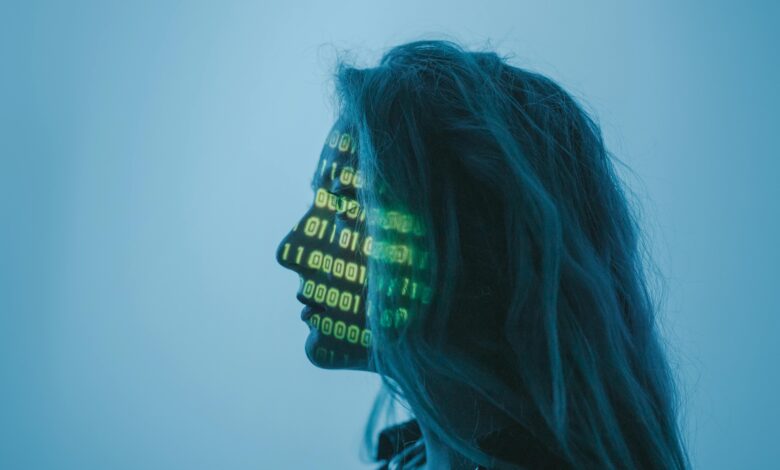
The rise of Generative AI and Large Language Models (LLMs) has accelerated productivity and unlocked a diverse set of use cases in enterprises. In a particularly impactful use case, generative AI and LLMs are helping companies to sort through the large amounts of data they’ve compiled, to provide predictive insights that drive business operations and strategy.
Some 74% of organizations surveyed by Deloitte said that their advanced Gen AI initiatives are meeting or exceeding expectations. According to multiple reports, the Enterprise AI market is set to cross the $100 billion mark by 2030.
Let’s take a closer look at some key trends in the use of GenAI for business analytics, where enterprises are seeing the most benefits, and what needs to change so that businesses can derive even more value from their AI-powered analytics in 2025.
Simplifying Access to Analytics
Companies adopting AI-enhanced analytics are better equipped for critical thinking and handling complex business scenarios than those that don’t use AI. Before the rise of GenAI, if the top brass in organizations wanted to leverage business intelligence and data analysis, they needed to ask a specialist to build a custom report, then wait for them to fetch relevant data, hopefully also creating a visualization around it to present to other stakeholders a snackable manner.
In the traditional field of business analytics, you need to know SQL to talk to the data. However, the advent of generative AI means that anyone can be a data whisperer by using natural language queries. This helps companies to scour gems from the under-utilized data troves they already have.
IBM CEO Aravind Krishna recently said that only 1% of enterprise data has made its way to AI models, and that’s why there is a huge opportunity for companies to unlock new value.
“If we’re being honest, most companies just don’t make the most of their data, and that’s mainly because traditional BI and analytics tools are stuck in the past. They’re powerful but often too complicated, intimidating, or not designed with different users in mind,” said Omri Kohl, CEO of Pyramid Analytics, whose company integrates GenAI with BI to offer a “GenBI” solution.
“Instead of having to navigate through menus or run complicated queries,” he continued, “with GenBI you’d just get instant, clear answers based on live data, presented in any way that’s convenient to you, whether it’s charts, graphs, or a verbal explanation.”
Mixing Things up Technically and Visually
New-age AI models have given us the ability to choose the best possible solution for enterprise usage. Different models have different levels of efficiency and capabilities in various areas. This gives us an opportunity to match the model suited for a particular query and get the best result.
Plus, this strategy also has cost benefits, making it lucrative for organizations to adopt AI. Think of a scenario when a cheaper open-source model like Deepseek arrives on the scene, nimble companies can integrate them into their workflows and reap gains on multiple fronts.
AI doesn’t just help companies become efficient on the backend. The technology can aid in presenting data in a visually appealing and more readable format.
The visual suit of traditional enterprise tools was limited, so the output was stuck in the past. With generative AI, both existing and new tools can take advantage of a vast amount of innovative formats for visualization.
Protecting Customer Data
Privacy and security are foundational values of good enterprise AI products. There is a reason why so many companies have barred their employees from using public versions of chatbots at work over data leak concerns.
AI tools that are used in the business area are exposed to an unprecedented amount of sensitive data, so they need to have data protection as a core feature to even have companies try their product.
Top solution makers are adopting techniques such as strong data governance through robust access control, an encryption layer to protect data, and separating the large language model and sensitive data through an AI-powered query engine and federated learning.
The CISO of Germa development agency GIZ, Eckhart Mehler has asserted that data privacy is paramount in terms of building trust and a sustainable future. “Data privacy and responsible AI are not mutually exclusive; rather, they are mutually reinforcing,” he recently wrote.
“Organizations that prioritize ethical considerations in AI development foster trust, which is essential for long-term success,” Mehler continued. “Moreover, ethical AI practices contribute to achieving the SDGs, paving the way for a sustainable and equitable digital future.”
Future of Gen AI Usage with Enterprise Data
As 2025 takes shape, more and more enterprises are set to integrate AI into their workflows. Gartner predicts that by 2030, usage of AI code assistants will jump to 75%. Companies are also already experimenting with agentic AI frameworks to automate their tasks and workflow to make the workforce more productive by engaging them in more critical tasks.
In the next few years, companies will also use AI to organize their data better to take advantage of natural language queries. Likewise, they will automate financial reporting, customer service, and other operational processes. There is also a huge opportunity to drive customer delight by powering personalization through AI.
And as GitHub CEO Thomas Dhomke pointed out, this change will be driven from the top. “Just as every company became a software company, we now live in a moment where every company must become an AI company,” he noted. “Cracking the code of AI transformation must be CEO-driven,” he said.