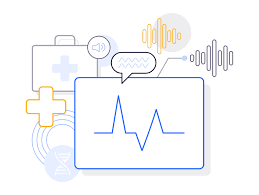
Developments in AI have undoubtedly changed the way the world works – from autonomous vehicles to chatbots to speech recognition technology. The recent eruption of generative AI and AI-powered chatbots has resulted in a frenzied hype in which everybody wants a piece of the pie. Yet, not only are we seeing factually inaccurate answers delivered with confidence – but there have also been gross instances of dangerous and/or offensive responses. Not too long ago, the creators of OpenAI’s ChatGPT admitted their bot is “politically biased, offensive, or otherwise objectionable”. Until we have complete confidence that the dangers and inaccuracies have been removed, we must all question how we safely deploy these models, ensuring fairness for all users. This applies to all technology, regardless of its benefits, we cannot ignore its risks and current limitations – which can range from adversarial attacks to AI bias and how to determine the truth.
To be better at AI safety, and make sure we only offer ethical technology, we must guarantee that capabilities progress in tandem with safety and regulation to minimise the risk of unforeseen harmful consequences in different circumstances.
A game changer
Accuracy is one of the most important features of speech recognition specifically – a market that is set to surpass $43.35 billion by 2032. It has a cyclical relationship with inclusivity; a more accurate offering across a diverse range of voices is more inclusive. If a speech-to-text product can only work well for a subset of people, then it is both limiting and damaging as an offering.
Accuracy across the board, so that every voice globally can be accurately understood will result in an inclusive speech-to-text platform that has universal use and appeal. It is a moral endeavor that also maximises your total addressable market, because if a technology works well for everyone, then everyone is a potential customer.
However, it is not yet working as it should be, particularly if we look at Big Tech. A study conducted by the Proceedings of the National Academy of Sciences found that 23% of audio files of Black speakers resulted in an unusable transcript compared to 1.6% of files spoken by white people. Such a disparity shows a gross failing in speech recognition tech to truly understand every voice, as it produces disproportionate results depending on what the speaker looks and talks like. Big Tech consistently underrepresents (and consequently inaccurately transcribes) speakers that aren’t middle-aged white men with high socio-economic status. However, scaleups are bucking the trend and by prioritising accuracy, they are making significant gains on their competition across some otherwise overlooked demographics, such as those over 60. Recent research conducted by startups and scale-ups across a multitude of datasets and demographics has determined that Big Tech is no longer the market leader. New speech-to-text systems have been found to be more accurate than Microsoft and OpenAI.
Time for a song and dance
Historically, the accurate transcription of certain voices – particularly those of women, people of colour, the elderly and anyone with a regional accent – has been difficult. This doesn’t even account for those who might be deaf, hard of hearing, or have a speech impediment such as a stutter.
Recent strides in speech-to-text API have honed in on these marginalised groups so that they can be understood accurately. This, however, hasn’t been pioneered by Big Tech, where established players like Microsoft and Google have seen relative accuracy gains by smaller fast-growth companies on their offerings. While Microsoft and Google might have high accuracy levels for certain demographics, they fall short when it comes to understanding every voice – which is the only way to deliver widespread inclusivity and accessibility.
Some smaller businesses are now not only able to accurately understand the aforementioned groups but also singing – another previously difficult to transcribe input. While no easy feat, increased accuracy – which means understanding the way more types of people speak – comes by improving machine learning (ML) models such as using self-supervised learning that allows speech models to learn more from unlabelled audio (that doesn’t have a transcript).
How to achieve this
Any AI or ML technology is only as good as the datasets and algorithms used to train them. Ensuring that datasets are widely representative of the people who use the end product is a way to stop – or at least minimise – technology-perpetuating bias.
High levels of accuracy, across a wide range of speakers rather than the usual subset, requires training self-supervised language models on over a million hours of unlabelled data (so that a wider range of voices can be leveraged at scale) across as many languages as possible. Doing so, with the help of market-leading AI hardware means that speech-to-text providers can use Graphics Processing Units (GPUs) to supercharge their ML capabilities by training larger self-supervised learning models with more compute power. The model is then capable of learning richer acoustic features from unlabelled multi-lingual data, which allows you to understand a larger spectrum of voices. You can use word error rate (WER) to measure levels of accuracy. The WER is the proportion of the total number of errors over the number of words in the reference. If all the words are correct, then the WER is zero and that’s what developers and engineers are aiming to drive towards with every research decision they take. A speech-to-text system having a lower WER than competitors is a hallmark of a more accurate speech recognition offering. This is, in short, how speech-to-text vendors can bridge the digital divide and guarantee technology is accessible to all.
The road ahead
Finally, there is a conscientious endeavour to address the inclusivity blind spot in speech recognition technology.
Such efforts to improve accuracy through diversity of data are a steadfast way to lead us to unbiased speech recognition technology. Adopting diversity and enhancing accuracy levels will create platforms that are accessible for all, and have a consistent positive outcome for all users, regardless of distinguishing characteristics. The latest leaps in speech recognition technology has propelled its accuracy levels forward and established a new benchmark. However, the industry can’t stop there, as we have to continue to ensure that every voice is understood by speech-to-text platforms. This mindset means that those spearheading such advancements in technology and accuracy will push boundaries and set a new standard for speech recognition.