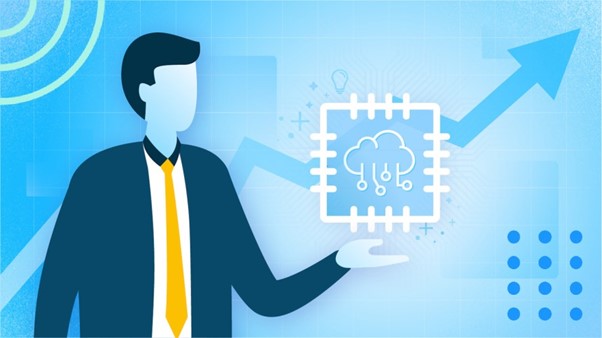
AI has potential to bring great gains to businesses in terms of scalability, efficiency, and innovation. But many businesses are still struggling to turn this potential into tangible, real-world value.
According to recent research from Deloitte on the global state of GenAI adoption, only 18-36% of organisations are currently seeing results that meet their expectations for AI to a ‘large’ or ‘very large’ extent, depending on the type of benefit being pursued.
In this article, we look at what differentiates this successful minority of ‘AI leaders’ from the majority of organisations, and consider some of the ways that companies can see the greatest returns on their AI investments.
Innovation as a key strategic differentiator
Deliotte’s 2024 State of AI Adoption report identifies the use of GenAI for innovation as a key strategic differentiator that sets AI leaders apart from other organisations. The report found that while most organisations view GenAI as primarily a tool for productivity and efficiency, organisations with higher levels of GenAI expertise are focusing more on innovation and developing new products and services.
This finding does not come as a huge surprise. After all, isn’t it always the case that the bigger, leading companies are the ones who tend to be able to hire more talent and invest more in R&D? Arguably, this is the very foothold that enables big companies such as Microsoft, Google, and Amazon to retain their dominant positions in the global market.
According to FDi Intelligence, Amazon spent $73.21 billion on R&D in 2022 (equivalent to Musk’s approximate net worth), with other dominant tech companies including Microsoft, Intel, Apple, and Meta also being among the biggest spenders on innovation. This is also increasing year on year, with the R&D proportion of Amazon’s overall budget rising steadily from 11.9% in 2021, to 14.2% in 2022, to 18.5% in 2023, according to Finbox.
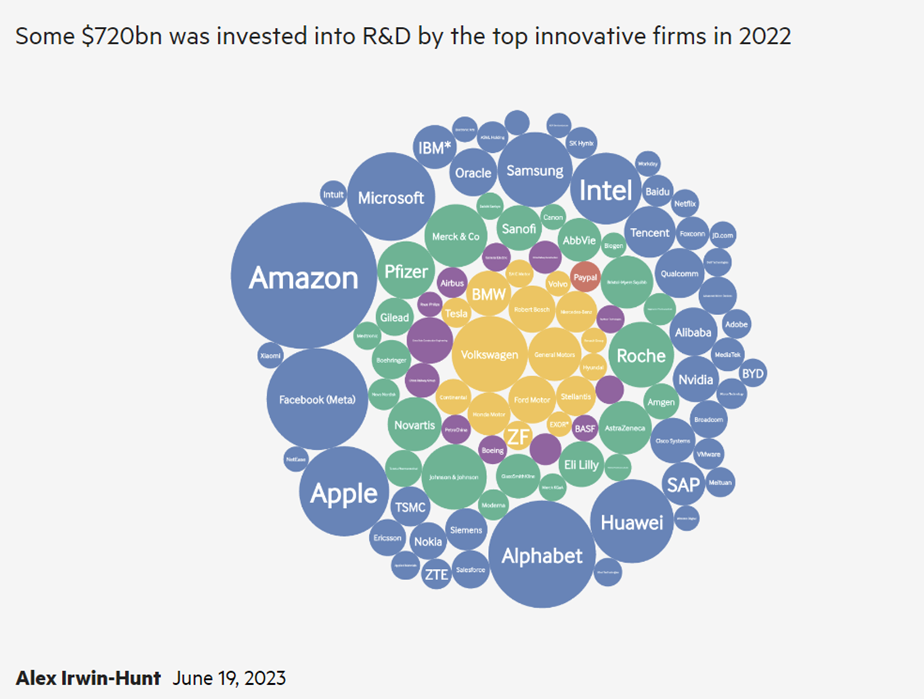
The visual representation of R&D investment above also demonstrates that the tech industry (blue) is by far the biggest spender on innovation, followed by healthcare (green), automotive (green), and other industries including energy, financial services, and industrial services (purple). This indicates the crucial role of innovation for gaining competitive advantage with emerging technologies such as AI – and the fact that the many of the highly successful tech companies are among those spending the most on R&D speaks for itself.
Now, as innovation becomes an increasingly recognised as the key strategic differentiator for ‘AI leaders’, we are also seeing increasing pressure on product development teams to innovate at a faster pace.
For example, software development company, OpenText, spoke at their World Europe Event in London, April 2024, about the faster development cycles they were implementing for their engineering teams. So far, they have increased the rate of development exponentially, moving from 18-24 month cycles to annual cycles, then to 90-day release cycles (their current rate), with the eventual goal of releasing new products and updates every month. This has been achieved within the context of the company increasing the R&D portion of its revenue from 12% in 2020 to 14-16% in 2024.
Nevertheless, as OpenText EVP and CPO, Muhi Majzoub, explained, this faster pace of innovation is made possible thanks to not only a bigger budget, but is also enabled by a change in mindset of the engineering teams as they embrace the efficiency gains of automation.
“Our [product release] quarters are January, April, July, October. This way, my engineers now have the discipline to be on a 90-day release cycle. The mindset of engineers and QA (quality assurance) managers are no longer thinking, ‘oh, I have nine months to test’. No, we don’t – we have two weeks to test. That means we need to improve our automation, we need to improve how we use Value Edge (OpenText’s DevOps platform). Because our customers don’t have the luxury to release every year or every 90 days. If you’re a McDonald’s or a BMW, you are pushing code through your test cycle every single night. The Head of Quality at BMW said that every single night they submit 2000-3000 test cases to the automation server. The goal for us is for my engineers to be submitting 1000s of lines of code the Value Edge Aviator, and Aviator will test it, document it, and regenerate it if needed. One day, the Aviator itself will fix the bug, and then give a log file report to a human being to look at and for the development manager to sift through.”
Muhi Majzoub, Executive Vice President and Chief Product Officer at OpenText
This indicates that automation, while not always the most exciting application of AI, is in fact a crucial catalyst for innovation. With many companies already harnessing the power of automation for efficiency gains in their existing operations, it is finding ways to automate the process of innovation itself that may be the crucial differentiator that gives companies a key strategic advantage, one that may well end up distinguishing the ‘AI leaders’ from their competitors.
Other attributes of ‘AI leaders’
Alongside leveraging AI to automate the process of innovation, there are several other attributes that AI leaders appear to have in common, which also serve to complement their ability to innovate.
The presence of an AI governance policy/committee dedicated to overseeing the use of AI within the company
According to a recent KPMG report ‘AI in financial reporting and audit’, 75% of AI leaders in the financial services have set up policies and governance aligned with the company values and ESG goals compared to only 57% who are not considered leaders. This suggests that another competitive advantage for companies could (surprisingly) be implementing oversight and governance strategies for AI. Despite the fact that legislation and governance are typically perceived as being an obstruction to technological innovation, internal governance for AI systems could in fact be fuelling innovation by helping companies to identify areas where AI can bring the most benefit, and giving them the assurance to innovate more confidently and fully invest in new use-cases for the technology.
Effective data management
Given that data provides the essential fuel for all AI systems, having an effective data management strategy is a crucial practice for any company wanting to see real gains from AI and automation. However, according to data management company, Semarchy, effective data management remains one of the greatest challenges for businesses today, and can be a key barrier to seeing significant ROI on AI investments.
“Businesses typically have too much data and cannot meaningfully organize and digest it in a meaningful way even for even basic analytics and intelligence, let alone training AI. Many of these LLMs actually require less broad datasets, but more focused, accurate datasets to achieve better results. Additional noise from bad, unorganized data not only reduces AI efficacy, but also increases resourcing cost to train and run them in production.”
Steven Lin, Product Marketing Manager at Semarchy
For this reason, AI leaders are investing a significant portion of their IT budgets into data management companies such as Semarchy, given that data is so vital to the accuracy of AI model outputs. High-quality datasets are also a must-have for AI leaders due to their fundamental role in innovation, and the fact that they can be used over and over again for an unlimited number of use-cases.
Prioritizing GenAI over other AI technologies
KPMG’s AI in financial services report also found that while data and analytics are the top priority for the majority of organisations, GenAI is the top priority for AI leaders. Indeed, GenAI brings unprecedented speed and accuracy to many aspects of the finance industry including auditing, risk assessment, fraud detection, and customer service. And this is not unique to this industry – GenAI brings a wealth of benefits for all industries, and while the technology is not yet perfect, AI leaders are not waiting around until perfection is achieved. This demonstrates that adaptability, tolerance for imperfection, and a willingness to take risks are crucial elements of the approach adopted by AI leaders, particularly when it comes to innovation.
Collaboration with external customers and partners
Collaboration is another key strategic advantage that AI leaders tend to display, due to the benefits of pooling resources. A specific example of this is highlighted in KPMG’s report, which found that there may be ‘virtuous cycle’ of AI learning that occurs between companies and auditors. For AI leaders, there seemed to be a particularly positive attitude towards collaboration and the sharing of knowledge, with nearly two-thirds saying that their auditors understand their use of AI ‘well’ or ‘very well’ compared to approximately just half of non-leaders. This indicates that AI leaders prioritize collaboration with their partners and customers when it comes to AI to a great extent, suggesting that this is a key aspect of successful innovation and application of AI.
Expert advice on what not to do
We can learn a lot from ‘AI leaders’ on best practices for leveraging the power of AI in more ambitious and creative ways to see greater value from this powerful technology. Equally, we can gain a great deal of insight by learning from example of what not to do.
Padraig Coffey, CEO of Zartis, a software company focused on consultancy services, and Dave Boundy, COO of energy solutions company, Innowatts, highlight a couple of key mistakes that businesses can make when scaling their operations with AI.
“I think the risk [with scaling operations] is of organisations trying to be something that they’re not. So in the case of a startup, this would be trying to do things in more of an enterprise mode – over-complicating things, maybe in terms of the way in which they build technology, the way in which they adhere to regulations, they might overdo things. Equally, the start-up world is really cool and shiny, so sometimes people in enterprise organisations want to behave in that start-up way, they want to go fast and break things, but it’s like, well, you’re a bank, so you might want to be careful about breaking things.”
Padraig Coffey, CEO of Zartis
Similarly, Boundy points out the potential pitfalls of over-ambitious scaling practices, illustrating the dangers of this with the example of Quibi, an online streaming company founded in 2020 which was forced to shut down less than a year later.
“They [Quibi] raised $1.75 billion, and the goal was to have 7 million customers in the first year. That was the key driver of business. They went about setting up to scale to 7 million customers, they bought content to scale to 7 million customers, and they invested massively in technology and brought in all these people to help them scale. By the time they closed, they had only 500,000 customers, and had burned through their $1.75 billion, and so their investors cancelled. So that’s an example of a company who got it wrong, investing too much too soon in scalability.”
Dave Boundy, COO of Innowatts
These snippets of advice from seasoned experts in their fields highlight one key takeaway in particular: a business’ understanding of its own successes and failures is key to making wise and strategic decisions, and knowing where AI can bring the greatest value. This is particularly crucial for AI leaders, given that effective innovation requires experience, resilience, and regular evaluation cycles.