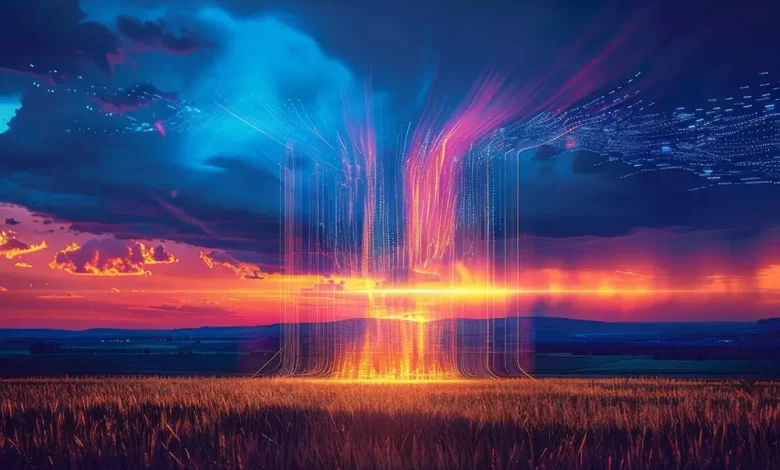
Ever orchestrated a vacation meticulously around blissful weather, only to find yourself ensnared in a downpour despite the forecast’s sunny promises? The conundrum of forecasting weatherāfraught with its inherent capriciousnessāextends beyond mere inconvenience. It plays a pivotal role in ensuring safety, whether it’s navigating through torrential rain, steering clear of wildfire-prone areas, or girding oneself against tempestuous winds and deluges. Envision a reality where weather predictions are as precise and tailored as the GPS app on your phone, making the morning quandary of whether to grab an umbrella or map out a safe route a thing of the past. This vision is swiftly becoming our present, thanks to the groundbreaking infusion of artificial intelligence (AI) and machine learning (ML) into meteorology, equipping scientists to better unravel and master the enigma of atmospheric phenomena.
AI’s prodigious capacity to wade through vast oceans of data and discern intricate patterns ushers in a new epoch in the science of weather prediction. Leading tech behemoths like Google Research, Google DeepMind, and Huawei have recently showcased the prowess of ML-driven models in eclipsing traditional gold-standard forecasting methodologies, all while consuming a mere fraction of the computational resources. From offering farmers bespoke agricultural insights to forecasting the trajectories of devastating cyclones, AI and ML are reshaping our engagement with and comprehension of meteorological phenomena. This discourse delves into the transformative influence of AI and ML on weather forecasting, examining the foundational science, the groundbreaking advancements and practical applications they herald, as well as the obstacles that must be navigated in our pursuit to forecast the unfathomable.
Figure 1. The evolution of weather forecasting techniques over time.
The Conventional Method of Weather Forecasting
For many years, meteorologists have leaned on intricate physical equations of the atmosphere to craft weather forecasts. Theoretically, if meteorologists possess an accurate grasp of present atmospheric conditionsāsuch as wind patterns, atmospheric pressure, and precipitationāthey can employ these variables to construct a comprehensive weather forecast. However, these equations frequently fall short, struggling to encapsulate the complex and ever-evolving nature of Earth’s weather systems. Even minor inaccuracies in measuring current weather conditions or errors in calculations can cascade into significant forecasting mistakes.
Despite these formidable challenges, scientists have made notable strides in enhancing these traditional physics-based models by fine-tuning equations and minimizing errors. Nevertheless, these advancements come with substantial costs, necessitating expensive computational infrastructure and specialized expertise. Additionally, these models falter in regions where precise equations and robust numerical methods are lacking, such as in predicting heatwaves or short-term rainfall.
A further significant challenge with these models is reconciling the dichotomy between different temporal scales. Typically, the public is interested in short-term weather forecastsāranging from several hours to a few days aheadāfor day-to-day planning. Conversely, environmentalists and scientists often focus on climate trends that unfold over decades. To address these diverse timescales effectively, different foundational equations or custom-designed algorithms are needed, along with considerable computational resources.
Learning from the Sky: AI Enters the Forecasting Arena
AI models, particularly machine learning (ML), represent a paradigm where computers gain the ability to learn from data and enhance their performance over time, much like humans do through experience. Fundamentally, ML involves supplying a computer system with vast amounts of data, ranging from images to numerical figures to textual information. The system then seeks out patterns and underlying rules within this data, often guided by a function that evaluates how closely its current predictions match the actual outcomes (Figure 2). By employing ML-based weather models, trained on extensive repositories of meteorological data, we can leverage the wealth of historical weather patterns to forecast future conditions with heightened precision and efficiency. Unlike traditional models that merely utilize data, these AI systems actively learn from it, uncovering subtleties and trends that may elude human forecasters or conventional methods.
Figure 2. Illustration of the workflow of ML-based weather forecasting models as described in the main text
The Fusion of AI and Traditional Methods in Meteorology
The infusion of AI and ML into meteorology heralds a transformative era. Recent research from Google DeepMind and Huawei has underscored the remarkable capabilities of AI-based models, particularly in handling intricate phenomena such as long-term climate forecasts and extreme weather eventsāareas where traditional forecasting methods often falter. Just recently, Google Research and Google DeepMind unveiled a cutting-edge hybrid model that synergizes the robustness of conventional physics-based approaches with the adaptive learning abilities of ML.
This hybrid model is comprised of two primary components. The first, known as the “dynamical core,” functions similarly to traditional methods. It is anchored in a framework of physics-based equations and divides the Earth’s surface into a vast 3D grid, calculating variables like wind, temperature, and humidity at each grid point. This setup provides a foundational understanding of the atmospheric interactions across different regions.
The second component, termed the “learned physics module,” acts as an extension of the dynamical core. It addresses more nuanced meteorological aspects that the core alone might not fully capture, such as cloud formation dynamics, precipitation patterns, and the interaction of sunlight with the Earth’s surface. This module employs ML models to assimilate current weather data and project potential changes, thereby refining the initial forecasts made by the dynamical core.
In essence, this hybrid model operates through an iterative process. It begins by assessing the current atmospheric conditions, then uses the dynamical core to generate preliminary forecasts. The learned physics module subsequently fine-tunes these forecasts by incorporating finer details. This cycle repeats, progressively enhancing the model’s accuracy and extending its predictive capability to the desired future period.
Figure 3. An illustrated comparison between traditional vs ML-based models.
Improving the Effectiveness and the Reliability of Weather Prediction
Standard numerical methods by and large us the extensive time and computational power to provide a representation of physical features of weathers systems. In contrast, the hybrid model optimizes this process by leveraging its AI-driven learned physics module, streamlining the overall workflow and significantly enhancing efficiency (Figure 3). As a result, this model not only excels in generating accurate short-term weather forecasts but also provides consistent and dependable predictions over longer periods. It effectively covers a spectrum ranging from immediate forecasts spanning 1 to 15 days, to more extended climate projections that can extend over a decade (Figure 4).
Figure 4. Prediction of the traditional (red) and AI-driven (green) forecasting models compared with the ground truth (black), in terms of cyclone tracking.
Outlook
Admittedly, AI has advanced a lot in the field of weather prediction, but there are still a number of issues that should be solved. One of them is the problem of the stability of the AI driven predictions, especially for āblack swanā events such as highly unlikely, unpredictable but severe meteorological events. Moreover, the application of such sophisticated forecasts in organizing timely and efficient public announcements is still another area of growth. However there are more opportunities in enhancing future implementation. As there are more new technologies in AI being introduced and with large volumes of data being produced, more accurate, high definition and longer range forecast becomes a realistic possibility.
In grazing through the dichotomy of the modern world, climate change and all its ramifications make AI weather prediction a phenomenon that is not just an innovation, but a necessity. The integration of AI with more conventional methods is gradually erasing the mystification of weather forecasting and painting a picture of the future in which society is capable of predicting and proactively planning for the climate that defines our existence.
FAQs on AI’s Meteorological Revolution
- What is AI in weather prediction?
AI in weather prediction can therefore be defined as the process of applying artificial intelligence, and specific branches of machine learning, in order to analyze large amounts of meteorological data. Through such approaches, weather prediction is enhanced through machine learning to present efficient outcomes compared to normal means.
- How does AI enhance weather forecasting accuracy?
AI improves the precision of weather forecasts by analyzing data collected from satellites, stations as well as records collected in the past. This data can in fact be understood by various machine learning algorithms; allowing for very accurate forecasts regarding the specific climate conditions that are expected to occur in future.
- What are the key benefits of using AI in weather prediction?
- Accuracy: AI can process extensive information quickly, resulting in better weather predictions.
- Speed: AI algorithms analyze data faster than traditional methods, providing real-time forecasts.
- Cost-Effectiveness: Businesses can access real-time weather information without expensive tracking equipment.
- Customization: Weather updates can be generalized for certain purposes these may include agricultural, aviation and many more.
- What role do weather forecast APIs play in AI-based weather prediction?
A weather forecast API provides developers with real-time weather data that can be integrated into various applications. This allows users to access current weather conditions and predictions without needing to interpret complex meteorological information.
- How do traditional weather forecasting methods differ from AI-based models?
In the current era of computation and technology, the conventional methodologies involve the use of physical equations and mathematical models for the description and portrayal of the characteristics of weather, which generally demands log computation power and skills. AI, on the other hand, learns from the previous data inputs and so, can gain and enhance the efficiency of its predictions alongside the minimal need for computational resources.
- What are the applications of AI in weather prediction?
- Agriculture: Fulfills the role of weather prognosis so that the farmers can be able to arrange for planting as well as harvesting.
- Transportation: Assists the airlines and the shipping companies in their planning regarding the place to avoid the occurrence of bad weather.
- Disaster Management: Climatological measures for natural calamaties such as hurricanes and floods to prevent their occurrence.
- Energy Management: Develops scenarios of further weather variations which influences the generation and utilization of energy.
- Tourism: Informs the tourists about weather to help in planning.
- What challenges exist in using AI for weather prediction?
- Data Quality: This means that AI forecasted results are valid to the accuracy of the data fed into it. While using the historical data for forecasting, it is noteworthy that wrong or incomplete information may result in creating false forecasts.
- Complexity: Generally, there are many factors that affect weather; therefore, the prediction of this factor is difficult.
- Dependence on Technology: Weather prediction through AI comes with benefits of efficiency, cost and time but it heavily depends on technology and facilities and these are set back in some areas.
- How do AI-based hybrid models improve weather forecasting?
The hybrid models adopt the conventional methods that are focused on the concepts of physical sciences and integrate these features with the learning algorithms of AI. These models rely on the ādynamical coreā for the initial forecast and the ālearned physics moduleā to amplify productivity and precision.
- What advancements can we expect in AI weather prediction in the future?
- Improved Accuracy: Improvements in data handling and algorithms in play means that AI will give far more accurate weather predictions.
- Integration with IoT: In conjunction with IoT, there will be supplementary information; thus, AI will have better predictive accuracy.
- User-Friendly Applications: More weather forecasts will be linked with API to more friendly applications for individual use and commercial use.
- Global Reach: AI weather prediction will be provided to areas that have poor equipment to predict the weather..
- What is the future outlook for AI in meteorology?
The future of AI in meteorology looks promising, with continued advancements leading to more accurate, high-resolution, and extended weather forecasts. As AI technology evolves and more data becomes available, we can expect even greater precision in weather predictions, benefiting various sectors and enhancing our ability to adapt to changing climatic conditions.
Balla