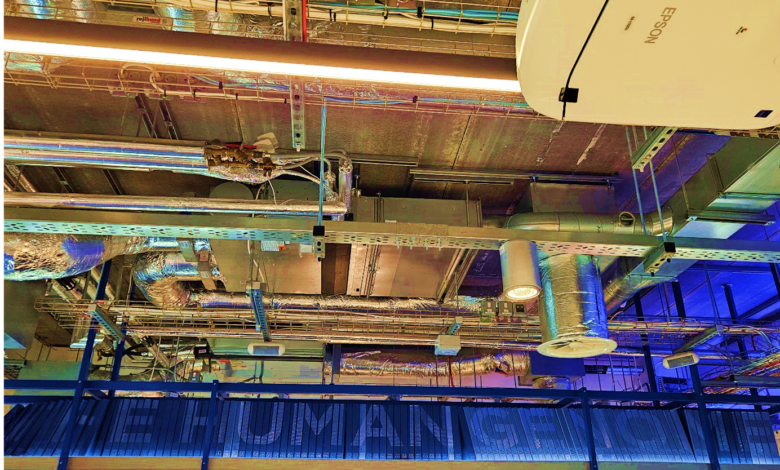
Tucked away in a small conference room at the Wellcome Sanger Institute, a few miles outside of Cambridge, lies a set of blue hardback books which house the core recipe of our DNA: the human genome.
But this old-school presentation of our biological code is really just for show. For practical access and storage reasons, the full library of genomic data for humans (as well as for 323 other species) is now stored digitally and annotated automatically through the AI-powered genome browser, Ensembl.
But the presentation and annotation of this data is but the tip of the iceberg when it comes to the applications of AI in genetic research. In fact, in the room right next door to these books, human lab researchers work alongside AI-powered sequencing machines in utopian harmony, bound under the common goal of breaking down physical samples from different organisms into invaluable data about their genetic make-up, and the function and structure of individual cells.
In this article, we delve into the crucial role of AI technologies in genetic research, bringing you expert insights from Schneider Electricās Press Conference, hosted on Wednesday (25th September 2024) at the Wellcome Sanger Institute, an internationally renowned research facility situated near the top of the UKās Innovation Corridor.
In particular, we consider how the role of AI has expanded in this area in the last few years, where it could go in the future, and the specific challenges of using AI in genetic research.
Mapping the human genome & its significance
The mission to charter the complete genetic make-up of human DNA started out as an international research initiative known as the Human Genome Project (HGP), which was carried out in twenty universities/research facilities across six countries.
With the project kicking off in 1990, it took researchers thirteen years to map out the first complete version of the human genome, published in 2003. Thirteen years might seem like a long time to us now, but this timeline was actually two years ahead of the schedule ā and this was primarily thanks to the use of AI technologies to automate the genomic sequencing process.
Now, due to recent advancements in AI alongside the availability of increasingly powerful processing infrastructure, the mapping of a human genome can be achieved in just one hour. This has enabled researchers to further genetic research in two crucial ways:
- To sequence the genomes of all eukaryotic organisms so as to identify and preserve the diversity of life on Earth – see the Tree of Life (ToL) Programme. Currently, genomic sequence data is available for approximately just 0.8% of Earthās species, highlighting both the ambition and importance of this project in protecting our planetās ecosystems.
- To sequence the unique composition of each individual cell found in the healthy human body in order to better understand and treat disease ā see the Human Cell Atlas (HCA) project.
While the HGP is widely regarded as one of the most ground-breaking achievements of 21st Century biology, these follow-on projects are arguably where genomic research could have a more direct impact on both the health of humans and the planet.
As Muzlifah Haniffa, Senior Research Fellow at Wellcome Sanger & Director of the Human Cell Atlas Project, explains, the DNA contained inside every cell actually tells you very little about how cells specialize for particular functions, and therefore how human tissues and organs develop. In this sense, the HGP, while a landmark achievement in itself, was really only the first step towards accelerating the study of human disease.
āThere is one problem in the sense that we have 37 trillion cells, but in every one of those cells, which collectively comprise all of the different tissue types found in the human body, we just have the one genome. Essentially this means that an identical copy of DNA in all of those cells is somehow able to create a huge diversity of cell types. This happens because different parts of the DNA are activated in the different cell types, which gives rise to the heterogeneity and differential functions of each cell type. What we can now do [with the Human Cell Atlas project] is begin to understand the different cells that make up the human body, where they are, and how they interact together to assemble into tissues and organs, and enable us to do everyday activities. But the focus isn’t just on how these cells keep us healthy, itās also about what goes wrong in disease ā and thatās really important because this is how we’re going to prevent and cure disease, find treatment targets, and continue to lead healthy lives.ā ~ Muzlifah Haniffa, Senior Research Fellow at Wellcome Sanger & Professor of Dermatology and Immunology at Newcastle University
From a more environmentally oriented perspective, Mark Blaxter, renowned author & Programme Lead of the ToL project, views the mapping of the human genome as just the first stepping stone within the bigger picture of collecting the genomic data of all of Earthās species. This, he argues, will be of critical importance for promoting biodiversity in the face of environmental change and promoting sustainability across human societies.
āWe’re living in an era of unprecedented technological change, especially when it comes to genomics. The Wellcome Sanger Institute was built on the basis of sequencing genomes, and while its first product thirty years ago was the sequencing of the human genome, we’re now proposing to sequence all eukaryotic life on Earth (estimated to consist of around 1.65 billion species). With this wealth of species comes a wealth of really exciting biology, so we will be able to understand life as we’ve never understood it before. This is going to be of critical importance as we face the future, so we have to do everything we can to preserve biodiversity. We have to not only conserve and maintain, but also possibly actively promote biodiversity so that this biodiversity continues to provide the ecosystem services on which human societies rely. We also want to also use the diverse genomes found in nature for biotechnology, which will enable us to discover new drugs and biomaterials that might be of use to human societies in the future.ā ~ Mark Blaxter, Programme Lead for the Sanger Instituteās Tree of Life project
How have technological advances shaped genetic research?
Despite the initial reservations of the original researchers involved in the HGP, a wide range of technologies are now used to automate gene sequencing processes. Below, we look at how these technologies works, how the process of genomic sequencing has become more efficient over time, the current limitations of the technology, and where AI comes into play.
In particular, we outline the role of AI in processing and managing data, and how Generative AI tools can help increase accessibility to this emerging source of big data.
The automation of genomic sequencing
The first proper method for genomic sequencing was a manual technique known as the āSanger Methodā, established 1977 by the Nobel Prize winning Frederick Sanger. At the time, Sanger Sequencing represented a major breakthrough in genomics, involving some pretty advanced biological techniques which centred around chemical manipulation of the DNA samples to break them up, and the subsequent labelling of the fragments with radioactive molecules for identification. This process, while highly innovative for the time, was extremely time consuming and prone to human error, given that all the data had to be manually collected and analysed by eye.
The first use of automated sequencing came in 1987, when two immunology researchers, Leroy Hood and Michael Hunkapiller, created the first gene sequencing machine based predominantly on the Sanger Method out of frustration at how much of their time was spent on genomic sequencing. This triggered a huge spike in efficiency, with the first machine (the AB370A) able to sequence 96 samples simultaneously, read 500 kilobases per day, and read strand lengths of up to 600 bases.
Since then, several more genomic sequencing machines have been released, each with slight variations in technique and efficiency. In particular, since the completion of the HGP and the surge of interest this brought to the field of genomics, manufacturers have focused on developing cheaper and more accurate platforms with higher throughput. These platforms, collectively known as next-generation sequencing (NGS) technology, are now the commercial standard for genomic sequencing, and are used for not just genomic research, but also in fields such as forensic science, agriculture, and reproductive health.
More recently, a third generation of genomic sequencing technology has also been introduced. This differs from NGS technology in that they do not require the chemical amplification of DNA. Not only does this reduce sample preparation time, but it also reduces the margin for human error. Pacific Biosciences, for example, have developed a technique called single-molecule real-time (SMRT) sequencing, where the data is generated via the capture of light emission patterns triggered by a chemical reaction involving the DNA strands.
Oxford Nanopore Technologies, meanwhile, have developed a different type of sequencer based on nanopore sensor technology. This technology is now the most powerful for method for generating ultralong reads from samples, and is unique in the sense that it does not require the DNA fragments to be chemically labelled. Currently, this technology can sequence between 10,000 ā 1 million DNA base pairs in one go.
Nevertheless, even this impressive throughput is not enough to be able to sequence the whole of an organismās genome in one go. The human genome, for example, consists of approximately 3 billion base pairs. As youād expect, this number is lower for smaller and less complex species, but even the fruit fly genome has 132 million base pairs, while bacterial genomes range from size from approximately 4-13 million base pairs, both of which are well beyond the scope of even the latest nanopore sequencing technology.
Blaxter explains that, for the moment at least, this means that samples still need to go through the process of chemical fragmentation. This then requires the reassembly of the output data in order to generate the complete genome of a species.
āIt’d be wonderful if we had instruments it could read the whole of an organism’s genome in one go, but we don’t have them yet. For this reason, we have developed specific ways of sequencing genomes, which involves first of all, smashing the genome up and then using computational tools to put it back together again.ā ~ Mark Blaxter, Programme Lead for the Sanger Instituteās Tree of Life project
Arguably, the dependency of genomic sequencing on the predominantly manual procedure of chemical fragmentation is a significant bottleneck restricting the full automation of genomic sequencing. However, with advancements being made in computer vision, and the integration of more sophisticated AI technologies with robotics, even this part of the process could soon become fully automated.
Companies such as biotech suppliers, Promega and Illumina, for example, are now offering automated solutions alongside more traditional manual kits to optimize various stages of DNA fragmentation.
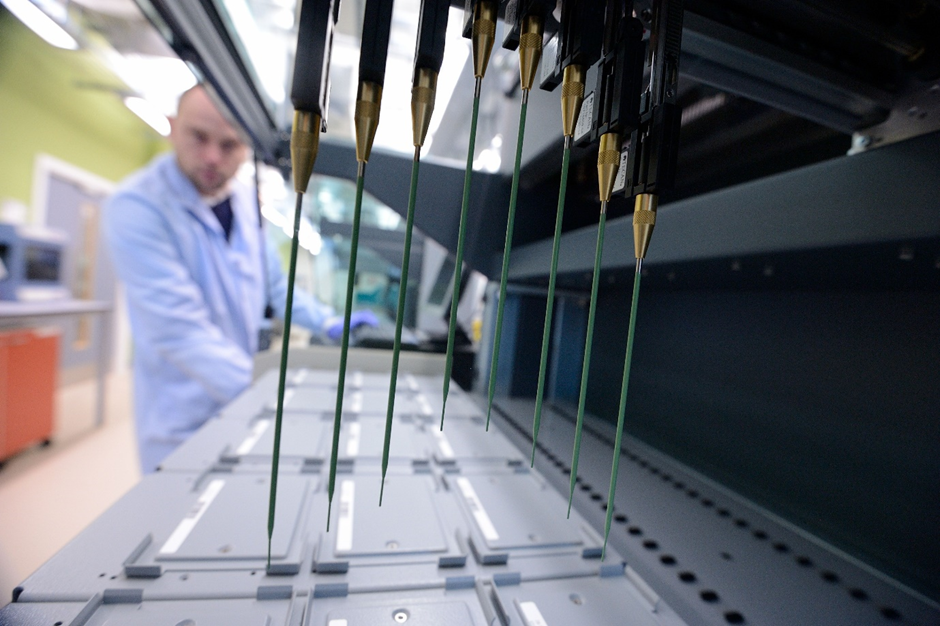
Above: A lab researcher using an Illumina machine to prepare DNA samples for sequencing. Image at the courtesy of the Sanger Wells Institute.
The developmental trajectory of genomic sequencing over the last few decades remains significant today not just because of the gains it has brought to the field of genomic research and the resultant understanding we have of the human body.
Beyond this, it illustrates the transformative power of automation in driving efficiency in life sciences research, highlighting just how easily AI technologies can lend themselves to a vast array of specialized procedures, especially when combined with cutting-edge science.
Indeed, from being an extremely time-consuming, manual process back in the 1970s to a highly efficient and scalable automated process today, genomic sequencing is an inspirational example of how AI can be used to accelerate human efforts to expand the frontiers of scientific research and open up a whole new world of big data found within each human cell.
Advances in single-cell transcriptomics
Beyond automated gene sequencing, technological advances have also been crucial to the development of a related area of genetic research known as single-cell transcriptomics. This refers to a branch of techniques used to uncover gene expression, the process by which the genomic information encoded in our DNA is translated into proteins that are specialized for various functions in different types of cells.
This is a key focus of the HCA project, which aims to uncover the structure and function of each type of cell in the human body.
āHow do we find out the types of cells that make up the human body? Well, technology is always at the centre of it. It was [automated] DNA sequencing that allowed us to define the human genome and complete the HGP ahead of schedule. Now, technology is enabling us to read all of the RNA, [the nucleic acid] that individual cells make as a surrogate for their identity and function.ā ~ Muzlifah Haniffa, Senior Research Fellow at Wellcome Sanger & Professor of Dermatology and Immunology at Newcastle University
Within the realm of single-cell transcriptomics, much of the technological innovation has focused on overcoming what was traditionally a significant bottleneck for gene expression research: the challenge of isolating cells from their natural environment without triggering stress, cell death, or aggregation.
āEarly on, when the technology first came about that enabled us to read the RNA of individual cells, you had to break apart the tissue so that you were left with individual cells. This worked really well for circulating cells in the peripheral blood, but it was a bit of a nightmare if you had to take skin or the heart, because in these contexts, the cells are forming a tissue. For these types of cells, in the process of dissociating them, you’re disrupting the key interactions they carry out, and you also end up changing their morphology or appearance. For example, they might be long and spindle shaped, but once you’ve ripped them apart, they kind of become circular.ā ~ Muzlifah Haniffa, Senior Research Fellow at Wellcome Sanger & Professor of Dermatology and Immunology at Newcastle University
Now, thanks to a developing field of techniques known as spatial transcriptomics, the RNA sequences of individual cells can be recorded in-situ (i.e. in the tissue). Spatial transcriptomics thus preserve the natural structure and contextual information of cells, i.e. where the cell is located relative to neighbouring cells and other non-cellular structures.
āThis technology [i.e. spatial transcriptomics] has mainly just come about within the last five or six years, so itās pretty recent. And what it does is allow you to look at the cells in a tissue in much the same way that a pathologist or a doctor looks at biopsies for tissues in hospital. At the moment, we have a chemical reaction that tags mRNAs with different shades of pink and purple so that we can read all of the genes that are expressed by those individual cells ā and the potential of this is enormous.ā ~ Muzlifah Haniffa, Senior Research Fellow at Wellcome Sanger & Professor of Dermatology and Immunology at Newcastle University
Early attempts to carry out spatial transcriptomics involved making targeted screens of specific mRNAs which could then be used to infer information about cell structure and function. Nevertheless, these attempts not only lacked efficiency and scalability, but only enabled very limited visibility into holistic cell structure and function given their targeted focus on just a few specific mRNAs.
However, thanks to recent advances in computing capacity, improvements in imaging and microscopy technology, and the falling cost of next-generation sequencing, there are now two more efficient and scalable methods for carrying out gene expression analysis: 1) imaging-based methods, and 2) sequencing-based methods.
Imaging-based transcriptomics chemically mark mRNAs to differentiate between the two types before capturing the structure of the cell in-situ via high-resolution microscope imaging technology.
Meanwhile, sequencing-based methods involve extracting mRNAs from tissue while preserving their spatial information via hybridization, and then using NGS technology to profile them.
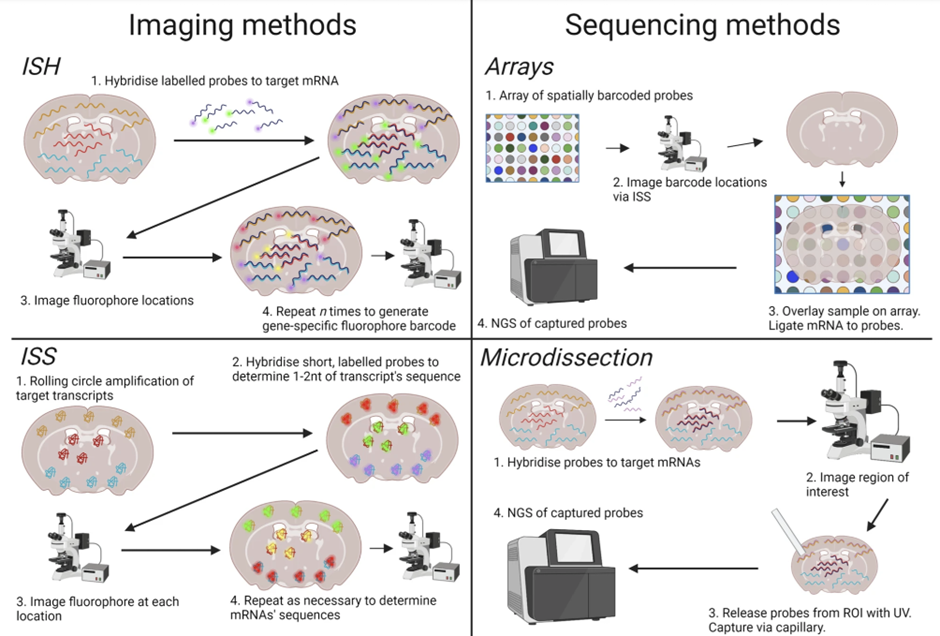
Source: https://genomemedicine.biomedcentral.com/articles/10.1186/s13073-022-01075-1/figures/1
Both approaches use a mixture of chemical techniques and microscopic imaging to capture the arrangement of mRNAs in a cell, and encompass a range of techniques that are typically developed for specific applications or concurrent use.
This is because, due to technological limitations, there is a trade-off between the mRNA capture efficiency of a technique, and the number of genes it is able to profile. For example, ISH detects mRNAs more efficiently than ISS, but ISS then has the benefit of being able to examine larger tissue areas.
Such trade-offs are not specific to the domain of genetic research, but are instead part of a broader AI trend that businesses are seeing emerge as they deploy the technology for more ambitious applications. For instance, many businesses today face the issue of whether to fine-tune one LLM, or utilize multiple SLMs in conjunction, a question which ultimately boils down to a trade-off between accuracy and scalability.
Nevertheless, this trade-off is felt particularly keenly in the field of genetic research, where accuracy is of course a key priority, but the increasingly large amount of data being generated now requires the technology to be highly scalable.
This brings us to the key issue of data management, an area of genetic research which is now predominantly handled by AI.
How AI optimizes genetic data management
Due to the complexity of the procedures described above, both spatial transcriptomics and automated genomic sequencing are highly reliant on AI technology to prepare, optimize, and translate data into different formats at various points along the way.
Primarily, this involves the use of analysis software programs such as Seurat, scanpy, and Giotto. These software programs utilize both supervised and unsupervised forms of machine learning to automatically perform both general and specialized data management functions. These include:
- Data preprocessing/data cleaning
- Data visualization
- āBridge-integrationā a statistical method to integrate experiments measuring different modalities using a separate multi-omic dataset as a molecular ābridgeā
- Data clustering between similar datasets
- Trajectory inference, a computational technique based on specific algorithms to predict the ordering of a group of cells in certain biological processes, thus generating synthetic data which can be compared to real data as a form of quality control or to highlight areas of focus for future research.
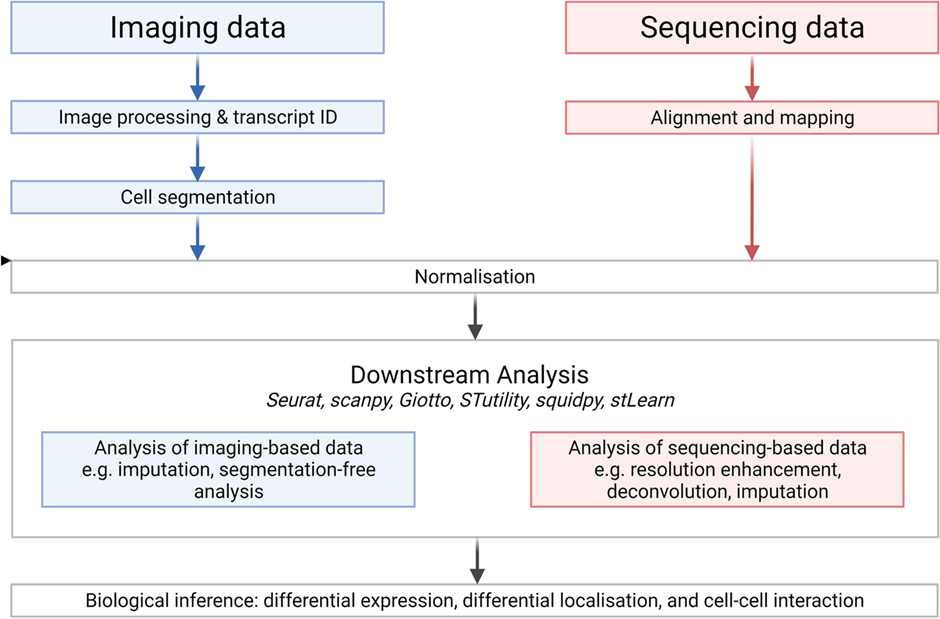
Source: https://genomemedicine.biomedcentral.com/articles/10.1186/s13073-022-01075-1/figures/3
Additionally, once the transcripts have been produced, AI software is used to annotate this data automatically before it is published so as to facilitate real-time public access to the data which can then accelerate research in healthcare and the wider scientific community as a whole.
In the Tree of Life project, for example, genome assemblies are annotated through software pipelines developed by their partners at EMBL-EBI, before being submitted to the publicly accessible European Nucleotide Database.
Similarly, data generated by the HCA Project is free for everyone to access through Web App Data Explorer & Single Cell Transcriptomics, a software programme which also provides optimal accessibility to this data by including a beginner-friendly visual exploration of gene expression at single cell resolution.
But the representation and presentation of this data may also present a further opportunity for optimization through AI, as Nathan Cunningham, Director of Platform solutions at Wellcome Sanger Institute, pointed out at the Press Conference there last week.
Indeed, with chatbots becoming an increasingly popular form of information management, research institutions such as Sanger Wells (which are essentially sitting on a goldmine of high quality data) could see significant gains in terms of publicity and efficiency by investing in a chatbot fine-tuned for genetic research.
Nevertheless, Generative AI workloads are notorious for the amount of compute and processing power they require, which is a key consideration for organizations looking to implement GenAI applications such as chatbots, particularly if they need to be fine-tuned on large and complex datasets such as genomic transcripts.
Challenges of using AI in genetic research
Within the life sciences industry in particular, the utilization of AI can elevate resistance to proposed research projects, or exacerbate existing concerns over the perceived existential threat of the technology.
You only have to look to the origins of evolutionary biology to see this, with even Darwinās ground-breaking Theory of Natural Selection struggling to gain widespread acceptance until relatively recently due to the perceived challenge it posed to the Christian teaching of Creationism.
In fact, the HGP itself was subject to an opposition campaign from the academic community, with several researchers viewing the project as overly ambitious, arguing that it did not have sufficient scientific rigour to justify its expense.
While the use of automation and genomic sequencing technology did in fact enable the completion of the project ahead of time, ultimately bringing down its expenses, the opposition to this project highlights one of the major difficulties of using AI in scientific research: namely, that a lot of AI technology, particularly Generative AI, is still in its infancy and undergoing rapid development.
This can make it difficult to quantify/guarantee the exact gains that the technology will be able to bring to a research project, and potentially detract from the perceived viability of certain research projects.
For example, arguably one of the most ambitious yet hoped for breakthroughs in healthcare is the dawn of personalized medicine. But the dependency of such a reality on still developing AI technology is proving to be a significant bottleneck to its mainstream realisation in healthcare systems.
Furthermore, the methodology of various genetic and medical research projects has raised major ethical concerns in the past. Indeed, the 20th century and early 21st centuries in particular saw many notorious cases of research projects which involved contentious and often downright unethical practices such as stem cell research, animal testing, and inhumane testing on minority groups.
This is a key concern for Haniffa, who argues that a major limitation of the HGP was the lack of diversity in its representation of the human genome.
āWhen the first sequence of the [Human Genome] project was revealed to the world, the genomic sequence came from a group of just four or five individuals, and was essentially a mash up of all their genes. So one of the issues with that project was that because it was limited to just a few individuals, it didnāt represent many different ethnic groups. Within genetic research more generally, it is quite a common phenomenon that at least one ancestry is not represented. With the Human Cell Atlas, we are applying lessons learned from from the original human genome project. For example, because it has global leadership, it is already designed to be a bit more diverse and representative of the global population from the onset.ā ~ Muzlifah Haniffa, Senior Research Fellow at Wellcome Sanger & Professor of Dermatology and Immunology at Newcastle University
With the propagation of societal bias and discriminatory data already proven to be a major risk of AI, its adoption within the field of genetic research could accentuate the risk of genetic research failing to be globally representative and diverse if not managed responsibly. This will become especially important as AI is used to generate synthetic data that may be used to not only calculate the feasibility of genetic research but also act as a quality control measure for real output data.
āMachine learning is essentially a ChatGPT for biology. It can predict, for example, what the structure of a new cell will look like. So by having AI in the lab, you can make predictions for the success of various medications and treatments for particular types of people which you can then measure against the real data collected from the patient. But for me, the big caveat is that it has to be ethical and accurate. What you donāt want to happen is something like that incident where an AI model was used to classify images of human faces but was trained on a biased sample of data, and so ended up propagating an existing bias against certain groups of people. If we want to use AI to benefit humankind, it has got to be well-trained. And this is where somewhere like Sanger Wells comes into play, because you actually have the ability to generate data at scale and to undertake this type of work to the highest level of quality.ā ~ Muzlifah Haniffa, Senior Research Fellow at Wellcome Sanger & Professor of Dermatology and Immunology at Newcastle University
The key role of data centres in powering genetic research
One of the reasons that the Sanger Wells Institute plays such an important role in the responsible deployment of AI in genetic research is, as Haniffa mentioned, its ability to generate data at scale.
This is because the institute is fortunate enough to have its own on-site data centre, which not only facilitates the efficient management of the genomic sequencing machines, but also ensures that the computing capacity of the facility is able to keep pace with the rapid pace of both technological innovation and genetic research.
Currently, the data centre contains approximately:
- 400 racks of IT equipment
- 100 GPUs
- 220Pb of high density storage
- 4MW of power
Ultimately, it was these on-site resources that enabled the Sanger Wells Institute to play such a prominent role in the HGP, carrying out approximately one third of the research.
Today, with the heavy demands that most workloads place on compute and processing power today, the data centre continues to be one of the instituteās biggest assets. Not only does it enable Sanger Wells to continue to play an active role in the publication and management of genomic data through software applications, it also facilitates the efficient management of the genomic sequencing machines which each now output approximately 2-4 terabytes of data per day.
However, the scientific orientation of the Sanger Wells Data Centre does not come without its challenges, as Nick Ewing, Managing Director of Efficiency IT, explains.
āThe work thatās done at Sanger Wellcome is incredible ā the science literally saves lives, whether thatās from Covid, Cholera, Malaria, or otherwise. But what that does mean is that the data centre environment is incredibly complex, because scientists have completely different approaches and needs to most businesses. And this can make the management of the data centre really tough, because youāve got a different type of client to usual, and a different type of infrastructure. So youāve got to deliver different services and accommodate lots of different technology, including AI platforms, high density storage, GPUs, etc.ā ~ Nick Ewing, Managing Director at EfficiencyIT
EfficiencyIT is a company specializing in data centre design and build, and is a UK Elite Partner to leading electronics provider, Schneider Electric. Together, via the deployment of their EcoStruxure IT Expert software, the two companies have helped the Sanger Institute gain greater visibility into the operations of the data centre, thus enabling them to work on ways to optimize its efficiency.
This is particularly important for facilities such as Sanger Wells, where the cost of operational downtime is painfully high given the specialized workloads of the genomic sequencing machines.
āOne of the biggest considerations for Sanger Wells, and other data centres powering genomic research, is the high level of redundancy required by genomic sequencing machines. If a sequencer goes offline, there is a tangible cost of approximately Ā£30,000 – Ā£40,000. If a chemical sequencer goes offline for more than six to eight hours, you would typically lose all the chemicals in that device. And given that there are around 50 of these devices in the data centre, a four to eight hour unprotected downtime incident could cost hundreds of thousands of pounds.ā ~ Nick Ewing, Managing Director at EfficiencyIT
Looking to the future, data centres are set to become even more fundamental to genetic research. This is not only because of the AI processes are now steadily embedded in genetic research, but also because the scale of data being mined from even a single human cell is growing exponentially.
In fact, genomic data is soon set to become the biggest source of data on the planet, with the National Human Genome Research Institute estimating that genomics research could generate up to 40 exabytes of data within the next decade.
This highlights the growing role of data centres in scientific research, which will ultimately bring significant gains to both the life sciences industry and the AI industry. This is because, in Haniffa’s view at least, these two industries have a mutually reinforcing relationship.
āSo the when it comes to whether technology is driving scientific research, or vice-versa, I would say the relationship is a little bit chicken and egg. Thereās always a few people wanting to do something that depends on the technology evolving. But also, once you have the technology, you start to identify what else you can do with it. So I would say its kind of a self-reinforcing or feeding system.ā ~ Muzlifah Haniffa, Senior Research Fellow at Wellcome Sanger & Professor of Dermatology and Immunology at Newcastle University
For many countries, particularly the UK with its historical strength in the life sciences industry, this strongly intertwined relationship can only be a good thing. This is not only because research projects such as the HGP are helping to drive technological innovation, but also because of the investment these projects will attract, as Mark Yeeles, VP at Schneider Electric, points out.
āThe life sciences industry is not only important in terms of what it does for our country and the whole world in terms of the different areas of research it covers, itās also super important from an investment perspective, both in terms of what it contributes to the global economy, and also in terms of the funds it brings to our country, in particular this region.ā ~ Mark Yeeles, VP of Secure Power at Schnieder Electric, UK & Ireland
Nevertheless, as the scale of genetic research increasingly stretches beyond the capacity of purely human cognition, these funds will probably need to reinvested into data centres, equipping them with the capacity they will need to manage specialized AI workloads increasingly required by genetic research, and the vast amounts of data this will generate.