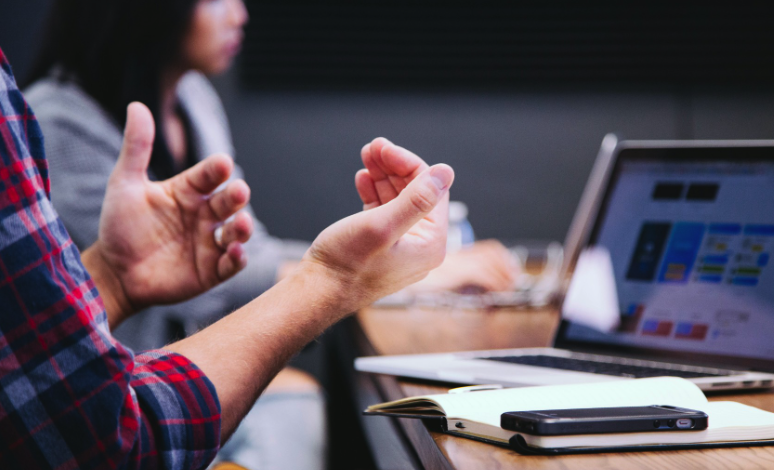
As organizations seek to harness the full potential of data, AI-powered tools are quickly becoming the focus for evolving data management and analysis capabilities. The advent of large-language models (LLMs) like ChatGPT has fired the imagination and renewed conversations around the art of the possible when it comes to AI, but as with many evolving technologies, some misconceptions exist about how it can and should be applied.
For many business use cases, AI tools can deliver immediate value and actionable insights that can be used to improve services and internal processes, often through the automation of complex tasks. Once set up, these automation tools will require only rudimentary intervention and refinement from data scientists. In other use cases, however, the outputs of AI-powered tools, such as machine learning models, cannot be operationalized on their own. This would be entirely irresponsible and would leave organisations exposed to many of the real problems that are inherent in AI-powered technologies, such as erroneous outputs or ‘hallucinations’.
In many industries, AI-powered tools must be used to support decision-making, rather than automate it. Natural language processing (NLP) models enhance data scientists’ ability to treat information, to extract and classify data, and create real-time indicators. This is a secure application of AI, as each use case has humans as the arbiters of what is true and useful in a closed data universe—the universe being constructed from data streams that have been carefully curated for a specific job.
This is why models that deliver outputs that cannot be verified, i.e. black box solutions, are of no use in contexts where confidence in precision requires explainable methodologies behind the models that inform the decisions. But this isn’t to say that black box solutions, such as generative AI and other large language models (LLMs), are not useful in many other business contexts. NLP can be used for prompt engineering, as we see with translation decoder models, and also as summarisation tools for content. In both instances, the results can be easily verified by human subject matter experts and native language speakers.
A fundamental approach
The real value of machine learning and deep learning models is their ability to arrange and analyze vast amounts of unstructured data, such as satellite data or textual data. For each use case, however, data scientists must ensure their models are grounded in material reality, which necessitates adopting a fundamental approach. This means that conventional and industry-standard data is used to corroborate and improve the outputs of models. In the context of estimating crop yields, for example, models must show a strong correlation with official statistics over a long period before they can be operationalized.
For every use case, the fundamentals of analysis that are pertinent to a model’s context must take precedence. If the supply of a commodity is suppressed, for example, we know that its price will rise; and if one of the world’s largest exporters of wheat is invaded and thrust into war, as was the case with Ukraine, the price of wheat will climb rapidly.
Following the invasion of Ukraine, it became crucial to understand the security of supply chains for grain around the world, which was made possible through the analysis of alternative data, such as meteorological data and satellite imagery that helped to estimate crop yields, highlighting the importance of extracting and correlating data from multiple sources. This is where NLP models also allow us to extract salient information about defined use cases from industry and national news sites, social media, and official statistics. These can then be analyzed and formulated into an indicator that correlates with today’s material reality, i.e. a nowcast.
Another example of this is the use of satellite data to analyze pollution levels from steel mills across a certain region. If pollution levels rise and remain consistently high over some time, we can assume that the country of origin is likely to begin large-scale construction or increase steel exports, which correlates with increased GDP. Using other indicators for GDP, that validate the hypothesis, it is possible to create a robust indicator for tracking GDP in real-time.
Importantly, in each of the above use cases, the outputs have not been delivered by artificial intelligence tools but rather informed by them.
Refining intelligence
A data scientist’s work is never done. No model should ever be considered complete, as they are always works in progress. There is always more data, intelligence, and ingenuity that can be applied to refine models and the indicators they inform. By measuring daily and assessing nowcasts against official statistics, data scientists will always be able to get closer to the material reality.
With the evolution of IoT and the natural proliferation of statistical information, there is more data than ever available for the construction and refinement of models. As new data comes online, data science teams will experiment, validate, and deliver increasingly accurate insights. IoT is improving the accuracy and quality of information, enabling us to extract better signals from the noise related to various use cases. Much like weather monitoring stations, that deliver real-time measurements of temperature, wind velocity, humidity, and atmospheric pressure, IoT devices are delivering real-time telemetry data that can be harnessed across industries.
When combined with models that enable sentiment analysis, contextual data extraction, and image and audio reconstruction, the possibilities are endless. For instance, we can use textual analytics models to measure supply and demand in the construction industry by analyzing social media and industry websites. It is also possible to extract text from videos or transform satellite images into an economic time series relating to NO2 emissions, drought conditions, or real estate construction estimates.
Of course, when the insights delivered by these indicators are combined, new and more compelling insights can be delivered through AI-powered tools. In many cases, however, these insights must be the servant and not the master—with human intelligence behind decision-making.