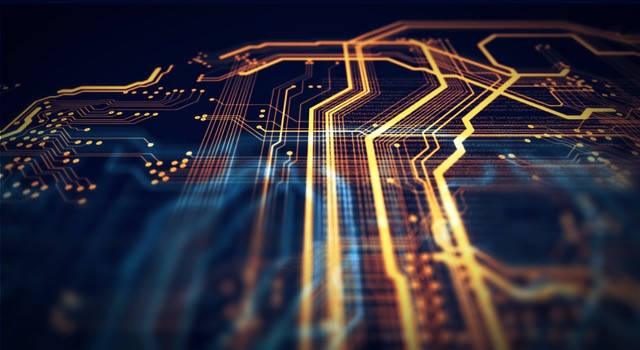
Chief Information Officers (CIOs) are now well-acquainted with the hype surrounding Generative Artificial Intelligence (AI) and its promise of time and cost savings. However, the practical hurdles in implementing AI-driven business applications, such as inaccuracies in models like ChatGPT and sluggish public versions of Generative AI, are not always acknowledged.
Despite this, if we can get AI to assist with business-critical database applications, then enterprise IT teams would be keen to know more. Envision a scenario where AI seamlessly collaborates with vital, business-critical database applications – this prospect would undeniably capture any savvy CIO’s attention.
The primary challenge lies in efficiently searching your database based on user prompts while maintaining data ownership and low latency. Until now, success has proven elusive, even with scalable, open-source databases like PostgreSQL. PostgreSQL users were pioneers in developing AI-powered applications long before the ChatGPT frenzy began last year and continue to lead the way with many born-in-the-cloud, AI-powered applications.
For instance, our company actively collaborates with a leading AI-driven procurement analytics platform, which utilises the cloud-native transactional database YugabyteDB. The database plays a pivotal role in optimising supply chain partner selection and pricing. It also delivers anti-money laundering (AML) initiatives by employing machine learning algorithms on a blend of public and anonymised private customer data.
Using a database to fuel large-scale, industrial AI systems is possible. However, a major impediment to AI progress in the database domain has been the absence of support for complex searches beyond traditional SQL schemas.
It’s important to note here that AI professionals prefer working with mathematical representations known as ‘vectors,’ which are capable of encompassing numerous features or attributes. By their multi-dimensional nature, Vectors enable efficient storage of detailed information as a single record, facilitating easier searches for similarities.
Vector processing, encompassing the storage and retrieval of vectors, is crucial for AI applications that leverage databases. The ability to swiftly find similar or relevant data based on semantic or contextual meaning is a hallmark of vector databases.
Specialised vector databases already exist, so use them if they make sense for your IT project. But, if you want the advantages of a scalable database that works as well on-prem as it does in the cloud (something PostgreSQL is all about), it’s better to be able to use vectors in your current database engine. This also allows you to connect to new or existing AI-friendly apps and services seamlessly.
A compelling route is to integrate your AI development with a scalable, SQL-based object-relational database like PostgreSQL.
Previously impractical, this route became a reality in 2023 with the announcement that PostgreSQL supports vectors following the introduction of the pgvector extension. Developers can effortlessly enable the extension by creating a table with vectors as a data type. This provides a streamlined way to propel AI applications through extensive datasets using the robust ‘search for similar things’ capability.
In technical terms, pgvector offers essential vector norms such as exact and approximate nearest neighbour search, L2 distance, inner product, and cosine distance. Its versatility extends to applications using programming languages that target a PostgreSQL client, from C to Ruby, and Java to Python.
This development is a boon for the enterprise database community as it offers a fresh way to integrate AI development using a familiar, scalable, SQL-based object-relational database like PostgreSQL (or PostgreSQL-compatible options like YugabyteDB).
The incorporation of pgvector is particularly beneficial for sectors like financial services, which demand ultra-low latency and real-time, fault-tolerant data handling in their pursuit of AI. It also opens the door for less traditional use cases, such as data warehouses, which are eager to delve into vectors and the advanced search capabilities they unlock.
So, don’t delay! Now is the ideal moment to discover how AI can benefit your business and capitalise on this newest wave of innovation.