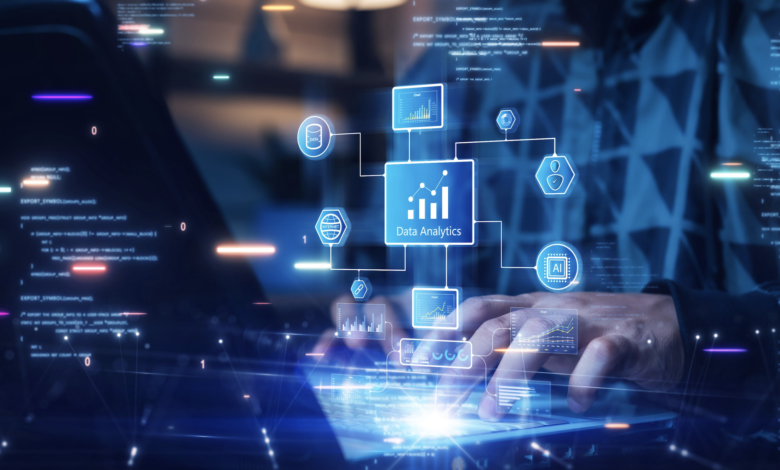
For every executive, AI has emerged as a central focus area, and for good reason. Advances in technology, availability, and applicability have been such that companies not investing in AI risk underperformance and ultimately obsolescence. But a key question emerges: if implementing AI is mandatory, which path will they choose? Buyers know there is a build vs. buy trade-off in technology. However, selecting and starting a long-term journey with a vendor is not a decision to take lightly when it comes to AI with its complex algorithms and fast-changing technological landscape.
Conversely, building AI with now widely available science talent dangles the promise of a perfect solution tailored to your requirements for maximum performance. However, it is too often crippled by the reality of rocky deployments, maintenance nightmares, and inevitable decline and failure. What if a third option could bring the best of both worlds? “Bring Your Own Science” is a unique approach embraced by companies who recognize the importance of enterprises developing and owning their company-specific AI, while running on modern productized platforms.
Buying productized AI is not like buying other software
Productized enterprise AI differs from traditional IT applications in many ways. The most obvious difference is that enterprise AI applications rely on central models and algorithms. These models learn business patterns from your data to create business-specific outputs, such as insights, recommendations, automation, etc. While varying degrees of complexity exist, nearly all AI models share one common pattern: complexity. Models are the result of months of research and engineering, leading to data transformations and calculations that will always require effort – sometimes considerable effort – to understand.
Going the productized route for AI therefore has implications. First, productized AI is built for many customers, rather than accounting for the fine specifics of every business. Standardization has evident benefits such as the ability to incorporate best practices, standards, and configurability, which help with transformation and change management. But with a one-size-fits-all approach, the match to your business will never be perfect, even with configuration and tuning, since every model design relies on foundational assumptions and hypotheses that may only partially apply to your business. Indeed, every AI model by definition represents the real world with assumptions such as some phenomena will be negligible, responses to changes will follow assumed mathematical patterns, etc.
For example, a forecasting problem such as predictive maintenance might find that algorithms designed by the vendor may work well to predict failure rates of your most common assets (trucks, machinery, etc), but your company’s most important and demanding customers happen to also be served by different specific assets (air logistics, specialized welders) which collect a lot more data and behave differently. The productized solution will be suboptimal for this unique yet important business area.
Furthermore, since AI models are complex, it will rarely be possible to truly understand how third-party models are designed, or even how detailed calculations are performed, requiring a high degree of trust and dependency on your vendor. This trust can quickly erode when users start to second-guess model outputs, lack the transparency and control to understand how the model behaves or correct it, and only see reassurance from the vendor’s experts rather than from their own eyes.
Company-specific AI is elusive
If those limitations are concerning, it is natural to be tempted to develop your own company-specific AI. After all, AI constitutes some of the most strategic and differentiating investments being made, and with data science talent now widely available, it is reasonable to want to own and control your AI destiny. This includes having a say in model design decisions and relying on internal experts to explain how model decisions are made. Additional transparency and accountability are greater guarantees of adoption and success.
Going from theory to practice in company-specific AI is where things break down. Most data science initiatives fail and home-grown AI projects are even more prone to failure than regular IT projects because creating a custom model opens a pandora’s box of adjacent critical requirements: the infrastructure and ecosystem surrounding the model, adoption and change management, etc. Those areas are precisely where productized solutions offer thought-through alternatives. Add to this equation the organizational complexity and higher total cost of ownership of custom AI, and company-specific AI becomes a goal that very few companies can afford and execute.
A third option: Bring Your Own Science
If custom company-specific AI is unreachable, companies that want to go beyond productized AI to perfect their algorithms remain stuck with imperfect solutions. Except if the vendor opens up its system to third-party algorithms developed or co-developed by customers or consultants, thereby allowing them to bring their own science.
Bring Your Own Science (BYOS) provides a unique opportunity for large enterprises to own their AI when it makes sense to do so. Noticed an algorithm that is underperforming in your solution? Replace it with your own. Have more data to bring to improve accuracy? Extend your solution with a proprietary algorithm leveraging new data. Whether it is to achieve greater performance, specialize algorithms for your business, or ensure you understand designs and decisions made by AI, BYOS can provide tremendous incremental value.
While BYOS requires an open vision from technology providers, it also relies on concrete software capabilities. A mere data exchange with third-party AI/ML platforms or public clouds would for example not be regarded as BYOS, since it would lead to poorly integrated solutions. Instead, mature providers should offer the ability to run customer-specific AI in the same way the productized AI runs, including transparent integration in business workflows, data availability, model lifecycle management, and incorporation of the same business best practices. This requires a conscious investment into platform openness, for example via the release of APIs and SDKs on a modular architecture allowing it to “compose” algorithms from multiple parties.
BYOS for futureproof AI journeys
Initially, companies buying a BYOS-ready platform benefit from a strong foundation allowing them to deploy AI quickly with a productized solution incorporating models designed by experts. This ensures a fast positive ROI on the initiative, reduces deployment risks, and generally forces enterprises to follow business best practices. This prescriptive approach minimizes short-term adoption risks at times when companies are the least mature.
The flexibility of BYOS can then kick in months or years after the initial deployment depending on each company’s strategy, maturity level, and opportunity. Similarly, the second part of the journey remains high-value and risk-conscious, since companies enhance an already-strong foundation iteratively to focus on differentiators and other specialized parts of their AI application. BYOS guarantees the solution is futureproof; it can constantly be improved when opportunities are identified and prioritized.
AI is evolving at lightning speeds and chances are your business is too. Picking a vendor that can keep you at the forefront of AI over the years as your company’s AI maturity grows is difficult. Therefore, BYOS is of strategic importance for companies that want to set themselves on the right long-term path.