In the rapidly evolving field of data science and artificial intelligence, ethical considerations have become paramount. This article will explore the ethical challenges that data scientists and organizations face when working with large datasets, machine learning algorithms, and AI technologies. It will delve into topics such as bias in AI, data privacy, transparency, and accountability.
Bias in AI: The Unseen Culprit
Bias in AI is a critical issue that demands diligent attention within the data science community. It’s of paramount importance to address bias in AI algorithms due to its far-reaching consequences. Biased AI systems can perpetuate and even exacerbate societal prejudices, leading to unfair and discriminatory outcomes in areas such as hiring, lending, and criminal justice. By examining real-world examples of biased AI systems, such as algorithms that discriminate against certain racial or gender groups in facial recognition technology or sentencing decisions, we can vividly illustrate the tangible harm that biased AI can cause.
Data scientists play a pivotal role in mitigating bias in AI models. They must first recognize that bias can manifest in various forms, including selection bias in training data, algorithmic bias, and even interpretational bias. To combat bias, data scientists can employ techniques like fairness-aware machine learning, which involves adjusting algorithms to ensure equitable outcomes for different demographic groups. Additionally, conducting comprehensive audits of training data for potential biases and continuously monitoring and re-evaluating models in real-world contexts are crucial steps.
Ultimately, addressing bias in AI is not just a technical challenge; it’s a moral and ethical imperative. It requires a concerted effort from data scientists, policymakers, and organizations to build AI systems that are not only accurate and efficient but also fair and unbiased, fostering a more just and equitable future.
Data Privacy: Guardians of Digital Sanctity

Data privacy is an ethical cornerstone in the realm of data science, carrying profound implications for individuals and society as a whole. At its core, data privacy concerns the responsible handling of personal information. It’s vital to recognize the ethical dimensions of collecting and utilizing personal data, as it can profoundly impact an individual’s autonomy, security, and dignity.
One paramount ethical consideration is obtaining informed consent. This means that individuals should be fully aware of how their data will be used and for what purposes, and they should have the option to opt in or out without coercion. Moreover, data anonymization, the process of removing personally identifiable information from datasets, is instrumental in protecting privacy while still enabling valuable data analysis. It’s not only ethically responsible but also legally mandated in many jurisdictions, including under the General Data Protection Regulation (GDPR) in Europe.
Compliance with data protection regulations like GDPR is not just a legal requirement but a moral obligation. These regulations provide a framework to safeguard individuals’ privacy rights, encouraging organizations to adopt transparent data practices, implement robust security measures, and be accountable for any data breaches.
Transparency & Explainability: Decoding AI’s Secrets
Transparency and explainability are pivotal aspects of responsible AI development that foster trust and accountability within the field. In an age where AI systems are becoming increasingly integrated into critical decision-making processes, understanding why and how AI makes decisions is paramount.
Transparency entails making the AI decision-making process clear and comprehensible to stakeholders, including end-users, regulators, and the public. When AI algorithms are transparent, individuals can better understand how and why certain decisions are reached. This understanding enhances trust, as users can verify the fairness and equity of AI-driven outcomes.
Explainability, on the other hand, focuses on providing meaningful justifications for AI decisions. It enables stakeholders to delve deeper into the decision-making process, offering insights into which features or data points influenced a particular outcome. Explainable AI models empower users to challenge and correct biased or unfair decisions, making AI systems more accountable.
Ultimately, transparent and explainable AI models not only bolster trust among users but also enable regulators to enforce ethical and legal standards effectively. They facilitate the identification and rectification of biases, errors, or unintended consequences, contributing to the responsible and ethical deployment of AI technology across various domains.
Accountability Unveiled
Accountability in AI is a shared responsibility between data scientists and organizations, critical for ensuring the ethical use of AI technologies. Data scientists play a central role in:
- Data Selection and Preprocessing: They must ensure that training data is representative and devoid of biases that might lead to unfair outcomes.
- Algorithm Design: Data scientists should create models that are transparent, fair, and robust, rigorously testing them for potential biases.
- Monitoring: Continuous real-world monitoring of AI systems is crucial to detect and rectify biases or unforeseen consequences promptly.
Notable case studies, such as Amazon’s biased recruiting tool, the ProPublica-revealed COMPAS algorithm bias, and Facebook’s ad targeting issues, highlight the repercussions organizations face when failing to uphold ethical AI standards. These cases underscore the critical need for accountability, transparency, and ongoing monitoring to prevent harm and ensure equitable AI benefits for society. Ethical AI is not an option but an ethical imperative in the AI era, and both data scientists and organizations must uphold it.
Ethical Frameworks
Ethical frameworks serve as essential roadmaps for data scientists embarking on AI projects, guiding them in making morally sound decisions throughout the development process. Among these frameworks, the AI Ethics Guidelines established by the Institute of Electrical and Electronics Engineers (IEEE) and the Association for Computing Machinery (ACM) stand out as influential and widely respected.
The IEEE’s AI Ethics Guidelines offer a comprehensive and principled approach to ethical AI development. They stress key principles such as transparency, ensuring that the inner workings of AI systems are understandable and accountable. Privacy protection is paramount, advocating for the responsible handling of personal data. The guidelines also prioritize fairness, encouraging data scientists to combat biases and discrimination within AI algorithms. Moreover, they emphasize robustness, pushing for AI systems that can withstand errors and adversarial attacks.
In contrast, ACM’s Code of Ethics and Professional Conduct, while not AI-specific, provides enduring ethical principles relevant to AI practitioners. These include general responsibility, emphasizing integrity and privacy; avoiding harm, prioritizing well-being in AI design; professionalism, encouraging adherence to ethical standards; and fairness and access, promoting equitable AI systems.
In addition to these frameworks, I invite readers to explore Stylianos Kampakis’ interviews and podcasts on this crucial topic to gain further insights from experts and thought leaders in the field.
Future Trends: Charting Ethical Waters
The future of ethics in AI is a dynamic landscape marked by both exciting advancements and profound ethical challenges. As AI continues to advance, emerging ethical issues demand our attention and consideration.
One pressing concern is the proliferation of deepfakes and AI-generated content. These technologies raise concerns about misinformation, privacy invasion, and the erosion of trust in visual and auditory media. Data scientists and technologists will need to develop robust methods for detecting and mitigating the impact of deepfakes while respecting legitimate creative uses of AI-generated content.
Autonomous systems, including self-driving cars and drones, pose ethical dilemmas related to safety, accountability, and decision-making in critical situations. The development of AI algorithms capable of making split-second, life-or-death decisions requires careful ethical guidelines to ensure public safety and legal responsibility.
The field of AI ethics is likely to evolve with increased collaboration between technologists, ethicists, policymakers, and the public. Ethical considerations may become more ingrained in AI development, with greater emphasis on transparency, fairness, and accountability. As AI systems become more integrated into our daily lives, the ethical dimension will remain at the forefront of discussions, necessitating a proactive approach to address emerging challenges responsibly and ethically.
Ethical Case Studies: Lessons from the Field
Examining real-world case studies of companies and projects that have either successfully navigated ethical challenges or faced backlash due to ethical lapses offers valuable insights into the practical application of AI ethics.
One illustrative case is Google’s Project Maven, a collaboration between the tech giant and the U.S. Department of Defense. Project Maven aimed to use AI to analyze drone footage. However, it faced intense backlash from Google employees and the broader public who raised concerns about the technology’s use in potentially harmful military applications. In response, Google decided not to renew its contract with the Pentagon, demonstrating the importance of employee activism and ethical considerations in shaping corporate decisions.
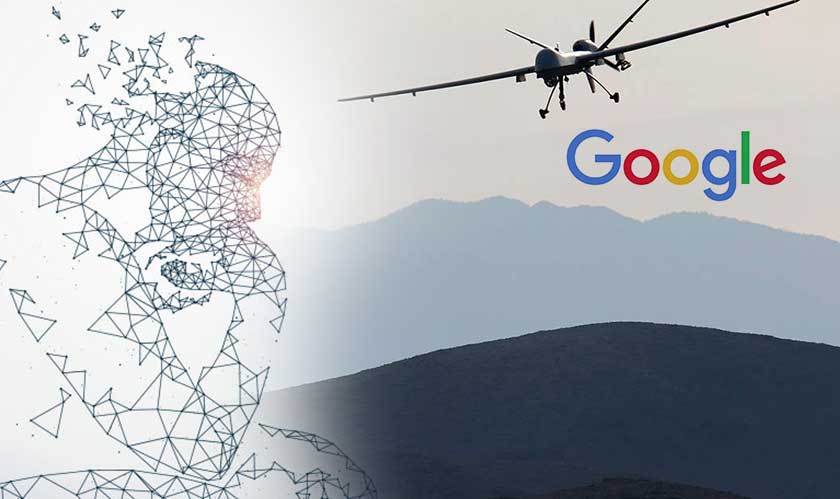
Conversely, IBM’s Project Debater provides an example of a successful ethical AI project. It developed an AI system capable of engaging in persuasive debates on complex topics. IBM ensured that the AI was transparent about its sources of information, actively highlighting potential biases. This transparency and the careful handling of sensitive topics exemplify ethical AI development.
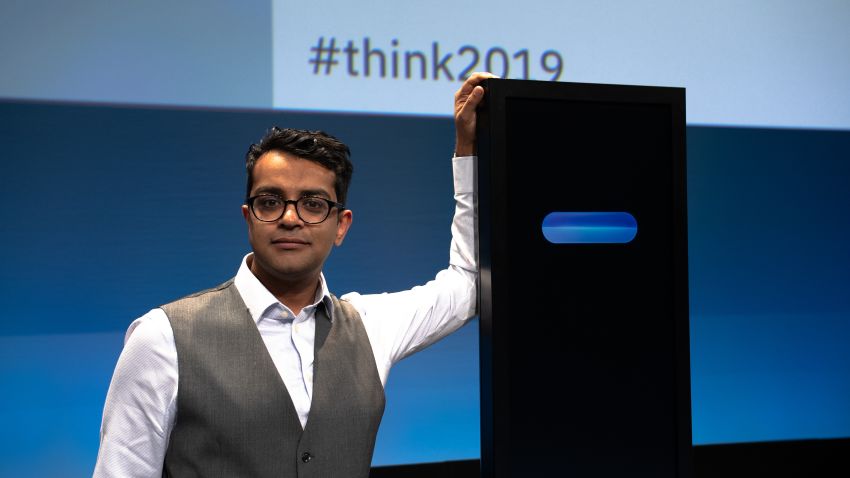
These case studies underscore the significance of ethical considerations in AI projects. They highlight the potential consequences of overlooking ethics or, conversely, the benefits of proactively addressing ethical challenges. They serve as valuable lessons for data scientists, organizations, and policymakers navigating the intricate ethical landscape of AI.
Best Practices Crafting Ethical AI
To integrate ethical considerations effectively into AI development processes, data scientists and organizations can adopt several best practices. Firstly, establishing clear ethical guidelines and principles specific to AI projects is essential. This involves conducting regular ethics assessments throughout the project lifecycle, identifying potential biases, and mitigating them.
Secondly, fostering interdisciplinary collaboration among data scientists, ethicists, and domain experts encourages a well-rounded approach to ethical AI. It’s crucial to ensure that diverse perspectives are considered when making ethical decisions.
Moreover, data scientists should prioritize transparency by documenting their algorithms and data sources comprehensively. Explainability tools can help in making AI decisions more understandable to non-experts.
Additionally, organizations must invest in ongoing ethics training, encourage learning from the Artificial Intelligence course for their AI teams and promote a culture of ethical awareness. Lastly, engaging with stakeholders, including end-users and affected communities, ensures that AI systems align with societal values and needs.
By following these best practices, data scientists and organizations can navigate the ethical complexities of AI development, fostering responsible and equitable AI systems that benefit society.
Balla