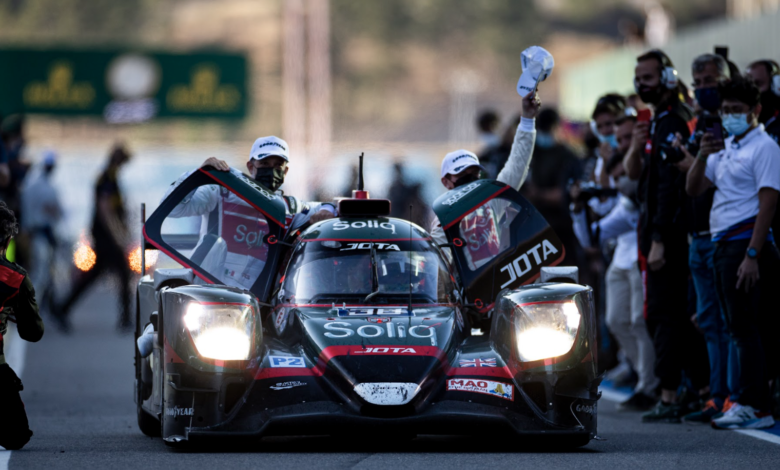
Dr Richard Ahlfeld, CEO and founder of Monolith AI, breaks down the two greatest barriers to augmenting a digital twin with AI and how these solutions are changing the world.
There’s a reason digital twin technology topped Accenture’s list of the top five technology trends of 2021. Regarded by many as the key to the fourth industrial revolution, digital twin technology is on the cusp of changing the way the world is designed and manufactured.
A digital twin is a virtual replication of a real-world design that is dynamically updated with data from its physical twin throughout its lifecycle. The ability to review and analyse a physical asset virtually helps with decision making, can avoid scheduling issues on building sites, or even maximise the performance of a wind turbine. The technology has become more widely used due to improvements in data integration and AI, and the wider democratisation of machine learning within engineering fields.
In contrast to classical model-based approaches used in engineering, data-based machine learning algorithms have proven their suitability. By continuously collecting and processing sensors, machines and other data, these methods provide an objective, data-driven design or simulation optimization as well as failure detection.
Digital twins can be used here to accelerate digital transformation initiatives for product development within sectors like urban planning, automotive design, manufacturing, and aeronautical engineering. The technology has rapidly grown in popularity over the last five years, with engineers, in particular, using this technology to help combat the location-based challenges the COVID-19 pandemic presented to them.
However, as this technology infiltrates development cycles across different industries, it’s apparent that there are still barriers for engineers to understand and be mindful of, in order to get the best usage from this technology. In a survey conducted among 250 engineers, lack of expertise, a too complex software landscape and a lack of real-time models, were cited as the top 3 barriers to adoption.
Most of the current academic research happening is focused on creating real-time models. There are two ways to achieve that.
On one side, there is data-driven modeling of the real-life system. For example, a race car’s full complexity can only truly be understood from real data. AI models like Neural Networks really help here because they can describe really complex systems. However, they are cumbersome to train and can become a complex ‘black-box’ without any physical interpretability and significant background knowledge on how to use them.
The main challenge is to therefore make them more accessible and explainable.
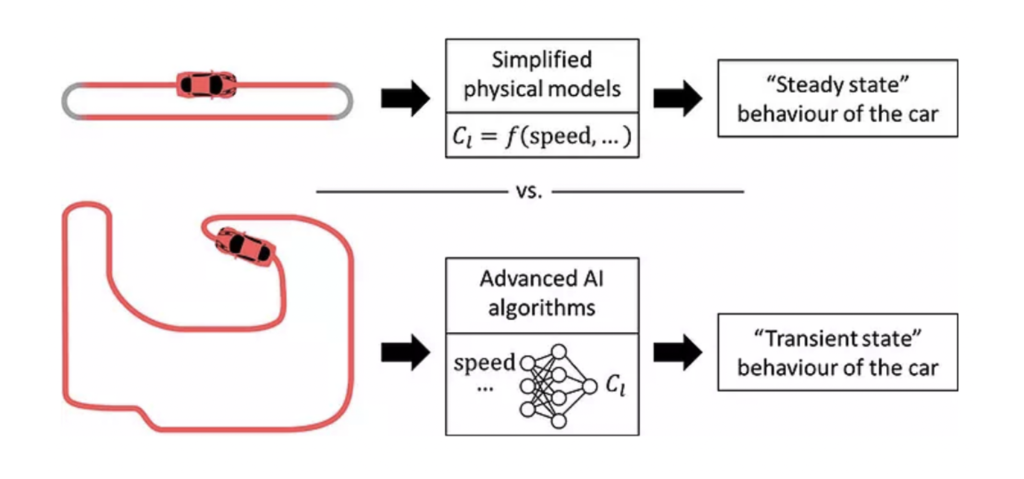
On the other side, there is the creation of faster surrogate models from traditional simulation methods such as Finite Element (FEA) or Computational Fluid Dynamics (CFD) simulations. They are interpretable and already widely used throughout the engineering industry. Yet, they are simply too slow to get a better understanding of complex physics in real-time. Here, the focus of the latest research is on using Physics-Informed Neural Networks (PINNs) to significantly accelerate or augment traditional CAE methods without losing the physical interpretability of the models.
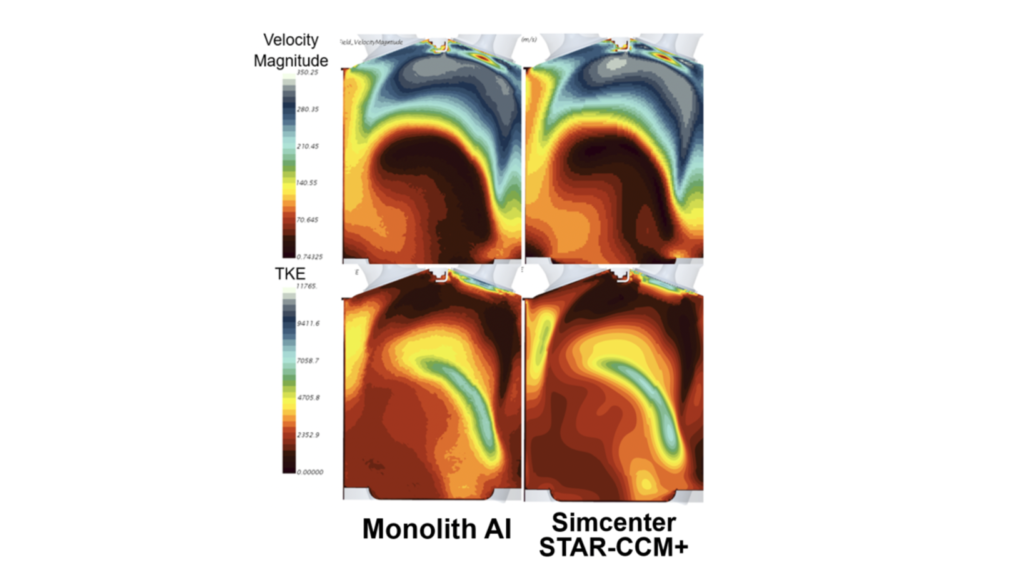
One of the main challenges that almost all engineers face when trying to create digital twins is hardly addressed by the majority of research happening in the field; the complexity of the software landscape and the level of expertise needed to build digital twins. This is one of the main reasons why I founded Monolith as a commercial business, and not just a research lab at Imperial College London. There was a strong need for software that could not just be published in Science but actually helped engineers create digital twins without being expert coders.
To give a concrete example, the Jota Sports Endurance Racing team are not software experts. However, they are using the Monolith platform to create a digital twin of their car from car setup, vehicle dynamics, aerodynamics, and tires. They train deep learning models on driving to allow them to rapidly make decisions in these key areas with dynamic models and predictive technology providing feedback on how driver performance and environmental factors impact car performance.
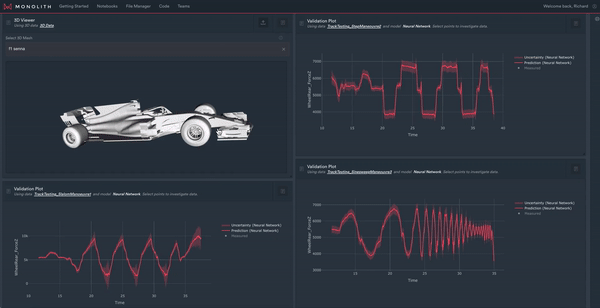
Tomoki Takahashi, technical director at JOTA, says that “Monolith has already radically changed how we operate within the engineering team here at JOTA. Their technology empowers our engineers to make faster, better design decisions and streamlines how our car and simulation data is validated. We are leaders in Global Endurance Racing and as the complexity of vehicle testing continues to increase, we’re confident Monolith’s AI-powered technology will support us as we continue to stay one step ahead of the competition.”
Overall, trying to model and understand complex multi-physical processes of systems is extremely challenging. Machine learning is increasingly being adopted within engineering design and simulation. Building digital twins using machine learning models has huge potential to revolutionise how we optimise different physical assets during an entity’s life-cycle, and to empower engineers when making difficult decisions with the added support and assistance of fast machine learning models making intelligent recommendations.