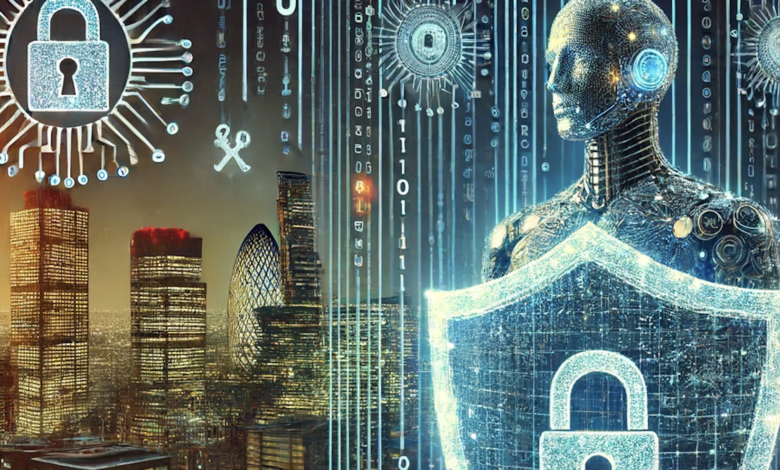
Artificial Intelligence (AI) is transforming data security management, offering exciting advancements alongside new challenges. AI simulates human intelligence, enabling learning, reasoning, and self-correction. This technology spans machine learning, natural language processing, neural networks, and robotics, all working together to analyze vast amounts of data, recognize patterns, and make decisions with minimal human input.
Imagine AI-driven systems scanning network traffic in real-time, spotting anomalies that hint at potential cyber-attacks before they cause harm. It’s like having a vigilant security guard who never sleeps. However, AI also faces challenges, such as data poisoning, where attackers feed malicious data to skew results and biases inherited from training data. Understanding AI’s dual facets—benefits and risks—is crucial for crafting effective security solutions.
Threats from AI in Data Security
While AI offers significant advancements, it also introduces vulnerabilities. Key threats include:
- Data Poisoning: Malicious actors can alter training data, compromising AI models. For example, biased data could skew recruitment decisions. Imagine an AI-driven hiring platform where attackers inject biased data, causing the system to favor or reject certain demographics unfairly (CSO).
- Model Theft: Attackers can steal AI models, gaining access to sensitive information. Cybercriminals might steal an AI model used for credit scoring, manipulating credit approvals fraudulently.
- Automated Attacks: AI can automate sophisticated cyberattacks, such as personalized phishing emails mimicking internal communications.
- Data Bias and Discrimination: AI algorithms trained on biased data can reinforce societal biases, affecting decisions like mortgage approvals.
- Adversarial Attacks: Hackers can subtly alter input data to deceive AI systems, bypassing security measures like spam filters. Imagine hackers slightly altering an image to fool an AI-powered facial recognition system.
Recognizing and mitigating these threats is essential for leveraging AI’s potential securely and ethically. Organizations must adopt comprehensive strategies to safeguard their AI systems and ensure the integrity of their data security measures.
Opportunities for AI in Data Security
When effectively managed, AI can revolutionize how organizations protect their data, offering advanced capabilities that surpass traditional security methods. Key opportunities include:
- Enhanced Threat Detection: AI can evaluate massive volumes of data in real time, identifying and responding to threats much faster than humans. For example, AI systems can detect irregularities in network traffic, flagging potential cyber-attacks before they escalate. Imagine a global enterprise mitigating a cyberattack within minutes thanks to AI’s rapid analysis.
- Automation of Repetitive Security Tasks: AI automates routine security activities, allowing human analysts to focus on more complex threats. This includes tasks like vulnerability scans and security flaw corrections. By handling these repetitive tasks, AI speeds up processes and reduces the risk of human error. An AI system could continuously scan for vulnerabilities in an organization’s software, automatically applying patches as needed.
- Proactive Risk Management: AI’s predictive capabilities enable proactive risk management by identifying potential security vulnerabilities before they can be exploited. AI can continuously monitor and analyze system logs to predict and prevent security breaches. Consider a retail company using AI to predict and prevent data breaches during high-traffic sales periods.
Example: Financial Institution Securing Online Banking
Imagine a financial institution leveraging AI to secure its online banking platform. The AI system continuously monitors login attempts and transaction patterns, identifying anomalies that could indicate potential fraud. With advancements in multi-modal capabilities, this verification might soon include biometric methods such as voice and facial recognition, enhancing security further by adding another layer of protection against fraudulent activities.
Looking to the future, AI could enable even more advanced security measures for online banking. For instance, AI could analyze the unique behavioral patterns of each user, such as typing speed, mouse movements, and navigation habits, to create a digital fingerprint. The system could automatically initiate a security protocol if a login attempt deviates significantly from these patterns. Additionally, AI could predict potential fraud by analyzing global financial trends and emerging threats, allowing banks to adjust their security measures preemptively.
Navigating the AI Landscape
Organizations must balance innovation with responsibility, ensuring their AI deployments are effective and ethical. Key considerations for integrating AI into security strategies include:
Carefully Analyze Use Cases
Clearly define the problem you aim to solve with AI and ensure it aligns well with the technology’s capabilities. Thorough analysis helps identify whether AI is the appropriate solution and how it can be most effectively applied.
Example: A healthcare provider uses AI to analyze patient data for early disease detection. Before implementation, they assess whether AI can improve diagnostic accuracy compared to traditional methods and ensure it integrates seamlessly with existing medical records systems. In the future, AI could be used to predict outbreaks of diseases by analyzing data from wearable devices and social media trends, allowing healthcare providers to take preventive measures in real time.
Mitigate Potential Risks
Implement robust data governance standards and continuously monitor AI systems for biases and vulnerabilities. Explaining AI approaches can enhance transparency, allowing stakeholders to understand and trust AI decisions.
Example: An online retailer implements AI to recommend products to customers. They regularly audit the AI system for biases to ensure diverse product recommendations and use explainable AI to clarify how recommendations are made to customers. Looking ahead, AI could personalize shopping experiences by analyzing customer emotions through facial recognition and voice tone during interactions, offering tailored recommendations that adapt in real time to customer moods.
Consider the Ethical Implications
Address the ethical concerns associated with AI, such as fairness, transparency, and accountability. Ethical AI practices ensure that AI systems are used responsibly and do not inadvertently cause harm (Egnyte)
Example: A financial institution uses AI for loan approvals, ensuring the system is transparent and accountable. They established an ethics committee to oversee AI practices and address biases that could unfairly affect loan applicants. Future AI developments could involve dynamic ethical frameworks that adapt to evolving societal values, continuously updating AI decision-making processes to reflect current ethical standards.
Technical Controls for AI in Data Security
Implementing AI in data security requires a balanced approach integrating technical rigor with ethical considerations. Critical components and best practices for deploying AI-driven data security measures include:
Data Access Control
- Implement Granular Access Controls: Use least privilege principles to ensure users have the minimum necessary access to perform their duties.
- Multi-Factor Authentication (MFA): Apply MFA across all access points to enhance security.
- Monitor and Audit User Access: Regularly review user access rights to detect and mitigate unauthorized access.
Example: An organization implementing granular access controls ensures that only finance team members can access financial records, reducing the risk of data breaches. They also implement MFA, requiring team members to verify their identity with a second factor, such as a mobile app, before accessing sensitive data. In the future, AI could enable adaptive access controls that dynamically adjust user permissions based on real-time assessments of user behavior and risk levels (Nist ).
Data Governance
- Data Classification and Labeling: Establish clear processes for classifying and labeling data based on sensitivity levels.
- Data Minimization: Implement procedures to ensure only necessary data is retained, reducing the risk of exposure.
- Data Encryption: Encrypt data both at rest and in transit to protect it from unauthorized access.
- Regular Backups: Maintain regular backups of critical data to ensure recovery in case of data loss or breach.
Example: A healthcare provider classifies patient data as highly sensitive and ensures it is encrypted and regularly backed up to prevent data loss and unauthorized access. They also implement data minimization practices, retaining only essential patient information to reduce exposure risks. Future advancements could involve AI-driven encryption algorithms that automatically adapt to emerging threats, ensuring data remains secure against the latest cyberattack techniques.
Model Security
- Secure Development Processes: Ensure security is integrated throughout the AI model development lifecycle.
- Monitor and Update Models: Regularly check for model drift and vulnerabilities, and update models as necessary.
- Explainable AI (XAI): Utilize XAI techniques to provide transparency in model decisions, fostering trust and accountability.
- Data Poisoning Detection and Prevention: Implement techniques to detect and prevent data poisoning attacks that could compromise model integrity.
Example: A tech company develops an AI model for fraud detection, continuously monitoring it for performance issues and retraining it with updated data to maintain its accuracy. They also use explainable AI to ensure transparency in how the model flags transactions as potentially fraudulent, which helps in gaining trust from stakeholders and customers. In the future, AI models could be self-healing, automatically detecting and correcting vulnerabilities or biases without human intervention.
Network Security
- Network Segmentation: Isolate AI systems from sensitive data through effective network segmentation.
- Intrusion Detection/Prevention Systems (IDS/IPS): Deploy IDS/IPS to monitor and defend against network threats.
- Regular Updates: Keep network security software and firmware up to date to protect against vulnerabilities.
Example: A retail company segments its network, isolating the AI-powered customer recommendation system from the payment processing system to enhance security. They also deploy IDS/IPS to detect and prevent unauthorized access attempts and ensure all network security software is regularly updated to patch vulnerabilities. Future AI systems could leverage quantum computing to detect and neutralize sophisticated cyber threats instantaneously, providing unprecedented levels of security.
Ethical Considerations
Ethical use of AI is critical to improve trust. Consider:
Bias Mitigation
- Check Training Data: Regularly review training data for biases and imbalances.
- Use Diverse Datasets: Ensure datasets represent varied demographics to reflect diverse populations accurately.
- Monitor and Address Biases: Continuously monitor AI model outputs for biases and address them promptly.
Example: A recruitment platform uses diverse datasets to train its AI, ensuring fair and unbiased candidate selection processes. They regularly review the training data and adjust the model to correct any detected biases, promoting equal opportunity for all applicants. In the future, AI could autonomously detect and mitigate bias in real-time, ensuring consistently fair outcomes.
Transparency and Explainability
- Utilize XAI Approaches: Implement explainable AI methods to make AI decision-making processes clearer.
- Communicate Limitations: Explicitly communicate AI models’ limitations and potential biases.
- Human Oversight: Establish human oversight and intervention processes in AI decisions.
Example: A financial services firm uses explainable AI to make credit scoring decisions transparent, allowing loan officers to understand and explain the rationale behind AI-generated scores to applicants. They also communicate any limitations of the AI model to ensure applicants understand the factors influencing their credit scores. Future AI systems might include interactive explainability features, where stakeholders can query the AI to understand decision logic more deeply.
Tools to Enhance AI and Data Security
To implement these technical and ethical controls effectively, several tools and platforms can help organizations enhance their AI and data security capabilities:
- Microsoft Security Copilot: An AI-powered security tool that helps organizations identify and respond to threats more quickly by integrating with existing security systems and providing real-time insights.
- IBM Watson for Cyber Security: Leverages AI to analyze vast amounts of data and identify potential security threats, providing actionable insights to security teams.
- Darktrace: Uses AI to detect and respond to cyber threats in real time, learning normal patterns of behavior to identify and mitigate anomalies.
- Splunk: A data analytics platform that uses machine learning to monitor and analyze security data, helping organizations detect and respond to threats more effectively.
- Symantec Endpoint Protection: Combines AI and machine learning to provide advanced threat protection for endpoints, identifying and mitigating potential security risks.
Conclusion
Navigating the AI landscape, particularly in the realm of data security, requires a careful and thoughtful approach. AI presents both tremendous opportunities for enhancing security measures and significant risks that must be managed diligently. To fully secure the benefits of AI, organizations must implement robust technological controls such as data access control, data governance, model security, and network security.
However, technology alone is not sufficient. Ethical considerations are equally crucial. Organizations must proactively address biases in training data, ensure AI decisions are transparent and explainable, and establish clear accountability frameworks. Continuous monitoring, regular adaptation, and strict adherence to ethical standards are essential for harnessing AI’s full potential while safeguarding sensitive data (Checkpoint).