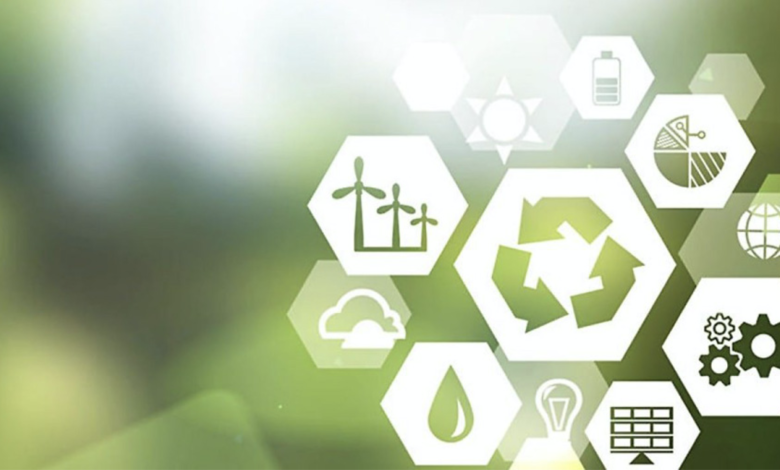
We are in the early stages of the Fourth Industrial Revolution (4IR), a fusion of advances in artificial intelligence (AI), robotics, the Internet of Things (IoT), 3D printing, genetic engineering, quantum computing, and other technologies.
The journey to digital transformation is at 4IR’s core and decision makers and analysts aim to make everyday processes simpler and more efficient. The aim is to fuel innovation and take advantage of new opportunities, new business models – within the boundaries of regulatory obligations.
There is a large potential for AI to contribute towards global economic activity, especially towards Environmental, Social and Governance (ESG) investing. A report released by the World Economic Forum (2020), found that around 70% of its targets to tackle environmental issues such as climate change and habitat loss, as well as social issues ranging from poverty through to inequality, could be tackled by harnessing the power of technology. Looking at the next decade, the WEF gives predictions, based on the applications of today, on what can be achieved in health, clean energy, industry, innovation and infrastructure.
Vision2030, launched at the World Economic Forum Annual Meeting in January 2020, provides a focal point for the mobilization of a more concerted and cooperative effort to apply advanced technologies to the achievement of the United Nations Sustainable Development Goals (U.N. SDGs).
It serves on one hand, as a global node and facilitator of networks of providers and users of technology solutions for sustainable development; on the other, it will advance intentional curated efforts, partnership building, government capacity development and finance to fast-track new technology solutions for the Global Goals.
The platform seeks to enable 4IR technology partnerships, focussing on three core objectives:
- Help countries identify and match technologies that address their specific Global Goals implementation priorities.
- Catalyse further collaboration to unlock barriers to breakthrough technology innovations for the Global Goals.
- Proactively convene key stakeholders to cultivate an enabling environment for 4IR innovations to drive positive outcomes.
This Fintech-backed initiative does not stand on its own but supports an ever-increasing trend of hybrid ESG / Green Finance, SDG + financial solutions, backed by public-private partnerships, NGOs, as well as investment firms, global exchanges, and other solutions and services providers.
Meanwhile, many ESG and mainstream financial data aggregators, solutions and index providers have started to leverage Fintech and a combination of AI, and other similar Machine Learning (ML) technologies, to screen, mine and analyse big data, from online sources. They apply AI ontologies to their research outputs to assess, rate, classify, benchmark, monitor, triage and report on extra-financial data sets. They look for the typical ESG indicators, in the context of “trends”, “risks”, “behaviours”, “sentiment” and/or “consistency” criteria, and to Fintech and AI, to identify these issues and potential controversies, in real time.
AI- / Fintech-powered ESG screening and analysis solutions have become “strategic enablers” that can address some of the inherent ESG information biases and potentially even ESG rating divergences arising from corporate self-reporting, and annualised, backward looking reporting of information. In this context, MIT Sloan (2019) found that the correlation among prominent agencies’ ESG ratings using this ESG information was on average just 0.61; by comparison, credit ratings from Moody’s and Standard & Poor’s are correlated at 0.92. This ambiguity around ESG ratings creates acute challenges for investors trying to achieve both financial and social return.
Moreover, corporate disclosures can vary by region, with companies in developed economies in general disclosing more information than companies in emerging economies. In such cases, the “alternative data” is key to endeavour for capturing more information from these “black-box” companies for extra disclosures.
New Fintech-, and AI-backed business and investment models can go a long way towards addressing key investor concerns and towards while supporting a regulatory push for more consistency and transparency in corporate reporting, auditing and research and ratings analysis, acting as the catalyst for sustainable, ESG, SDG and impact investing at scale.
Potential for artificial intelligence in ESG investing comes from sentiment analysis algorithms which allow computers to analyse the tone, type, and pattern of a conversation by comparing the words used to a reference set and classification system of existing definitions. As ESG investing is evolving towards a more dual and dynamic materiality concept, sentiment analysis in turn needs to constantly evolve to capture different nuances and their context.
However, adopting AI and Fintech such as Blockchain can also pose a significant negative environmental impact. The process for creating and training AI algorithms requires large amounts of computing power and capacity, which in turn consumes large amounts of electrical energy.
Further work needs to be done to address inherent biases in sentiment analysis algorithms that are programmed to replicate and reference existing factors and patterns. If reference points such as ESG definitions, KPIs and frameworks are not clear, information biases and (scope) divergences will remain.
To address these challenges, the Sustainability Accounting Standards Board (SASB) announced, in October 2020, to engage PwC’s XBRL Practice to support an XBRL-led non-financial taxonomy. Many regulatory bodies already mandate the use of XBRL for financial disclosures in their jurisdictions. The European Securities and Markets Authority (ESMA)’s European Single Electronic Format (ESEF) and the United States Securities and Exchange Commission (US SEC) mandate XBRL tagging of annual financial reports, as have many other regulators and exchanges. It is hoped that providing a common language for business reporting, XBRL can enhance the usefulness of SASB disclosures.
These latest developments and innovation-centred collaboration efforts between regulators, standards setters, assurance, and innovation providers can provide a meaningful approach for ESG data complexity management.