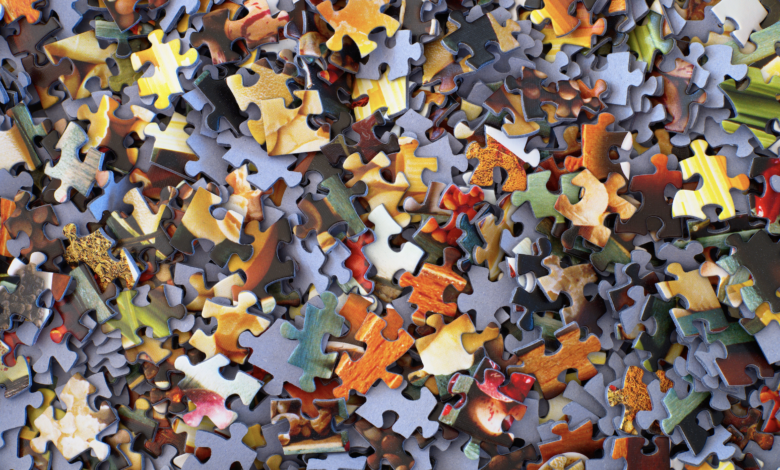
Process discovery is no longer a time-consuming and resource-intensive practice. Creating and analysing current state processes is now entirely automated. At least, that’s true for organisations that have successfully implemented process mining.
Process mining helps organisations discover and improve the performance of their processes, identifying bottlenecks and other areas of improvement. When implemented correctly, process mining reduces costs, improves SLAs and empowers teams to solve inefficiencies quickly. It has become a valuable asset for Business Analysts and Operations Excellence Consultants, but crucially, it provides management with rich insights about the performance of their operation.
If your organisation employs people to execute business processes using IT systems, then you should be exploring process mining.
How Does It Work?
Process mining combines data science and process analytics to mine log data from information systems. These systems, such as Enterprise Resource Planning (ERP) or Customer Relationship Management (CRM) tools, create event logs with every transaction which provides an audit trail of processes. This shows what work is done, when it’s done and by who.
Process mining software then uses this data to create a process model. This allows the end-to-end process to be scrutinised, showing the detailed steps and any variations. Built-in machine learning models help give insights into the root cause of deviations. For example, it might point out that every time a new customer needs proof of address, the process is slowed down considerably. These models enable management to see if their processes are performing as intended and if they aren’t they provide the information needed to optimise them.
Choosing where to apply process mining is vital. Organisations who apply it to processes that have already been digitised, i.e. processes that use core IT systems, tend to see the best results. It provides an evidence-based view of how processes are performing and it’s an easy sell to ExCo once they see where problems and opportunities lie.
What Are The Different Types of Process Mining?
There are three basic types of process mining: discovery, conformance, and enhancement.
Discovery: this technique uses event logs to create a model without outside influence. Under this technique, no previous process models exist to inform the development of the new process model. This type of process mining is the most widely adopted.
Conformance: this technique compares expectation with reality. This approach aims to identify any deviations from the expected model.
Enhancement: this technique is sometimes known as performance mining and is used to improve an existing process model. For example, the output of conformance checking prompts the identification of bottlenecks, allowing managers to optimise the process.
Why is Process Mining Important?
Lean six sigma has proved itself to be an effective methodology for reducing operating costs and increasing return on investment. However, identifying opportunities and measuring the effects of improvements has always been a difficult endeavor. Process mining helps by quantifying the inefficiencies in processes and showing management how effective any changes have been. The discovery of these processes can not only reduce costs, but it also drives more innovation, quality, and better customer retention.
A recent example of where process mining has been used successfully in the industry is with a large insurance company. A major source of inefficiency and cost for this company was their end-to-end claims process: from FNOL through to Claims Assessment and finally Claims Payout. Process mining was used to understand how c.300,000 claims were routed, which steps in the process had the longest lead times, which steps had the most variation, and why.
The company found that a combination of manual data processing, handling various documents and managing multiple hand-offs between third parties during the Claims Assessment was adding operating costs whilst affecting the customer experience. Within 8 weeks, process mining provided a rich map of the end-to-end Claims process, with clear insights on pain points and the number of opportunities for improvement. A combination of user training, process automation and process improvement initiatives followed, resulting in a 43% improvement in cycle times and over 1200 hours of time savings generated each month.
Since process mining is still a relatively new discipline, there are still some challenges to overcome. Those challenges include:
- Data Quality: Finding, merging and cleaning data is usually required to enable process mining. Data might be distributed over various data sources. It can also be incomplete or contain different labels or levels of granularity. Accounting for these differences will be important to theinformation that a process model yields.
- Process Changes: Sometimes processes change as they are being analysed, resulting in theprocess model shifting.
Organisations that strive to become digital businesses will need to enhance the ability to investigate andanalyse processes. The adoption of new automation technologies, such as RPA, Machine Learning andNatural Language Processing has proven that business leaders are continuing to invest in technologies that will help them improve business performance. As a result, organisations will increasingly lean on process mining tools to achieve their business outcomes.