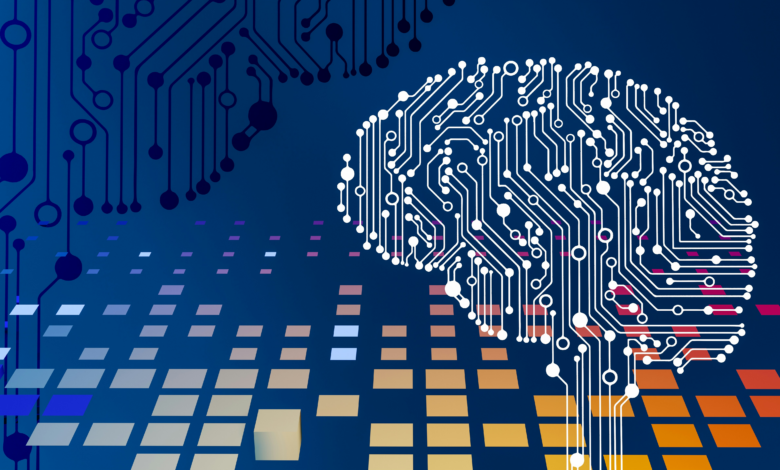
Artificial Intelligence (AI), initially a speck in global innovation, has emerged to become a powerful force in reimagining the way we do business today. Organizations across the globe are now seeing beyond the looking glass, witnessing remarkable productivity gains— some even up to 42% in software development alone, telling a compelling story of efficiency and progress.
Beyond AI’s resounding success lies a paradox that keeps tech leaders on their toes: the conflict between enterprise vision and readiness continues to prevail, with about 87% of executives acknowledging the critical importance of responsible AI deployment and a mere 15% feeling truly prepared to implement it effectively. |
This dichotomy presents a complex challenge – the urgent need to bridge the gap between AI’s potential and its practical, responsible deployment.
The year 2024 marked a defining chapter in AI transformation. Global service integrators found themselves at the confluence of two powerful forces: the explosive growth of Generative AI and the maturation of AIOps. Together, these movements rewrote the rules of efficiency and innovation in software development. And with this power came profound responsibility, raising questions that echo through boardrooms and development chambers alike: How do we measure the actual value of our AI systems? Which processes should remain in human hands, at the potential cost of efficiency? How do we balance automation with workforce evolution?
From the perspective of opportunity and responsibility, three fundamental hallmarks have emerged as the cornerstones of successful AI deployment. User centricity, transparency, and robust guardrails form both a framework for implementation and a philosophy towards driving AI that serves its highest purpose: enhancing human capability while preserving faith in human values.
User centricity: The foundation of AI success
AI exists to serve people, and its success depends on prioritizing user rights, experiences, and well-being. True user-centric AI goes beyond intuitive interfaces; it integrates seamlessly into daily workflows while maintaining user trust through ethical design and robust feedback systems. For instance, when an AI system with strong privacy protections is deployed, users not only get better data security but also experience more personalized services because they feel comfortable sharing relevant information.
User-centered design principles are fundamental to developing effective artificial intelligence systems that deliver genuine value. This foundation must be built upon a solid strategy that embraces accessibility for all users, ensures consistent reliability, and accounts for a diverse user base. Success in the space also hinges on delivering increasingly accurate results while maintaining transparency through clear terms and conditions, implementing progressive disclosure to avoid overwhelming users with information.
- Building user trust through experience
Recent data paints a sobering picture: only 23% of organizations feel highly capable of addressing user adoption and change management in their AI initiatives. This highlights a critical gap between technological capability and practical utility. The challenge isn’t just about deploying AI; it’s about deploying AI that users trust, understand, and willingly integrate into their workflows. Building this trust requires transparency. Organizations must prioritize clear terms and conditions, progressive disclosure of AI capabilities, and consistent accuracy in AI outputs. Transparency must be coupled with tangible benefits, such as robust privacy protections, which increase user engagement and personalization.
- Drawing on the human-AI partnership
Adaptive interaction systems that integrate with existing workflows are key to bridging the adoption gap. This requires context-aware ethical frameworks that navigate complex decision-making scenarios while respecting user preferences and organizational values.
Successful AI implementations also feature robust feedback integration systems that create dynamic feedback loops. Organizations leading in AI adoption have established mechanisms to collect and translate feedback into meaningful system enhancements, driving a cycle of improvement and adoption.
Finally, user centricity extends to the teams developing these systems. Organizations must prioritize diverse AI development teams, bringing varied perspectives to system design, preventing bias, and ensuring effective service for diverse user populations. Comprehensive AI literacy programs must ensure that everyone, from developers to end-users, understands capabilities and limitations of AI.
Measuring success in user-centric AI deployment involves tracking user satisfaction, adoption rates, and the impact on workflow efficiency and job satisfaction. This holistic approach ensures AI systems enhance human capabilities rather than replace them.
Transparency: The key to sustainable AI adoption
Organizations navigating AI deployment usually face the challenge of harnessing AI’s potential while maintaining stakeholder trust with adequate transparency. This extends beyond disclosing AI usage to encompass data privacy, compliance frameworks, and nuanced communication about features leveraging user information.
Enabling AI transparency requires a comprehensive strategy built on four pillars:
● Effective clarity: With open communication about data usage and privacy, we build informed confidence, helping stakeholders grasp AI’s capabilities and limitations.
● Stakeholder empowerment: Transparency fosters a dynamic partnership, empowering users to control AI interactions, enabling developers to craft ethical, user-aligned solutions, and supporting regulators in balancing innovation with oversight.
● Risk management: Leveraging transparency serves as an early warning system, with clear documentation and communication enabling organizations to address issues proactively, avoiding AI misuse or overreliance. ● Sustainable growth: Finally, with transparency at the pinnacle of AI adoption, we accelerate user acceptance with open feedback channels, driving continuous improvement for the road ahead. |
For example, organizations like Adobe, with its transparent approach to AI-powered tools like Adobe Firefly, demonstrate how openness can drive user engagement and sustainable competitive advantage. Therefore, it’s important for organizations to adhere to:
- Cataloging AI models and data: Establishing a comprehensive catalogue of AI models and data is foundational. Regular algorithmic and data reviews help maintain the level of transparency and consistency.
- Ensuring repeatability and observability: Observability allows stakeholders to monitor model behaviour and outputs, fostering trust in AI’s consistency and fairness.
- Clear communication of AI capabilities and limitations: Transparent systems require clear communication about what AI can and cannot do.
As organizations navigate the complexities of AI deployment, it is imperative to explore an emerging frontier: AI agents. These autonomous systems represent a quantum leap from traditional AI implementations, bringing both enhanced capabilities and heightened responsibility for transparency. The challenge now extends beyond merely disclosing AI usage to fostering trust in systems that can make increasingly sophisticated decisions.
By adhering to these strategies, organizations can build transparent AI systems that enhance trust, ensure compliance, and foster sustainable adoption.
Guardrails: Enabling safe and ethical AI operations
At the heart of responsible AI lies a comprehensive layer of protection that transcends mere compliance checkboxes. Laying the foundation begins with new-age algorithmic impact assessments (AIAs), which serve as the first line of defense by evaluating potential consequences before deployment. Consider, for instance, the critical role of AIAs in healthcare settings, where they can spotlight potential biases that could skew treatment recommendations across different demographic groups. This evolution in AI safety has naturally led to the emergence of sophisticated bias detection systems that work in tandem with AIAs, building on a continuous monitoring mechanism that vigilantly watches for prejudicial patterns in both data and decisions.
Building upon these foundational elements, organizations must also implement robust containment protocols. These protocols serve as sophisticated safety nets, establishing clear boundaries for AI operations and preventing systems from exceeding their intended scope or making unauthorized decisions. We could think of them as digital guardrails that not only keep AI systems within predefined ethical and operational parameters but also adapt to emerging challenges.
In this layered approach to AI safety, watermarking has emerged as a powerful verification tool. This technology enables organizations to track and authenticate AI-generated content with unprecedented precision—a capability that proves invaluable in today’s landscape where the line between human and AI-created content grows increasingly blurred.
While these protective measures form the cornerstone of responsible AI deployment, their effectiveness hinges on implementation through robust safety systems. This requires organizations to establish clear governance structures with dedicated oversight mechanisms, including AI ethics boards and well-defined chains of responsibility for AI-related decisions.
Supporting these governance structures are rigorous testing and validation procedures that must evolve continuously. Through regular reviews, penetration testing, and performance evaluations, organizations can ensure their AI systems not only maintain intended functionality but also align with emerging regulatory frameworks like the EU’s AI Act and other global standards.
Completing this comprehensive safety architecture are incident response and mitigation strategies. These strategies represent the last line of defense, requiring organizations to maintain clear protocols for investigation, containment, and remediation. By establishing dedicated communication channels and predetermined response plans, organizations can react swiftly and effectively to any AI-related incidents that may arise.
Charting the path forward
The journey toward responsible AI deployment involves integrating user centricity, transparency, and robust guardrails, building something of a flywheel effect: user-centric design informs guardrails, transparency fosters trust, and protective measures encourage complete and holistic engagement with AI systems.
At the forefront of this evolution, we, at HCLTech, are dedicated to helping enterprises embrace responsible AI while maintaining the highest standards of ethics and sustainable growth. Our offerings span three arcs: service, value chain, and technology transformation— starting right from ideation to scaled deployments.
Our commitment to AI excellence is further exemplified by our solutions, such as AI Force, an intelligent GenAI-driven platform that revolutionizes the software development lifecycle by balancing automation with human intuition. AI Force delivers 360-degree productivity gains for stakeholders across the development spectrum, systematically reducing technical debt while elevating code quality. This approach stems from our foundational capabilities in AI and GenAI engineering, enabling us to deliver continued business impact for global enterprises.
As enterprises worldwide accelerate AI adoption, responsible AI practices and partnerships become increasingly crucial. The future of AI belongs to those who deploy it responsibly. The time for responsible AI deployment is now.