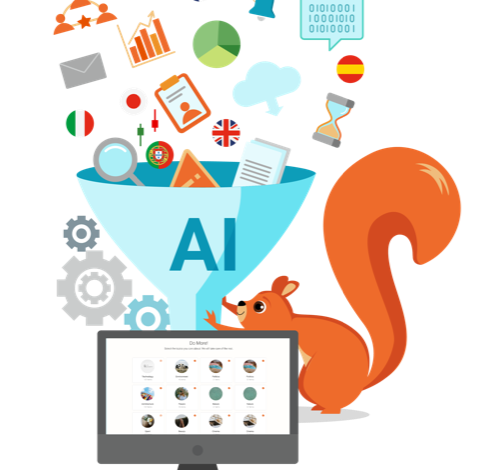
Search is a vital element of what makes the Internet such an essential tool for so many of us. Without the ability to find information in a matter of seconds, it is questionable whether the internet would even have gained a fraction of its ubiquity and popularity. At the very least, it would be vastly different to how it is now.
Every person that uses the Internet will almost certainly conduct at least one search every single day. Most of those will only use one search engine. They type in a keyword or two, are presented with a list of results and usually, do not look beyond the top two or three on the list. This is how we have all been programmed to use search; an approach honed over 20 years of repetition.
However, we are on the cusp of a transformative change in search. Advances in AI mean that the way that search has traditionally worked – people go to the information – could soon be a thing of the past. It will be replaced by a more contextual, relevant search, which does not depend on the user knowing what they are searching for.
The limitations of traditional search
Search has traditionally worked by crawling data, creating an index and providing for a search interface, typically a single field search bar. Trillions of person-hours have been invested by the leading search providers to get the relevance of the result right. It is also something in which I have a long-term interest.
Back in 2004, I co-founded local.ch, the largest homegrown search engine in Switzerland. In the early days, our key metrics were 1.23 keywords as input. That is not even enough for a user to spell out ‘Bern Restaurant’. It was either ‘Be Restaurant’ or ‘Bern Rest’. No one wants to actually be a restaurant, and not many would be searching to take a rest in Bern either.
This has evolved, and query lengths can stretch around two words now. However, the core principle remains the same: users must go to the information. This is not a bad thing per se and has proved an effective way of finding information. The major search providers and more specialised Enterprise Search vendors have added layers of sophistication to this approach, such as ingestion optimisation, profiling, relevance ranking, and many more.
A different approach to search
But the world in which we currently live and work has an overload of information. There is more info than we have even seen or could even imagine, so the ways in which we use to find information are becoming less effective.
To find information, people need to know what they are looking for. Only if a user can enter into the search bar the precise terms of their quest will they find meaningful results. This is heightened even more when looking at Enterprise Search. If someone starts a new job, they are unlikely to know too much about products, customers or prior history. Given most enterprises store information across a multitude of systems, locating information becomes much more challenging.
An entirely different approach to search is required to get to the next level. Instead of users going towards the information, the right insights need to come to them at the moment they need to get their work done. The whole provision of information must be turned upside down.
AI and computationally aware informational objects
For this to happen, computationally aware informational objects are required. This is not to suggest that a data item will develop cognition about itself. But the construed concept of the informational object must express in computational terms the concept and the notion of the underlying information/data.
In essence, the information must be expanded into a concept of an informational object with lots of (probabilistic) meta information to get a level of cognition of its content and meaning. This is not yet another relational schema but a continuous probabilistic re-compute – information changes, situational changes, user preference changes. This is the power of Machine Learning – an ongoing development process, continually learning more about the insight and the user.
The transition of information is followed by the computationally encoding of ID within that object. When this is complete, the object becomes tradable, AI is used to develop cognitive understanding, and the most relevant insights can find their way to the user.
The future of Enterprise Search
The Insights Economy has made the quick retrieval of the correct information more important than ever. Enterprises cannot afford for their employees to spend too long on finding information when their business models are partially or wholly dependent on how data is deployed for competitive advantage.
That’s the reality that will make traditional Enterprise Search obsolete. But by creating computationally aware informational objects, a new wave of Enterprise Search is upon us.
When we started local.ch, the first three restaurants in Bern we delivered as search results were not necessarily the best. Rather, they were the ones the ranking algorithm decided were the most relevant to a user’s search and which matched their search profile. This concept can be extended to think of search as akin to a continuously cooked bouillabaisse. The system automatically picks and delivers a bowl when it is time for lunch. So next time when you open your phone three restaurant recommendations tailored to your taste, whereabouts, calendar and time availability will already be displayed on your phone.
This is the AI-powered future of Enterprise Search.