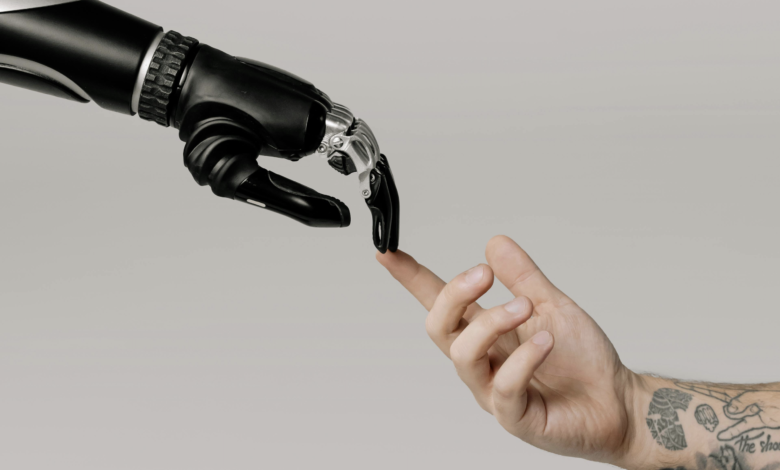
What are AI agents? AI agents are autonomous, intelligent, and task-oriented programs that act as “workers” to achieve a set goal. They are aware of their environment, can learn, use tools, and can be orchestrated to work with other agents to perform simple or complex tasks.
Studies have found that globally, 95% of companies already use AI and 99% expect to in the future (MIT Technology Review Insights). However, few organisations have graduated beyond pilot projects: 76% have deployed AI in just one to three use cases (MIT). Many organisations are finally deploying their own AI solutions, but on average, only 1 in 4 GenAI projects make it past the pilot stage to being fully operational (Lucidworks).
Going all in on one AI model and trying to craft and deploy solutions, with an ever-changing technology landscape is risky. One key challenge with working directly with GenAI models is finding a way to reduce hallucinations and provide more consistently meaningful responses. Choosing the right model for every scenario required requires a lot of trial and error. There is another way. Agents can specialise in particular functions and each use relevant AI or ML models to accomplish small goals. They can help bypass unpredictable usage and infrastructure costs associated with LLM usage.
By leveraging agents, orgs can hugely simplify and optimise everyday processes – an easy way to innovate on the fly without making a massive commitment. It’s like having tireless and talented interns working for you non-stop.
One example is the use of a finance AI agent, FinTalk, which allows users to ask financial questions in natural language. The agent can pull structured or unstructured data from financial databases, ERP, CRM or any system required, interpret the meaning of the request, analyse the results, and present the answer in a meaningful way. You get your answer in real time without needing any system expertise and you can keep your data safely in-house. Users can focus on analysis and interpretation of the results rather than on how to get the relevant data.
Imagine Maria, an operations manager, wants to know the top 10 suppliers with the highest spend in the last 3 years. She can simply type this request into an input box and can quickly identify ways of expanding her business.
How they work: LLMs, integration and no-code
Integration is the key to connecting and maintaining critical data pipelines between applications and systems, especially in a digitally driven world. Using an iPaaS (intelligent integration as a service) platform is the most effective way to manage integrations (via API or low/no code connectors) because it offers a way to easily create the connections using embedded AI agents, and then centrally govern and control them. This is an essential part of creating a data flywheel to feed AI. Integration and no-code development capabilities allow for an autonomous system to understand and generate business context from the live data coursing through your organisations’ data pipelines, learn and recall actions, interact with external resources, and create and execute effective actions to automate business tasks.
LLMs are the brains behind GenAI solutions but to reduce hallucinations businesses need to leverage their own business context. Getting the right data at the right time to the models without allowing your data to be used to train public models is where you need help from central integration frameworks.

Imagine it like a skilled robot in a factory – the robot is equipped with sensors including a camera, pressure sensors, and temperature gauges, which allow the robot to observe the factory environment such as the status of the assembly line or the availability of resources. The robot has a control center much like a brain that processes the information collected by the sensors, using in-built instructions, like rules, and even learning from past experiences to decide on the next steps. In a factory setting, this stage could be recognising a defective product on the assembly line. The robot then takes action – removing that faulty object from the factory line. Each time these robots are not just performing tasks, but learning, using their experience to make better decisions next time. And finally, we can’t forget teamwork – these robots don’t always work alone, dividing and conquering tasks to improve efficiency and performance.
Why AI agents are needed
This is not simply a quest to make everyone’s lives easier. Beyond the obvious benefits of speed and ease, AI agents help you keep pace with the new AI-driven world.
● Tracking data and classifying data fields: makes it easier for companies to know what treasure trove of data they have and where it is located. Classification also means sensitive data can be located and protected.
● Reduce the complexity of integrating systems and applications: autonomous design and build of integrations make connecting your data, systems, and people simple and incredibly fast.
● Transparency: AI agents can help you stay abreast of the growing number of integrations. AI-driven documentation of new and existing integrations brings greater transparency – giving you far more oversight and control when managing your digital connections.
How to develop and integrate AI agents
At least for now, GenAI comes hand-in-hand with questions about trust. Fears of hallucinations, compromised data, and otherwise rogue AI. Enterprises developing AI agents can focus on:
● Transparency and explainability: AI agents should provide clear and understandable rationales for their actions, ensuring users can trust their recommendations.
● Data management and security: Robust protocols for data privacy and security are critical, including stringent access controls and encryption to protect sensitive information.
● Data hygiene: AI agent outputs depend on the quality and availability of relevant data and information that go in. The AI layer needs well-organised, accurate, and complete systems beneath.
Back to Maria. What if the data retrieval process is mishandled and sensitive financial data is accessed? What if the agent’s analysis is based on data that isn’t synched, up to date, or validated? If these things happen, at best, the report would be of little use and, at worst, a trigger for further poor decision-making, financial losses, and declines in client confidence or even legal action. Having a trusted and secure integration platform allows you to build the confidence to trust the AI-generated outcome and reap the benefits of working smarter.
Stay strategic
There are lots of benefits to be had and businesses need to keep up to stay competitive, but, as with all IT transformations, don’t rush into it without careful thought and planning. Setting clear goals, conducting thorough cost-benefit analysis, and choosing strategic timing are the only ways to guarantee value:
● Clarity of goals: Start by identifying the specific problems you aim to solve. Are you looking to automate repetitive tasks, enhance customer service, or gain deeper insights from your data? Precise objectives will help select the right AI solutions and set realistic expectations.
● Cost-benefit analysis: Assess the investment required against the potential benefits and ensure it matches up with current priorities. This involves not just the initial cost of implementation but also ongoing expenses such as maintenance, updates, and training.
● Timing: Avoid the rush to adopt AI without a solid understanding of its relevance and timing for your specific business needs. Do you have the infrastructure, data quality, and talent needed? A phased approach, starting with pilot projects and gradually scaling up, can help minimise risks.
While organisations focus on developing agents of their own, third-party agents are also well worth exploring, but the first step is to make sure your data is in order. Systems already running across your data network have the level of oversight and integration for more immediate and powerful value add. Get yourself a good partner network to help.
AI agents are democratising AI services for all enterprises and the way employees leverage technology. The AI agent revolution is here. Are you ready for it?