8 minutes read
Scalability is emerging as a major competitive advantage for businesses who are investing in an AI-driven future – and arguably, there has never been a better time for businesses to scale their operations.
With AI’s predictive and analytical capabilities, businesses are able to identify and access new markets more easily than ever before. Furthermore, Generative AI tools and automation are enabling businesses to increase their customer base while still providing quality services and products that are tailored to their customers and local markets. This means that they no longer have to compromise on quality as they expand.
According to research from software company, Zartis, the use of scalable software brings significant benefits, including:
- 2.3x higher revenue
- 61% increase in innovation
- 23% improvement in performance
Nevertheless, scaling with AI is no walk in the park – and this is not just because of the complexity and limitations of the technology itself. To gain real value from automation and other AI tools, businesses need to have productive and adaptable systems in place. This means more than just investing in scalable software, and requires companies to address the underlying social and structural issues which are hindering their innovation and productivity more generally.
A human-centric perspective on AI
Zartis approaches AI and the issue of scalability from a human-centric angle. With a background in providing SaaS for HR departments, the company brings seasoned experience to the process of digital transformation, particularly when it comes to handling the unique challenges that typically arise when human workflows meet their technological counterparts.
At Zartis’ Growth Summit last month in Madrid (23rd May 2024), the company brought together industry experts and entrepreneurs to discuss the current technological landscape, and share best practices for scaling organisations in the AI era. Throughout the day, keynote speeches and panel discussions illustrated the specific challenges and benefits that different businesses had encountered in their unique experiences of scaling operations with AI.
Interestingly, many of these challenges turned out to be social and/or structural problems, rather than technological ones. This indicates the prevailing importance of ‘the human factor’ in achieving sustainable company growth, and in reaping the rewards of Generative AI and automation in the long run.
Nevertheless, the role of humans in laying the groundwork for AI is easy to lose sight of in a world which has been awestruck by the unprecedented abilities of today’s Generative AI technology, and is prone to viewing AI as a threat to human uniqueness.
Zartis take a refreshing take on this matter. In their view, while the creative processing abilities of Generative AI might be unprecedented, its impact on society is not. They see Generative AI & Deep Learning as simply the latest techno-paradigm shift in the wave of technological innovations that have been shaping the modern world since the first industrial revolution over 200 years ago.
In a keynote speech at the Growth Summit, Niamh Bohan, Transformation Leader at Zartis, highlighted that human resistance and social disruption were common symptoms of these shifts, given their profound impact on many industries, and society as a whole.
“These [big technological revolutions] caused massive shifts in the economy, society, ideologies, laws, and institutions. They caused entire industries to have to unlearn and learn again. They caused massive tensions between the old business models and the new business models. And they caused tensions between the people who have the new skills and the people who didn’t… AI is a technical innovation, but it’s not confined to technology. It’s going to affect all sectors and all business units.”
Niamh Bohan, Transformation Leader at Zartis
Considering Generative AI from this broader and sociological perspective can help us to see the transformative impact of this breakthrough technology, and realise what we can achieve with it.
Additionally, by understanding the holistic impact of previous technological innovations, businesses can better prepare for the key challenges that they can expect to encounter as they leverage AI for efficiency gains, financial assets, and strategic insights.
Ultimately, while current emphasis is on early AI adoption as the defining factor for success, the real winners are likely to be the ones that prioritize the human factor as they integrate AI into their business operations. This means investing in workplace culture, creating a resilient organizational structure, valuing employee skills, and fostering strong communication channels between teams. Such investments will help businesses to overcome some of the key barriers to productivity in their workflows, and avoid the common pitfalls that companies can fall into as they integrate AI into their operations.
Below, we look at workflow bottlenecks, which are one of the most stubborn structural barriers to successful scaling with AI, along with tried-and-tested advice from industry experts on how to overcome them.
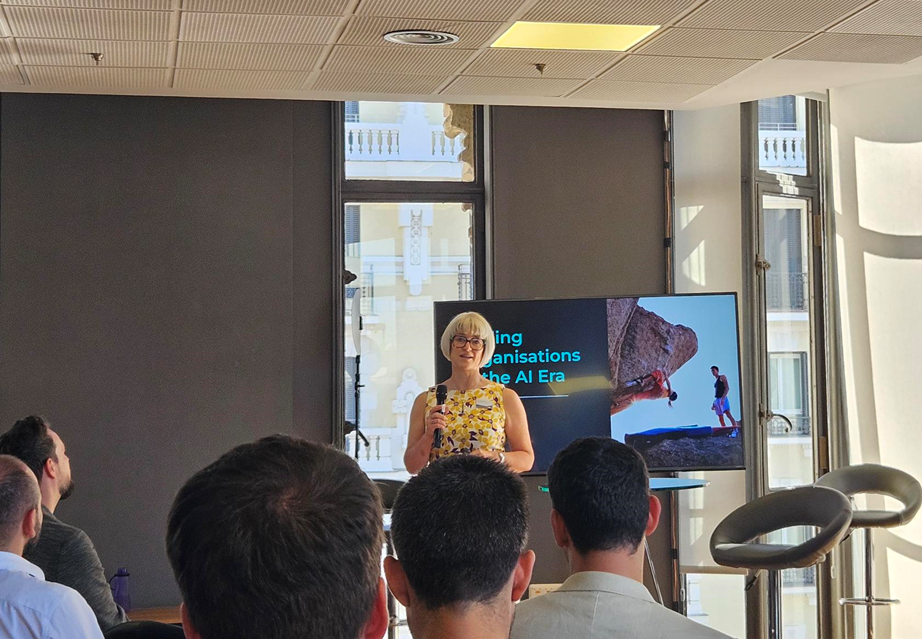
Workflow bottlenecks
Workflow bottlenecks, where nearly all the work accomplished by a team has to pass through one individual, can be a big hindrance to both general productivity, and innovation with AI. They are most likely to occur in teams where projects require a high level of expertise and where there are team members of significantly different abilities.
In particular, they can cause problems in software engineering teams, where ‘hero culture’ is relatively common due to the technical expertise of this domain. Drawing on her own personal experience and background as a software engineer, Niamh Bohan explains how the productivity of such teams can be limited by the bottleneck of coding expertise.
“One of the teams I worked with doubled the size of their engineering teams from 8 to 16. They had lots of data flowing but they realised they weren’t getting a substantial increase in productivity from the team. When they looked at why, they realised it was due a Senior Director of Engineering who had started out on the team, was one of their heroes, one of the people involved with all production, every incident, and he was the only one to do code reviews. He was involving himself in every project for the best reasons – because he really understood the code. But that just shows you how something simple like human interaction can cripple a team and their productivity.”
Niamh Bohan, Transformation Leader at Zartis
Workflow bottlenecks tend have a particularly negative impact when businesses move to scale their operations, which might increase or change the workload of a team. If a major part of a team’s production rests on one individual, the company will struggle to increase its operations much beyond what this one individual is able to oversee.
They also present significant challenges with bringing in new talent or changing the composition of a team. Not only do workflow bottlenecks impact the social dynamics of a team which can make it difficult for new individuals to integrate themselves, but they can also limit the company’s flexibility to mix and match team members with different skillsets to create new teams to oversee pilot projects.
Structural solutions to workflow bottlenecks
Addressing the problem of workflow bottlenecks can look quite different according to the unique structure of each business model. Nevertheless, streamlining the structural composition of teams appeared to be a common factor of tried-and-tested approaches discussed at the Growth Summit.
Global B2B car rental company, CarTrawler, found that an effective way to prevent the formation of workflow bottlenecks was to ensure that each team is a self-sufficient unit. Jim Carr, VP of Product at the company, explained the benefits of this model in a panel discussion at the Summit.
“So you build scalable applications… by prioritizing atomicity, i.e. doing one thing well and not having a shared state between your application modules. We’ve done the same thing with our teams. Every team is a full stack team, every team can build something. Your content middleware, the back-end data – all that you can just go and solve in one place. This way, you have minimal dependencies that spider out from each other. These dependencies slow you down because as you scale the organisation and the number of teams, those dependencies grow logarithmically and everything slows down.”
Jim Carr, VP of Product at CarTrawler
This model, which treats teams as independent business models in themselves, promotes greater autonomy and productivity by giving teams a more defined workflow structure. By allocating specific tasks that align with the particular strengths of different members of the team, this model helps to distribute the workload rather than the majority falling on just one individual.
Compliance & Risks, a software company based in Ireland, adopt a similar practice, implementing vertical topological frameworks to structure their engineering teams. This means building teams from people with varying levels of ability and different areas of expertise, meaning that each team represents a cross-section of the business’ workforce. Key advantages of this model include: interdisciplinary collaboration, more structured workflows, and increased employee investment in projects.
Shoibhan Fairman, Head of Engineering at Compliance & Risks, explained in the Summit’s panel discussion that this framework was helping their teams to avoid workflow bottlenecks by involving the engineers in a greater variety of collaborative tasks. This enabled them to learn through experience, develop their expertise through teamwork, and increase their holistic understanding of the product development process.
She pointed out that this model had the added benefit of enhancing the job satisfaction for the engineers – it meant that they could see, for example, how the product meets the customer’s needs, rather than focusing just on the technical aspect of the project.
A crucial factor for success that both these models rely on, however, is having external teams who are responsible for overseeing the process of product development, and providing training programs. This is a key way to level the playing field within teams, and mitigate the impact of any existing workflow bottlenecks.
The impact of AI on workflow bottlenecks
AI’s relationship with workflow bottlenecks is a double-edged sword. On the one hand, by automating certain operations within a team’s workflow, AI can help to address workflow bottlenecks by reducing a team’s dependence on a particular individual for certain tasks. For example, for a team who are tasked with drafting contracts which have to adhere to particular standards, AI could act as a compliance manager, checking that the contract complies with the company guidelines.
On the other hand, AI can make workflow bottlenecks worse. In practice, integrating AI into workflows typically involves automating the easier, lower-level tasks which any of the team members can do. This means that, while the workload for the team as a whole might be reduced, most projects will still have to go through the bottleneck because AI outputs typically require oversight and troubleshooting from an expert. Thus, AI itself is not really a solution to the problem – if anything, it may simply accentuate existing bottlenecks and structural issues within a team.
Therefore, businesses need to optimize their workflows before they automate and throw AI tools into the mix. While automation is a key facilitator of scalability, it is only beneficial if the process being automated is in itself valuable. Without evaluating existing workflows and team dynamics, companies risk simply scaling their problems and inefficiencies. As Bohan pointed out, any value from automation ultimately comes down to how it impacts the employees.
“We need to look at how we can help people be more productive using AI. That’s not just about tools, it’s also about understanding how they work and giving employees the knowledge to identify where their work can benefit from AI.”
Niamh Bohan, Transformation Leader at Zartis
Conclusion
Ultimately, the structural issue of workflow bottlenecks requires structural solutions rather than technological ones. Businesses need to consider carefully where the bottleneck is, and why it exists. Is it purely the technical expertise of an individual that is creating the bottleneck, or are there other social factors such as who has responsibility for the success or failure of a project, for example.
Without examining how the bottleneck has developed within a team, and first dealing with the social and structural dynamics that the bottleneck has created, the value that AI can bring to a team will be limited, and businesses will struggle to scale their operations much beyond what a few individuals are capable of.