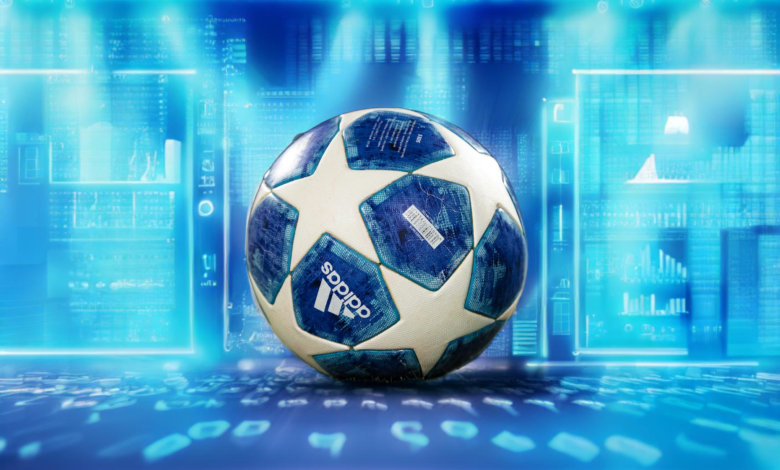
Employing AI without having a strategy in place is like telling Lionel Messi to play football without introducing him to his teammates or briefing him on the competition. Like Messi on a football pitch, AI is multi-talented; its skill set can be applied in many areas of business, but it can’t solve all organisational challenges in isolation or without smart planning.
To even ask it to do so would be a financial and branding disaster. Conversely, when deployed in line with a clear-eyed business strategy the potential is exhilarating, particularly if you focus on an area such as predictive modelling.
AI predictive modelling helps retailers with demand forecasting, financial organisations with fraud detection, manufacturing businesses with predictive maintenance and healthcare with epidemic modelling; the possibilities are enormous.
For most businesses, accurately predicting customer churn is essential. Managed poorly, teams will struggle when forecasting profit and loss or inventory levels and can end up suffering serious cash flow difficulties. AI predictive models analyse customer data, buying habits, and market trends to provide accurate insights. Businesses can then tailor their sales campaigns and anticipate demand fluctuations, allowing better management of resourcing and procurement, thereby giving them a competitive edge.
It’s all about quality
Although AI and predictive modelling can transform a business, they can’t manage it alone. Even Lionel Messi isn’t winning trophies by himself! To ignite AI’s predictive magic, another ingredient is required: quality data. But why?
Imagine a coach briefing a football team in gibberish. No matter how intelligent the players, their ability to learn and perform well would be hampered. The trophy would be lost. Now think of AI as a powerful learning machine. It can analyse vast amounts of information, identify patterns, and even learn from experience. But what if the data being injected into it is nonsensical? Predictive modelling uncovers hidden insights humans might miss in raw data.
However, if the data is full of errors, inconsistencies, or missing information, these supposed insights can be misleading or even non-existent. To avoid this, there are three key considerations organisations need to keep in mind to ensure quality data. I call these the 3Cs:
- Coverage
- Contextualisation
- Correlation
Let’s look at the 3Cs in more detail.
Coverage
Coverage, when it comes to data quality, boils down to: what data do I have, and can I see it all? Just as a player like Messi needs to be able to see what’s happening in the game around him – who he’s playing with, who he’s playing against – organisations need to have full visibility of their technology environments and the data held within.
Once you have worked out where your data is and how to access it, you need to move on to the next stage – understanding and contextualising it.
Contextualisation
Contextualisation is the ability to understand, not just where your data is coming from – is it generated internally or collected from external sources? – but to work out what it means.
Another football analogy may help explain this: a player may be able to see the opponents in front of them, but if they don’t understand the tactics in play, they will make the wrong decisions on the ball, or at least put in a sub-par performance. Contextualisation is vital for informing the AI programme that delivers your predictive models.
Correlation
Correlation is about getting rid of silos and integrating information from different contexts.
Once you have the coverage and the context of your data, you need to bring it all together and make it correlate. This step is about ensuring that data from different systems is working in harmony, working towards a united goal. You don’t want to be giving your AI programme disconnected, confusing information.
This overview of the 3Cs should offer you the insight you need to begin the planning process. Applying the 3Cs is vital for providing AI with the clean, quality data it needs to build the predictive models you hope to benefit from. Only once that data quality is elite, can predictive modelling calculate what will happen next with a degree of certainty.
Practice makes perfect
The process should not stop after you gather quality data and feed it into your AI programme. Just like in football, to succeed in the league or be promoted to the Champion’s League you need to keep playing. The more you play, the more information there is to analyse, the greater (high-quality) data you can secure. The greater the data, the better your predictive modelling gets. And the better the modelling gets, the better informed your business decisions will be, creating the ideal mix of informed strategy and in-game adaptability.
Is it worth the effort? Yes. AI predictive modelling holds immense potential to revolutionise how organisations approach their future. It’s the ability to see beyond the present and into the possibilities of what lies ahead. By leveraging its power, managers can make data-driven decisions, mitigate risks, and unlock a world of possibilities, no matter their industry. As AI continues to evolve, AI predictive modelling will empower us to shape the future, not just react to it.