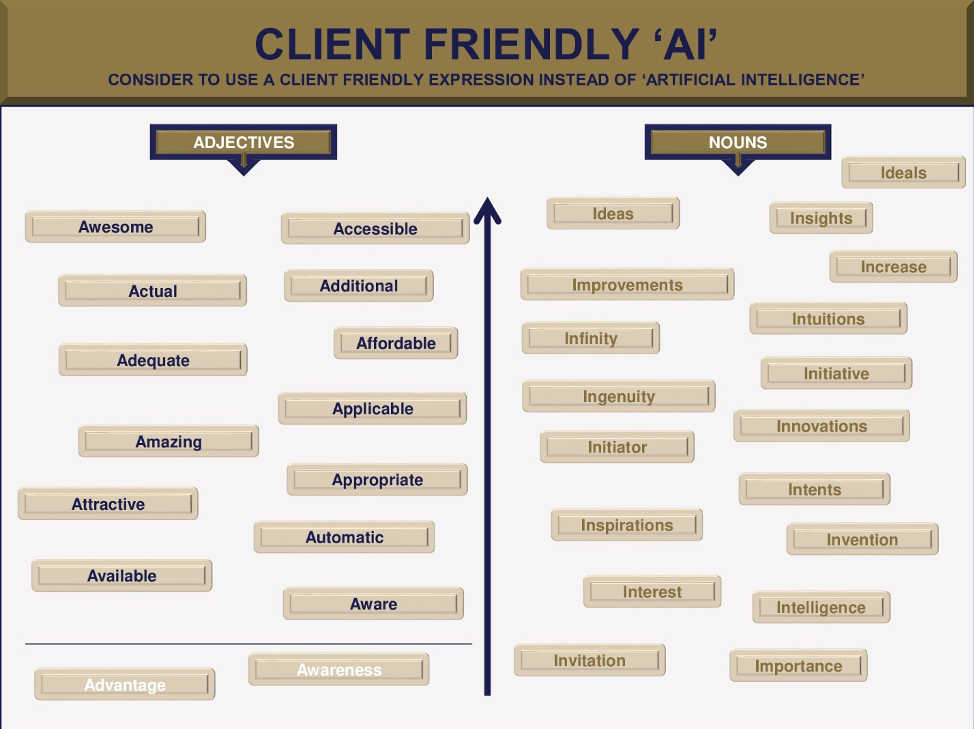
In case you also want to explicitly mention Artificial Intelligence, you can say something like Attractive Improvements powered by AI.
15. Explicitly commit to ‘ethical AI’
Make sure you proactively and explicitly communicate to stakeholders your absolute commitment to ‘ethical AI’.
AI in banking will be increasingly surrounded by skepticism among global opinion leaders in terms of ethical issues as well as legal, liability, and risk-related questions.
It is best to express your commitment to and familiarity with ‘ethical AI’ proactively in your Annual Report, in your CSR or Sustainability Report as well as in AI-related PR and marketing activity.
16. Client facing AI has to have absolute priority
While there are great opportunities in AI applications in your back office, treasury and other internal functional areas the guiding star has to be instant consumer value.
Yes, CTI (the cost to income ratio) is extremely important, and back-office AI can cut costs.
Still, by far the greatest battleground will be CX (Customer Experience) between traditional, large, incumbent, bricks and mortar banks versus Neo Banks, FinTech Startups, Technology Giants, and other data-savvy players.
Therefore any and every client-facing AI application has to have priority.
Large banks with legacy IT are typically equally unprepared for AI in their back offices and in their client-facing interfaces.
When they finally take the effort to cut through this, it first has to go into added client value.
Full back-office transformation is almost impossible, it is like changing engines mid-flight.
Back office AI is valid, but very often it ends up as a ‘one-off pilot’ and terminates.
And the bank keeps on investing in these scattered pilots.
It is much better to produce something which is client-facing and hands you competitive advantages in Customer Experience.
Core systems are key and they need to be fundamentally updated, but banks now need to produce shiny and meaningful immediate front end, client-facing achievements.
17. Have an internal as well as external ‘AI narrative’
AI in banking is an expensive and tough issue for large banks.
You have to, therefore, explain to internal as well as external stakeholders why you are doing this.
You have to tell people what your goal with using AI in banking is, why AI in banking can be used as a great tool to reach this goal, and how this fits into the broader narrative of your bank.
You have to derive your current innovative excellence, preference, and appetite from your past.
You have to show to internal stakeholders why it is beneficial for them that your bank takes AI seriously and how you are consciously mitigating the downsides.
For example, it is typical to hear bankers express concern that AI in banking takes their jobs away and the bank, in fact, wants to get rid of them and ‘the AI and RPA project’ is just a codename for making them redundant – of course, they will resist your AI attempts as much as they can.
You have to explain the same things to shareholders and clients as well.
Make sure that the narratives for different shareholders are in synchrony.
18. Prefer in-house AI in banking projects over vendors
Pulling off meaningful in-house AI in banking projects is hard and for a traditional bank, it is extremely hard.
In fact, it is so hard that besides the primary difficulty (data infrastructures and data systems not being ready for AI) this is the second most painful hardship.
There is a lack of available AI in banking talent and cutting edge AI is a fast-moving target in every aspect.
Furthermore, AI efforts need a relatively sizeable team to focus on and when a bank tries to go big on AI it usually bumps into the number one difficulty: the legacy core systems and fragmented, outdated data infrastructure.
Yet, the premium on a successful in-house AI team is huge. It lowers the reliance of mixed quality vendors, it reduces the vulnerability to the ‘black box’ nature of AI and it creates real competitive advantages.
It can also make the 2nd, 3rd, 4th, 5th…. 20th AI projects increasingly fast, conscious, high quality, and cost-efficient.
And once you have a functioning and successful AI team producing customer value, again and again, you are in a much much better position to negotiate with vendors as well.
Alternatively, you can even take your internal capabilities further and spin them off, monetize this unit and keep some ownership in it, while selling some of the innovations to competitors and use the revenue and profit for further innovations.
This is what we call the ‘inside-out’ methodology of innovation.
19. Learn to separate ‘AI hype’ from ‘AI reality’
When evaluating a project proposal for your own organisation you need to be confident and able to separate the hype around AI from the real potential of it.
This is tough and needs learning way beyond the boundaries of this article.
Yet, in an oversimplified way, the exercise is about being able to separate, categorise, and recognise systems that Act, Predict, Learn, Create, Relate, Master, and Evolve.
Most experts put the barrier between traditional software and AI between ‘predict’ and ‘learn’.
20. Have explicit, written, documented, official, and enforceable executive commitment led by the c-suite for your ‘AI in banking strategy’
Your ‘AI Strategy’ has to be derived from your ‘Innovation Strategy’.
Your ‘Innovation Strategy’ has to be derived from your overall ‘Business Strategy’.
C-suite is responsible for the overall ‘Business Strategy’.
Therefore a strong, stable, conscious, and well managed ‘AI Strategy’ needs the approval of the C-suite.
Otherwise, it will be organisationally weak and get lost in old style, occasionally overly risk aversive banking culture and set of preferences.
Having a smart ‘AI Strategy’ is necessary, but not sufficient.
To have real achievements, you need explicit, written, documented, enforceable, permanent, solid, and resourceful C-suite commitment.
How do we get AI in banking right?
Getting AI in banking right, especially as a traditional bank is an uphill battle.
Yet, winnable.
This makes any client-facing value achieved by AI a strong, powerful, and meaningful competitive advantage compared to your peers and a cutting edge best practice compared to tech companies, challengers banks, and FinTechs.
In the short run, you will have to work around your current data infrastructure to get the right quality and structure of data to feed AI.
Yet, client-facing AI success is so sweet and powerful that this may be the necessary incentive for the bank to gain the courage to ‘change the engines mid-flight’ and migrate to an entirely new and cutting edge overall data infrastructure.
So AI is the best excuse to finally ‘get data right’.
One Comment