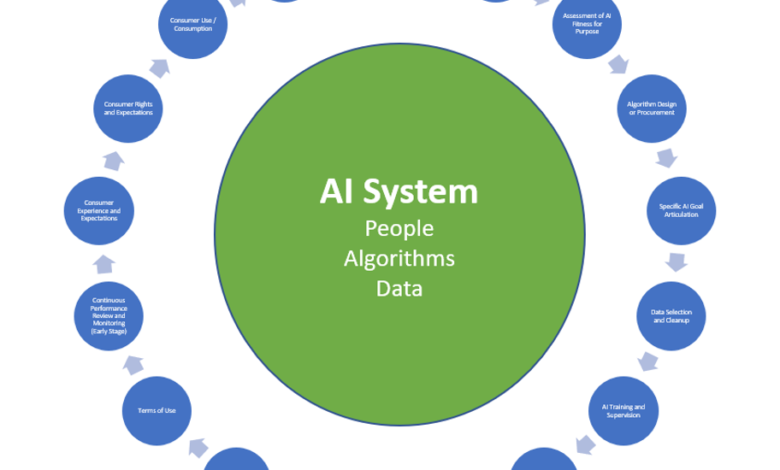
Any non-technical person sitting through an explanation of artificial intelligence (“AI”) or its most common flavor today, machine learning (“ML”), has been advised at some point that ensuring a “human in the loop” is the secret to using AI safely and successfully. This is confusing short-hand and often diverts the broader discussion we should be having about the many different roles we play in improving AI’s functioning and responsibile use. This broader discussion is especially critical because almost all AI in use today and for the foreseeable future, will augment human function and require our participation, not replace it.
Usually “human in the loop” is used in an overly-narrow way to mean that for certain highly consequential AI-driven outputs – think filters (or decisions) on loan applications, college admissions, hiring, medical diagnoses, and so forth – humans should oversee and remain accountable for the AI’s outputs and their alignment with the user’s expectations, standards and legal obligations. This is a central theme in the EC Proposed Regulation for AI .
There is no single understanding of what “human in the loop” means however. Sometimes it refers to an effort to ensure that humans (not AI-agents) are the decision-makers; sometimes it refers to an effort simply to require that humans review and assess the AI’s overall performance or output against expectations. Sometimes it means a team of humans, but almost never does it mean all humans. And therefore the concept does not do justice to all of the ways humans interact with AI to impact its design, functioning and effect. Nor does it describe the more ubiquitous obligation on all of us, to become smarter and more involved in how the technology around us is working and deployed.
Backing up. The power of AI/ML is the breadth and speed at which it can process massive volumes of data and thereby generate predictions – predictions that are based on so many different data points at once that we interpret them as insights and connections that meet (and increasingly exceed) the human ability to do the same. Similarly, the risks of AI/ML include that these outputs almost inevitably exceed our ability to understand how they are generated and almost inevitably include errors and unintended biases while nonetheless scaling rapidly. These risks aren’t necessarily the result of lax human oversight (although that too). Such errors and unintended biases are inherent in the technology. They can arise from
a) data sets and the data within them that are artifacts of history and tend to preserve errors and encode and exacerbate biases of the present and past,
b) engineering choices that are forced to reduce complex contextual activity to code and inevitably introduce designer and/or developer assumptions into any algorithmic model (e.g., how an engineer describes a red ball (or other simple object) in code and how a human would describe it in analog life are very very different) and
c) human instructions that are unclear, full of assumptions (cultural and otherwise), imprecise, overly narrow, overly broad or just misunderstood.
So simply inserting a human at the end of such a system is a pretty insufficient way to think about accountability and AI. It is bound to miss most of the key moments when AI can be designed to work better, engineered to be safer and deployed to be more trustworthy.
Taking the issue of human communcation struggles alone, sometimes this is hilarious: for fun, enjoy this video of a father trying – using only written instructions – to make a peanut butter and jelly sandwich, or virtually any sitcom ever. On the other hand, sometimes these struggles are frustrating: for instance when asking someone to do something we think is straightforward (e.g., file the forms today, get ready for dinner, set up a meeting, do your homework) we are often met with surprisingly good follow up questions or remarkably off-base results.
Steven Pinker, has documented the “Curse of Knowledge,” which is perhaps the most charitable version of the problem, wherein someone with great amounts of knowledge about a thing cannot see what others do not know about that thing, and consequently he or she fails to communicate very well about it. Sense of Style, Chapter 3 (2014) (consider as well, Tim Harford’s Cautionary Tales podcast about the Charge of the Light Brigade, you will not be disappointed). In all events, this happens all the time, and we are no better (as a species) at giving or interpreting instructions when we are designing or engaging with AI than when we are doing anything else.
Today most AI applications work to augment or supplement human function, not displace it. We are now, and will remain, central to how AI functions for the foreseeable future. We need to approcach these technologies, therefore, with a clear vew of our impact on them, and to ask what we do well and what do we not do well? This is a question we can consider at a species level, a team level, or an individual level.
If we think about AI/ML technology, data and humans all working together – as a sytem – within a specific context, then it is easier to see how the answers to those questions will affect how well those systems work and whom they impact.
So, the responsible use of AI requires we look at the whole system of the technology, the data and the people, in context, wherever humans are either implicated or impacted. Likewise, however well an AI tool is working as a technical matter, how it augments human performance or meets a business purpose is just as likely to be determined by the people surrounding it.
Fundamentally, it is not enough simply to insert human oversight at the end of an AI process and declare success because humans are “in the loop.”
Instead, we can put the AI System right in the middle of the AI Lifecycle Loop and begin to unpack where we fit at each step of the way:
The AI Lifecyle Loop
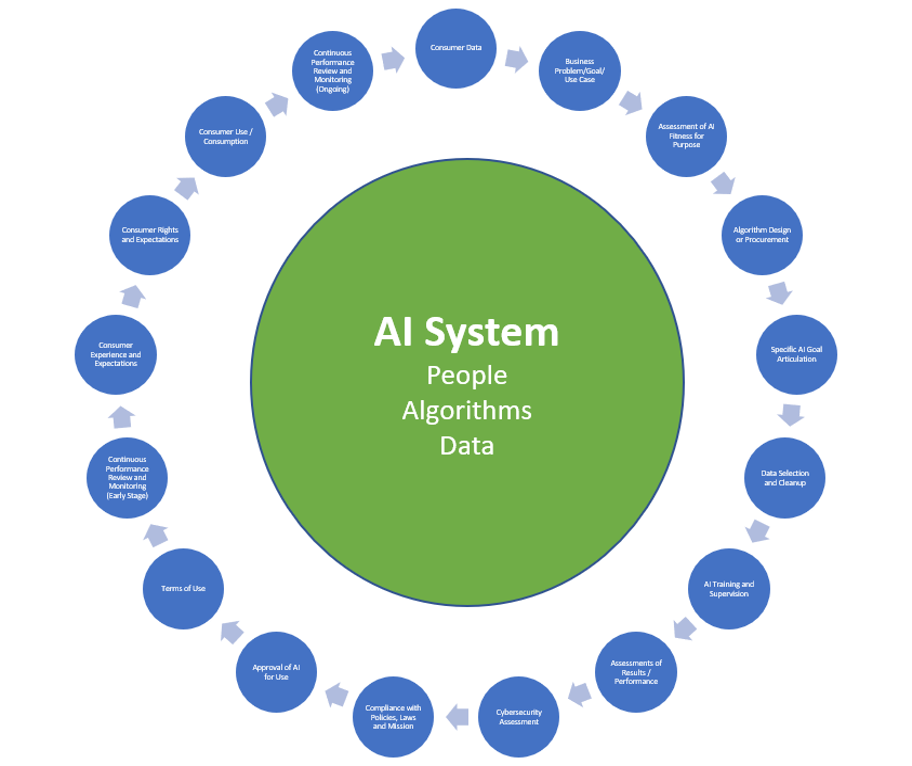
To do that, we can flatten the AI Lifecycle Loop and focus on just the human piece to see our relationships, impact and dependencies at each stage (which is not to say that only humans impact each of these steps; we can do the exercise with technology and data too). While each one of these cells could be a whole discussion, and the intention and purpose of any particular AI model will dramatically affect a chart like this, we can start to pull it apart in general:
The AI Lifecycle Loop Flattened: Human Relationships, Impact and Dependencies
Step of the AI Lifecycle Loop | People | Technology | Data |
Consumer Data | Human behaviors, practices and preferences are the backbone of the data that fuels the AI/ML in use today; humans also assess the data that trains and animates these systems; humans will develop better and better tools for managing the data they produce and consume | AI/ML relies on massive quantities of data about humans to generate predictions, insights and sometimes decisions | Reflects historical human behaviors, practices and preferences (everything from clicks, keystrokes, and eye movements to research, purchasing and sentencing decisions), including historical successes, assumptions, context, biases and errors |
Business Problem / Goal / Use Case | Identify, assess and define the use case, what success and risks look like, where the boundaries are, create the policies and processes for compliance and responsible use | Humans provide the context of what, specifically, it is being asked to do and what its boundaries are | Humans select and remediate data sets that are appropriate to the use case |
Assessment of AI Fitness of Purpose | Determine whether AI is the best tool to address the problem, and if so assess different AI methods for utility against the use case and standards | Humans assess a tool’s capabilities viz the problem at hand and any constraints | Humans determine if data is sufficient, accurate and appropriate; available and properly obtained, administered and secured |
Algorithm Design or Procurement | Design, develop and evaluate readiness and proficiency of the AI tools | Humans develop and design and/or assess | Same |
Specific AI Goal Articulation | Instruct the AI system to optimize for and/or achieve the business or use case and its objectives (including what not to do, or other limits) | Humans instruct and build context | Same |
Data Selection and Cleanup | Determine what data to use, how to label/clean it up (or not) to improve accuracy and bias concerns | Humans provide its understanding of data contents, labeling, quality or utility | Humans select and remediate |
AI Training and Supervision | Design the training and assessment of AI tools | Humans train and guide | Humans input, label, select |
Assessment of AI Results / Performance | Assess the system, use the tools and the results of the AI | Humans evaluate its efficacy and understanding of the risks | Human experience, adjustments and reactions generate new data, now affected by the AI tool |
Cybersecurity Assessment | Assess ongoing risks and mitigation | Humans evaluate novel risks presented by AI | Humans evaluate novel risks, and may be impacted by breaches (or create new risks by intention or carelessness) |
Compliance with Policies, Laws and Mission | Sets the standards, training and evaluation of AI for compliance with rules, regulations, internal policies, other strategies and mission; humans also behave (or don’t) with legal and business rules standards, and generate related data | Humans set compliance standards and articulate its consistent functioning with those standards | Data regarding behaviors and risks, objects and purposes of AI, etc., inform the ability of AI to comply with other rules and regulations; compliance risks and strategies and predictions about conditions that improve compliance (or don’t) |
Approval of AI for Use | Approve the use of AI tools in real world settings with real world impact, strategies for mitigating foreseeable risks and unintended outcomes | Humans approve it for use in real world settings, understand users and provide the contexts in which it will be deployed | Data on prior performance informs readiness of AI for broader use |
Terms of Use | Set the terms of commercial use, liability and risk shifting, IP rights, warranties | Humans set the terms and standards | Human set the terms and standards |
Continuous Performance Review and Monitoring (Early Stage) | Supervise the appropriateness of an AI tool’s function over time, in real world settings | Humans set the cadence and standard for continuous review; some AI audit tools on the horizon | Humans input, label, select, review, assess and interpret AI function, which creates feedback data |
Consumer Experience and Expectations | Manage and hold responsibility for how AI tools function (or don’t) and for their impact | Humans provide context | Humans provide context and feedback data |
Consumer Rights and Expectations | Possess rights (in some jurisdictions) and expectations about how AI operates on and around them | Humans provide context | Data is increasingly subject to regulatory standards for usage and security |
Consumer Use / Consumption | Deploy AI-tools to analyze, predict, determine and serve consumers, employees and others | Humans are impacted by its functioning | Generates and consumes data about humans; creates feedback data |
Continuous Performance Review and Monitoring (Ongoing) | Supervise the appropriateness of an AI tool’s function over longer periods of time and larger populations and conditions | Humans set the cadence and standard for continuous review to keep AI tools adhering to and not overtaking their original purposes | Humans input, label, select, review, assess and interpret AI function, which creates feedback data… |
… And back to Step One and Consumer Data | … | … | … |
All of which is to say, we need to pay attention to the human side of this equation, not only from the get-go but throughout, and not only when it comes to restricting automated decision making. Some of this work simply requires thinking a little differently about how people are impacting and being impacted by the technologies in use. Some of this work, however, requires much more and newer attention to the human aspects of building trustworthy technology.
How to do this is well-beyond the scope here, but as I have written elsewhere, the following is a good and easy-to-remember starting place: the most senior executives i) set the tone and standards for trustworthiness throughout the organization and ii) direct resources to a multi-stakeholder process that focuses very directly on ABC&D:
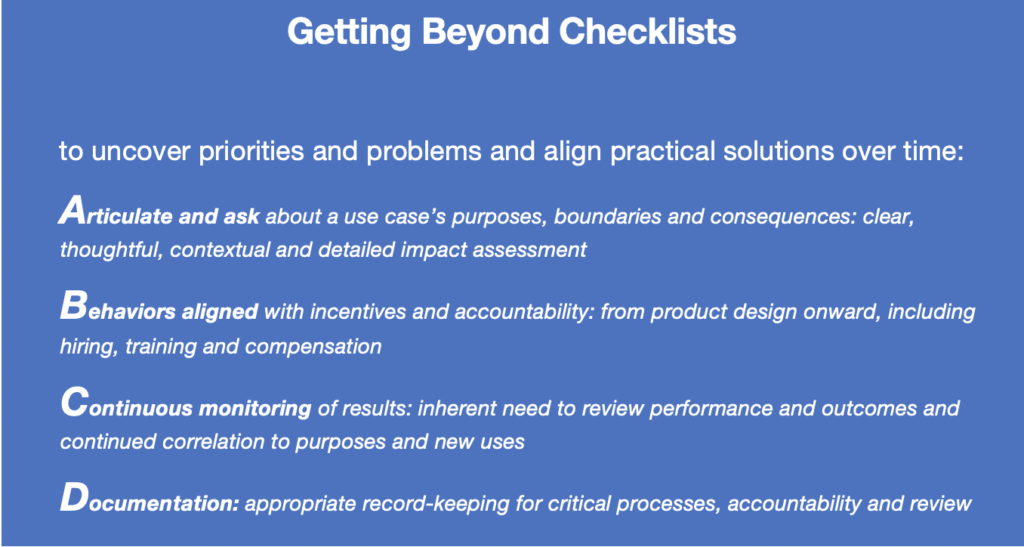