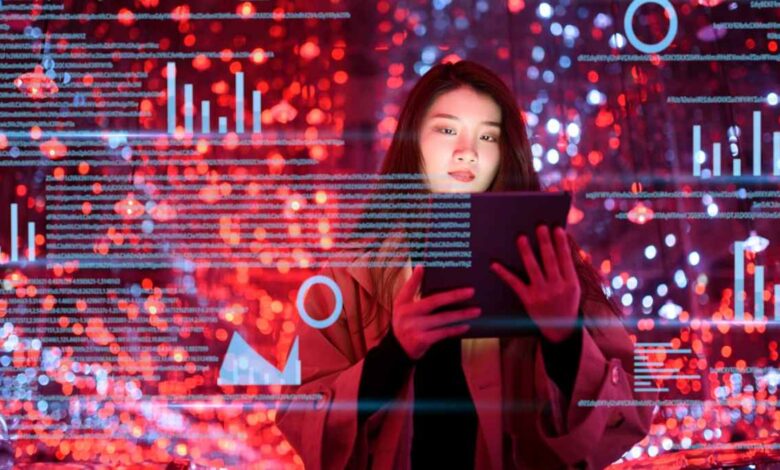
The way we consume news has changed significantly over the last decade. Gone are the days of having to wait for the 6 o’clock news to catch up on the events of that day. Reading the newspaper with a morning coffee is no longer a daily ritual for many of us because it is now neither the most efficient nor engaging way for us to consume media. Our 24/7 access to the internet via smartphones means that we can keep up with the news literally as it unfolds.
In addition, the media forms we have access to now are far more diverse than they used to be, and have evolved to be as engaging as possible to compete for our increasingly short-lived attention spans. TikTok videos, Instagram photos, LinkedIn posts, discussions on X… all of these forms of social media content and more offer us not just real-time snapshots of what’s going on in the world, but also the often greater appeal of seeing other people’s reactions to it and the opportunity to engage with it ourselves.
The great power of social media lies in its potential to amplify the voices of ordinary individuals to a global audience, a privilege previously reserved for just VIPs and celebrities. The potential for an individual voice to go viral and gain millions of views holds endless and tantalizing appeal for many of us as a quick and easy way to gain fame and status.
But the amplification of individual voices has turned many social media platforms into frenzied and overcrowded marketplaces full of influencers and content creators, all vying to get the most clicks, views, and reactions. Algorithms inset into many platforms to promote the most popular content magnify this competition and make the endgame primarily about grabbing the attention of browsers. This has had a severe impact on the type on content generated on social media, and led to an overall decline in the quality of media that most people consume on a daily basis.
The situation has been further inflated by the proliferation of generative AI, which takes down the time needed to create content from hours to just seconds. Furthermore, generative AI can compete with human creativity by producing a seemingly endless stream of content on the same topic using a finite set of information. But while content overload and the resulting decline in media quality is a problem that has certainly been accelerated by AI, it is also an issue that AI may be able to help us to manage.
Addressing content overload
The problem of content overload has given rise to an emerging consumer demand within the media industry: a need for informative and digestible summaries of the news. For many, keeping up to date with the news is an imperative for their jobs or lifestyle, but one that can be time consuming.
In response to this emerging need, a number of companies are stepping into this gap in the market to provide the service of media management.
For example, 1440 Media delivers a daily news breakdown to its subscribers’ inboxes, eliminating the need and temptation for them to doom scroll through news feeds in order to stay up to date on the world’s developments. Co-founded in 2017 by venture capitalist Tim Huelskamp, the company is run by a small team of human editors who scour over 100 news sources for information, and then create a concise daily summary of the news based on them.
Other companies are taking a less traditional approach to provide a similar service.
Otherweb, for example, utilizes machine learning to evaluate and summarize news articles from approximately 900 sources across the web. But in contrast to 1440, where human editors essentially rewrite the news in a more concise and factual form, Otherweb provides an automated summary and a ‘nutrition label’ for existing articles to inform the reader of what they are going to be reading about before they click on the article.
Additionally, within the social media world, Elon Musk has recently rolled out a ‘stories’ feature for premium subscribers to the social media platform X. This feature is powered by the platform’s AI chatbot, Grok.ai, and will provide a summary of the latest news and trending content on the platform.
Such use-cases demonstrate the broad set of applications for AI within key media management tasks such as content summarization, filtering, and evaluation.
With the potential for AI to be further integrated into other news and social media platforms, alongside growing demand for an antidote to content overload, the world may be on the cusp of a new era of AI-managed media consumption.
Below, we look at the key implications of AI’s curation of media. In particular, we consider how effectively AI can mitigate bias in the news, the impact of personalized news feeds, and the potential risks of relying on algorithms to dictate what we read about in the news.
Tackling bias in the news
Within the news and media space, the issue of bias is difficult to avoid. Articles are filled with the perspectives of their authors and/or the outlets that they write for – and, no doubt, opinion certainly has its rightful place within the news.
However, the media industry has a notorious reputation for propagating the deep-rooted political and cultural biases of dominant political organisations and majority groups. This is damaging particularly to minority groups within a society, and can give rise to misinformation, ignorance, and prejudice.
By tracking features and phrases associated with bias and prejudice, AI can be used to mitigate some of these issues and risks. For example, the European Commission against Racism and Intolerance (ECRI) uses AI to detect and remove hate speech in social media posts.
Another company, the Bipartisan Press, is utilizing AI to tackle political bias, rating articles with a score from -42 to +42 to indicate how heavily respectively left or right leaning they are. According to the company’s own research, the algorithms provide an accuracy rate of 96%, though this is clearly subject to their own subjective views on what constitutes political bias.
Other media management companies take a different and less direct approach to bias. Otherweb, for example, works by passing news articles through a set of ML filters which have been trained to look for certain, predetermined features that are associated with bias. These include features such as known propaganda techniques, attention grabbing headline, and source diversity.
Alongside these specific features, the filters also evaluate the overall linguistic style and tone of the article, providing a score out of 100 for informative language and neutral language, which can be further indications of bias/subjectivity of opinion.
One of the benefits of the rating/scoring models which the Bipartisan Press and Otherweb use is that they do not attempt to actively remove bias from the content. This indirect approach eliminates the risk of the algorithms instilling any biases they might have into the content of the article.
By contrast, applications which rewrite the news, or automatically remove bits of content, face more risk of propagating other forms of bias which might be innately built into the AI model. Previous uses of biased models have shown just how damaging this risk can be. In particular, such use-cases for AI have higher risk of silencing and disproportionately targeting minority groups, as discussed in a study by Gerrard and Thornham.
Amazon’s facial recognition technology, for example, was found to be propagating skin colour bias when it falsely matched 28 members of congress to mugshots from a database, and these mismatches disproportionately targeted coloured members.
Overall, AI itself is still subject to some element of human bias, even if this might just be in terms of deciding which linguistic features are indicators of bias in the news. Therefore, given that AI is not free from bias itself, it cannot yet be depended on to completely eliminate bias within the news. Nevertheless, it may still have significant utility in identifying potential biases. This can help readers become more attuned to the overall reliability and factuality of a news article, and make them less susceptible to inherent biases in content that may otherwise go unnoticed.
On the other hand, as AI becomes more widely integrated into the media industry, it will become increasingly important for consumers to remember that while AI is in itself a neutral and objective technology, it is still just a tool that can be manipulated. For example, it would be incredibly easy for social media tycoons such as Musk or Zuckerberg to finely tune algorithms on a select variety of training data in order to propagate various biases and promote particular types of information.
Indeed, most media outlets and social platforms have their own agendas, or will edit/manipulate the content they publish and promote in order to survive. For example, a big appeal of X to its subscribers is the potential to view and share controversial opinions, and engage in heated discussions, more than having access to reliable and neutral information.
For this reason, the input data for Grok’s ‘stories’ feature will not be based on news articles and the actual information itself. Instead it will be a summary of the conversations and opinionated posts that have been sparked in response to a news story, as revealed in an X post from tech journalist Alex Kantrowitz.
In a TechCrunch article, Sarah Perez argues that this is as both a clever and a worrisome move which raises concerns from a misinformation standpoint. Indeed, Musk’s plan shows that if AI has the potential to eliminate misinformation, bias, and sensationalism, it has just as much potential to propagate and spread this type of content.
On the other hand, it also shows how flexible AI is; as a versatile technology, it can be tailored to meet consumer needs whether this is to get a quick summary of news presented as bare facts, or whether this is to catch up on the conversations and opinions that have been sparked by a development in the news. There is demand for both, and AI can help businesses cater to them either way.
Personalization of the news and the dangers of ‘filter bubbles’
Asides from countering bias, a key way that AI is being integrated into the news is to filter and personalize content to individual consumer preferences. This is no surprise. Personalization is a key AI trend that is currently impacting many consumer-serving industries, and bringing many benefits including enhanced consumer engagement and satisfaction.
Within the media industry these benefits are clear. With a personalized feed of content that is customized to individual preferences, consumers will be able to spend less time searching for the type of content that they want to find. Additionally, personalization can also help consumers to expand their repertoire of knowledge through suggestions of new content and topics they might be interested in based on their existing preferences.
However, there is a risk that comes with personalization that is specific to the media industry. This is what is known as the echo chamber effect, where consumers will end up reading a filtered bubble of content that simply reinforces their beliefs and world view.
Indeed, a personalized feed of highly subjective articles could end up simply validating a reader’s existing views and opinions, rather than challenging them with new perspectives. This matters because exposure to new and opposing points of view is a key way that people become more intelligent, tolerant, and resilient.
In a Medium article, Titus Plattner highlights the key risks of personalized news feeds, but argues that these risks are overrated given that people are already able to narrow the scope of their content consumption just by choosing which news outlet to read from and which news channels to listen to.
Nevertheless, he admits that personalization could potentially accelerate our tendency to surround ourselves in a comfortable bubble of familiar and agreeable content, particularly in cases in cases where the reader is surrounded by articles that are highly opinionated and/or analytical.
Otherweb CEO Alex Fink agrees, but argues that this risk can be managed by basing personalized content feeds on thematic interests rather than just likes or dislikes.
“There is this idea of like and dislike that every social media platform right now is built on. But in our case, what we’re trying to infer is not whether the person likes what the article says, but whether they are interested in the topic. We’re trying to decouple those things and focus on topics you’re interested in as opposed to whether you like or dislike a particular point of view.”
Alex Fink, Founder and CEO of Otherweb
Otherweb allows users to manually configure their news feed using several sets of gradable filters, allowing the user to indicate the proportion of content they would like to see on a particular topic, from a particular source, relevant to a particular location, or even with a particular emotion in its tone.
The intent is to allow users more agency and control over what they read. But while the approach of promoting thematic interest over the like/dislike response may help to reduce the effect of filter bubbles in some cases where articles are highly subjective, it remains unclear how this tackles the risk in cases where the articles are more informative and balanced.
For example, let’s say a more emotionally sensitive reader decides to reduce the level of depressing emotions in the content he reads. Given that most regular news is just innately negative and depressing, the user will be creating a potentially harmful filter bubble, ostensibly protecting him from content that he might find emotionally triggering, but which in reality just misleads him about reality and prevents him from seeing a lot of what’s happening in the world.
Another way that personalization will be implemented within the media industry is through chatbots. The impact of this development may be felt less explicitly given that most of us are now accustomed to having a customized response to even quite complex questions spun out within seconds by ChatGPT or other publicly available LLMs.
Nevertheless, it represents a significant new form of media distribution, and one which means that personalized news consumption could become a new standard for the industry. Fink, for example, suggests that the future of the industry will be fundamentally shaped by the use of chatbots.
“I think that chatbots might replace feeds entirely in the future because it’s more efficient than the user trying to configure with sliders, or in any other way, and see a list of articles you’re going to consume.”
Alex Fink, Founder and CEO of Otherweb
Chatbots certainly add a greater degree of convenience to the process of personalization, especially with the new ‘memory’ feature that chatbots such as ChatGPT have recently rolled out. But it remains to be seen if there will be much difference for consumers between getting news updates from general-use chatbots and media-specific ones such as the ‘news concierge’ chatbot that Otherweb has recently introduced.
Can we rely on algorithms to manage content for us?
It is easy to see the value of AI in media management, with its potential for summarization tasks, identification of potential biases, and personalization of content to individual preferences. Above, we have highlighted both the risks and benefits of these use-cases.
However, there is an additional, existential risk to using AI to manage our news consumption.
This risk is more related to the innate weakness of the human condition rather than the limitations of the technology itself, and comes from the trust we are placing in algorithms to evaluate content for us.
For better or worse, the impressionable nature of our human brains makes us vulnerable to manipulation from both other humans and machines. The particular danger of AI and its management of information is that it is widely perceived to be neutral and unbiased given that it doesn’t have any personal motive or agenda.
Indeed, for many of us (myself included), the mere availability of an automated article summary or some form of algorithmic evaluation, will reduce our motivation to read the original article and use our own critical thinking abilities to come to our own conclusions. This may inadvertently increase our dependency on algorithms to ‘think for us’.
Over time, this could make us inclined to view content almost exclusively in light of its algorithmic evaluation, essentially letting AI dictate our thoughts and opinions. It could be argued that this is not necessarily a bad thing, given that in many ways AI is already smarter and more rational than human intelligence. Nevertheless, for the moment at least, AI is still prone to making errors and ‘hallucinating’, particularly when it comes to more complex cognitive processes.
Thus, the real danger of AI in media management may simply lie in our disinclination to question what we are told, particularly when the answer comes from a machine.
On the other hand, you might argue that this is the exact human tendency that media management services essentially aim to cater for? After all, if AI can’t be used to do the cognitive tasks that we are unwilling or unable to do for ourselves, then really what is its point?
Dr. Eric Siegel, writer, professor and seasoned expert in machine learning, acknowledges the risk of relying on algorithms given that they are still error-prone, but suggests that the degree of risk also depends on the costliness of AI making errors in the given context.
“For most labelling/categorization tasks, machine learning is the best approach. But it will make mistakes. In general, we don’t have crystal-ball levels of performance, but we do have performance that’s much better than guessing. So, how costly are such errors? For labelling news stories, will the end user lose out substantially because they choose not to read an article on the basis that an ML model wrongly labelled it as “click-bait”, “fake news”, or the like? Or do they not really care that much?”
Dr. Eric Siegel
And while this is a rhetorical question for us to mull over, Siegel proposes the two plausible ways to find out the answer:
- Collecting hard data from end users to find out how often they encounter errors, and how badly it has impacted them when it happens.
- Just putting the AI system out there to how many people pay for/use it. This ‘wild west’ approach (as Siegel calls it) means that users may not know how much misinformation they are being exposed to, but over a long period of time, the performance and reliability of the system should become familiar to regular users.
In practice, despite the emphasis on data in today’s world, many companies will likely take the ‘wild west’ approach for the simple reason that it takes less effort. Thus, consumers are essentially the guinea pigs for many applications of AI.
Within media management, the risk remains that AI and algorithmic evaluation could impact our ability to digest content and think critically about it. This is a risk that most companies are going to be willing to take, as long as there is consumer demand for the AI service they provide. Therefore, the future of AI in media management ultimately depends most of all upon consumer trends and its perception in the public eye.
If and when this changes, it will be a sure sign that AI has truly ushered in a new era of media management, an era where AI can even control its own public image.