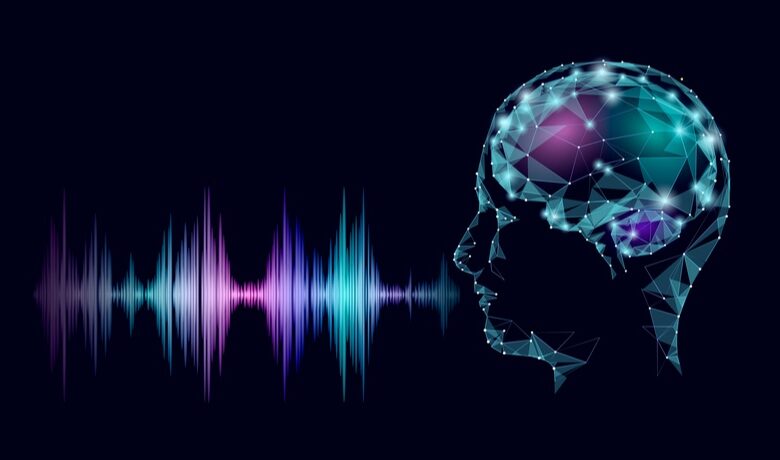
Text to speech generators have become inseparable from peoples’ daily lives. They have not only transformed how people interact with machines but also became their valuable asset. From providing greater accessibility to educational content to visually impaired students to offering hands-free audio-enabled directions while driving- this technology provides a lot. While remarkable, have you ever wondered what fuels this cutting-edge technology or what goes behind the scenes to deliver such excellent results? This article will give you a glimpse of the processes that never make it to the front while adding tremendous value to your lives, so read the full article.
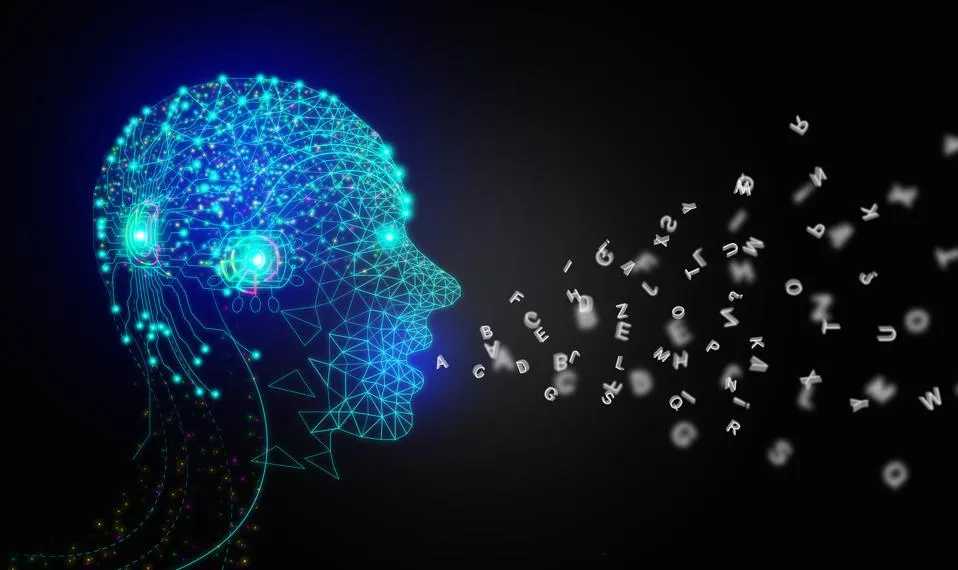
Understanding the Fundamentals
The technology behind text to speech AI is complex and sophisticated, so it is best to understand the fundamentals that laid the groundwork for this technology. The entire technology is built on speech synthesis, which has evolved and continuously progressed. It started with a concatenative synthesis that stitched together pre-recorded speech fragments to form complete utterances. While this approach delivered satisfactory results, it was limited by the size and quality of the speech corpus used.
Another essential element of this technology is formant synthesis, designed to recreate speech signals by modeling the vocal tract’s resonances. While it offered flexibility and control, it lacked the naturalness of human speech. Next came the statistical parametric synthesis, a sophisticated approach utilizing advanced machine learning techniques to model the relationship between acoustic parameters and linguistic features. It helped generate more natural-sounding voices.
The advancements in this technology noticed a steep rise with the introduction of neural networks. These powerful models could make complex mappings between text and speech, paving the way for more advanced and realistic speech synthesis.
Deep Dive Into Essential Architectural Components
All modern text to speech engines are intricate systems composed of various vital elements. While each component is essential and plays a distinct role in transforming text into speech, the following architectural components are necessary for the following reasons:
- Text Analysis and Normalization
All written words undergo a thorough analysis and normalization process before any speech synthesis occurs. This step involves many tasks, such as tokenization, expansion of numbers and abbreviations, handling special characters or symbols, etc., for text normalization. It facilitates producing intelligible and natural-sounding speech.
- Linguistic and Prosodic Modeling
Once the written text is normalized in the above step, the engine starts its linguistic and prosodic modeling to determine appropriate pronunciations, patterns, and intonations. These models use statistical methods to capture language complexities and generate natural-sounding speech. They also factor in aspects like speech tagging, phrase boundaries, emphasis placement, etc., for the best results.
- Acoustic Modeling
It is a crucial component of all text to voice free tools because it generates the actual speech waveform from the previous stage, i.e., linguistic and prosodic information. Earlier, machines relied on complex signal processing and concatenating pre-recorded speech units, but now, it has been replaced with modern neural network-based acoustic models. These models directly synthesize speech waveforms, offering superior-quality speech synthesis.
- Waveform Generation
This is the last stage of the text to speech process, in which the actual speech waveform develops. Previously, voice coders were used to reconstruct the speech signal from acoustic parameters, which are now replaced with neural voice coders, like WaveNet and its variants. This has significantly improved the quality and naturalness of synthesized speech.
All these architectural components work in harmony to transform text into speech, leading to a seamless auditory experience.
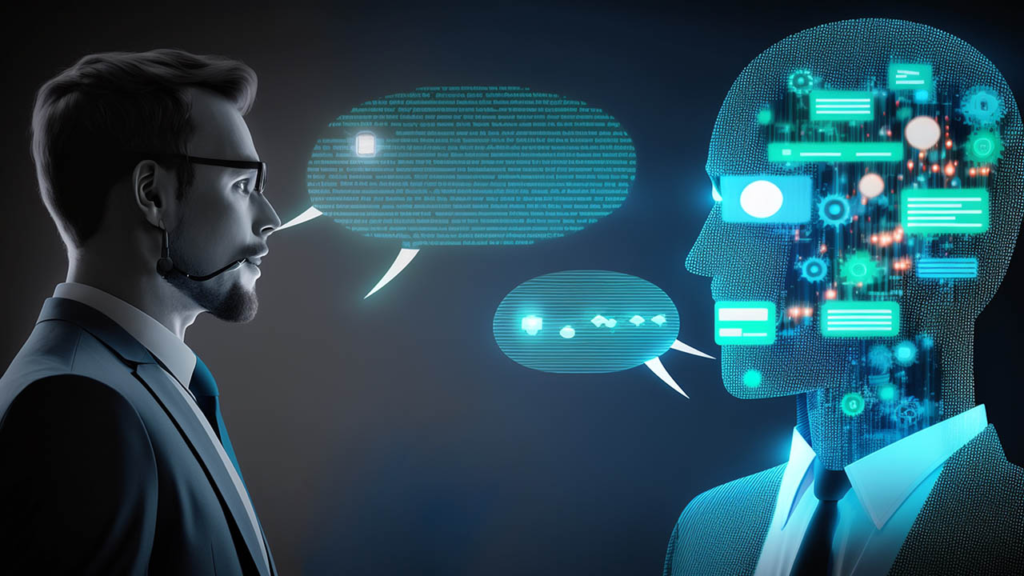
Advancements in Neural Text to Speech
While all advancements in the text to speech journey are remarkable in their own light, the emergence of neural networks deserves special mention. Previously, text to speech machines relied heavily on complex signal-processing algorithms and extensive manual tuning. However, the launch of neural text to speech brought a radical shift in this approach. These new models now harness the power of deep learning to understand the intricate relationships between text and speech directly from data.
It has led to massive improvements in the naturalness, expressiveness, and overall quality of synthesized speech. A pioneering advancement in this domain was the introduction of end-to-end models like Tacotron and its variants. These models streamline text to speech processes by combining various components like linguistic modeling and acoustic synthesis into a single neural network architecture. End-to-end models join these tasks to capture more nuanced patterns and generate more natural-sounding speech.
Neural voice coders were another significant development in this field because they could directly model the raw audio waveform for creating more natural and realistic speech synthesis. Incorporating attention mechanisms in the process was another breakthrough because they supported the process by improving the handling of long and complex sentences and better capturing contextual information and prosodic patterns.
Embracing the Power of AI-driven Sophisticated Voice AI Models
Text to voice generators have influenced every area of people’s lives. What’s impressive about these tools is their underlying technology and its evolution from concatenative synthesis to neural networks. While the architectural components of this process present text to speech engines as a testament to the ingenuity of researchers and developers, evolved concepts like attention mechanisms, neural voice coders, and end-to-end models did an excellent job of infusing a layer of realism and quality, blurring the lines between synthetic and human speech.