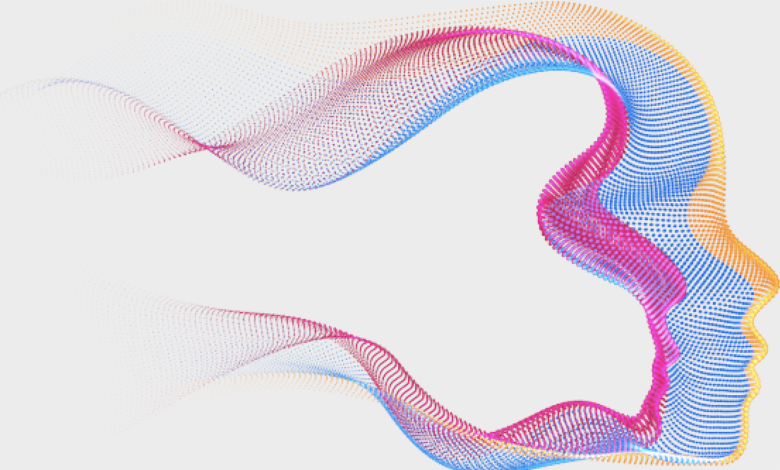
While artificial intelligence (AI) seems to be advancing at a much faster pace than even the most optimistic experts ever thought possible, the leap to AI’s next phase – the ability of a machine to understand or learn anything that a human can, better known as artificial general intelligence (AGI) – apparently is being slowed by the very human need for instant gratification.
Think about it. Self-driving cars are already in use on our highways, but if a feature was produced that suddenly enhanced vision capability and in doing so drastically improved the safety and reliability of those self-driving cars, it would immediately be rushed to market because it is commercially viable. Similarly, if a new feature that improved language skills and understanding was developed, it would be rushed to market because it would revolutionize the already formidable skills displayed by personal assistants and online search functions.
In short, the market’s unquenchable thirst for instant gratification is resulting in each of these new capabilities being marketed individually as soon as they become available. As a result, the commercial need for short-term results may be impeding the long-term development of AGI.
Think of what would happen if each of these more specialized, individually marketable AI systems could be built on a common underlying data structure. Doing so would allow them to begin interacting with each other, building a broader context that would actually understand and learn. As these systems become more advanced, they would be able to function together to create a more general intelligence, suggesting that at some point we’re going to get close to the threshold for AGI, then equal that threshold, then surpass the threshold.
Given that, it would seem that getting to, and eventually exceeding, that elusive threshold is inevitable because market forces eventually will prevail. But it is also likely to be gradual. At some point, we are going to have machines that are obviously superior to human intelligence and we humans will begin to agree that yes, maybe AGI does exist. To get there, however, we may need to change our current approach to AI.
Take, for example, the current poster child of the AI world, OpenAI’s ChatGPT. Without a doubt, ChatGPT possesses some very impressive capabilities, including remembering a dialogue thread, creating content, and generating AI art. But like other language models we might encounter, it is entirely dependent on a large dataset of text and uses machine learning techniques to predict the next word or phrase in a sequence. And while it has been optimized for conversational text and can generate responses that are more contextually appropriate and coherent than those generated by other language models, it is still manipulating symbols without any understanding of what those symbols mean.
To make the leap from AI to AGI, researchers ultimately will need to shift their focus away from
ever-expanding datasets and machine learning models to a more biologically plausible structure that contains four essential components of the appearance of consciousness:
- First, the ability to understand that physical objects exist in a physical environment will be needed for a system to become an AGI. But while words are most useful in representing objects, thoughts, or ideas which exist in reality, concepts such as music or art and physical objects which have textures, tastes, and smells are often difficult to express in words alone. To overcome such limitations, multi-sensory inputs and an underlying data structure which supports the creation of relationships between multiple types of data must be incorporated into the AGI.
- Next, an AGI will require an internal mental model of surroundings with the AGI at its center. Doing so will provide the AGI with a point of view, mimicking the way in which humans see and interpret the world around them,
- Thirdly, the AGI must possess a perception of time which allows for an understanding of the impact of current actions on future outcomes.
- Finally, the AGI will need an imagination so that multiple potential actions can be considered, the outcomes of each evaluated, and the most plausible choice selected.
In short, for AGI to emerge, it must begin to exhibit the same kind of contextual, common-sense understanding as humans do to experience the world around them. To get there, AI’s computational system must more closely resemble the biological processes found in the human brain, while its algorithms must allow it to build abstract “things” with limitless connections, rather than the vast arrays, training sets, and computer power today’s AI demands.
When considering the likelihood of such a system, it’s important to recognize that the human brain does all these things in an organ which weighs about 1.5Kg and uses about 12 watts of energy. But while we know a lot about the brain’s structure – that it is defined by each individual’s DNA and that the complete human genome is only about 750 megabytes – we don’t know what fraction of our DNA defines the brain or even how much DNA defines the structure of its neocortex, the part of the brain we use to think.
If we presume that generalized intelligence is a direct outgrowth of the structure which is defined by our DNA and that structure could be defined by as little as one percent of that DNA, we might be able to define a complete AGI in a program that is as small as 7.5 megabytes, something researchers easily could write in just a few years. As a result, the real AGI problem is not one that requires gigabytes to define, but really one of what to write as the fundamental AGI algorithms. One thing is certain: AGI is inevitable and the sooner we focus on how the brain works and what its algorithms are, the faster AGI will emerge.