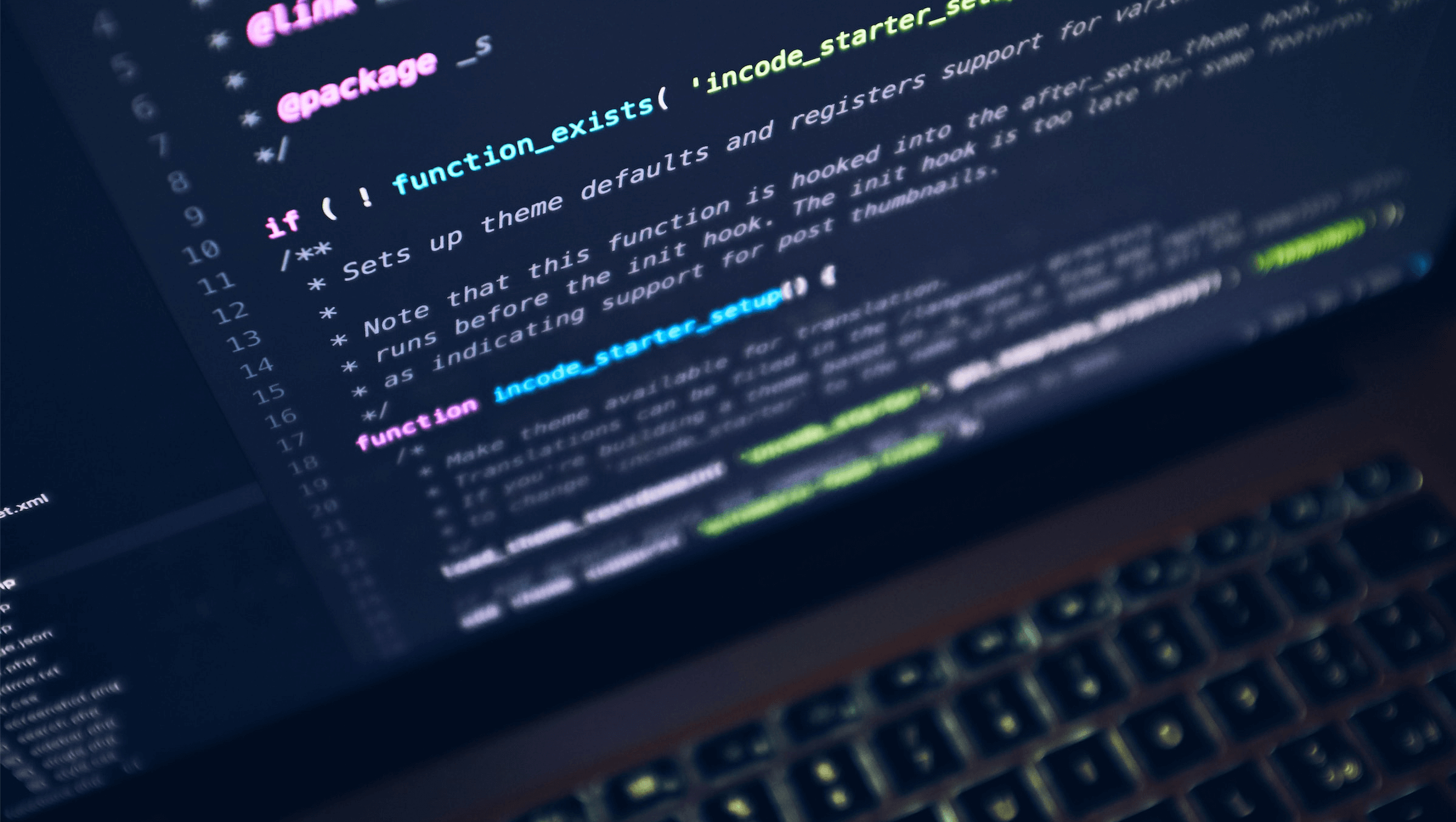
Efficiency is on the mind of every organisational leader. There is a spectrum of business risks to manage while balancing the drive to innovate and improve customer experience. Striking that balance is difficult given the macroeconomic environment and geopolitical instability affecting the supply chain and business environment.
Yet, even with the lingering effects of the pandemic, banking sector instability, and inflation all affecting businesses and consumers alike, customer expectations remain as high as ever. The pace of innovation demanded by the business to delight customers has not slowed down; in fact, itās only accelerated. However, the number of technical resources supporting these services often is. Developers and engineers are being asked to do more.
According to PagerDuty platform data, event volumes coming from monitoring and observability tools continue to grow by 70% YoY. This indicates that more services are getting created, and technical resources are being asked to do more. However, headcount, especially in the past year, is not growing at the same rate, and in many cases not growing at all.
Digital is everything. Digital operations are the bedrock of how we provide services and produce goods. Getting it right is essential to modern business operations. AIOps has rapidly gained traction to support these processes and boost efficient outcomes.
Deploying AI to make sense of the chaos and focus on what matters
Logs, traces, metrics, events⦠Teams are drowning in data – they donāt need any more data – what they need is actionable information. The signal-to-noise ratio has risen too high for people to manage manually on their own. Making sense of the volume of data businesses are processing today requires intelligently filtering events down to the incidents that matter and need human attention.
There are two ways to reduce noise. The first is the case of the flapping or transient incident. If an incident has historically shown that it resolves on its own shortly after it’s created, then there’s no need to alert a human in the first place! Machine learning can help by automatically detecting and pausing these transient alerts, so they don’t turn into human-impacting incidents. Nobody wants to wake a responder up or take them away from what theyāre doing unnecessarily. The second way to reduce noise is to leverage intelligence to correlate and group similar alerts using payload data and user interactions so that developers arenāt bombarded by alerts that are really about the same thing. Ideally, models should continuously learn based on this information, reducing the need for human attention.
By identifying incidents that matter, we greatly improve operational efficiency. Setting automation as the first line of defence takes the human out of the equation until theyāre absolutely needed. With this set up, humans avoid toilsome work since they no longer have to sift through events and determine what is important, which frees up engineering time to work on value-added initiatives. It also reduces downtime and customer impact because incidents are identified and triaged faster. Finally, it supports a healthier working environment, lowering the risk of staff churn and burnout as responders no longer have to pivot between tools, sift through noise, and troubleshoot irrelevant incidents.
Augmenting automation with AI
Despite progress seen by the DevOps movement, the reality for many organisations continues to be a manual, reactive state of digital operational maturity. Thereās often legacy infrastructure and services that emit a high volume of event data and require manual processes to remediate issues. This legacy infrastructure comes with an increased risk of outages – resulting in lost revenue and lower customer satisfaction. Legacy systems were often built as a monolith, so there’s spaghetti code and often few developers actually know how the system works. It’s difficult to break down and replace, and rapid digital transformation often results in simply āsticking plasterā on top. These incidents are harder to manage because there’s a lack of tribal knowledge, and issues typically cascade in ways that technical teams can’t predict and haven’t documented. It all increases complexity and engineering toil.
Yet to run a modern organisation requires always-on digital operations. Part of achieving a more mature state involves accelerating the incident response process using automation driven by AI recommendations.
Using AI to identify the automation rules or scripts that can reduce toil on developers and engineers is critical. By leveraging AI to make sense of streams of data coming from a variety of monitoring tools, teams can return services to normal faster. With a limited number of domain experts, they must be deployed where a critical decision needs to be made and action taken. An example use case is automated diagnostics. When an incident that requires human intervention happens, engineers must have context at their fingertips in order to fully understand the root-cause of an issue. Having event-driven automated diagnostics proactively collect relevant information from systems and services saves steps and valuable time.
Teams can also use event-driven automation to remediate redundant issues that donāt need involvement of a developer. Event-driven automation can span from real-time event ingestion to complex multi-step logic, to action being taken. Well-defined automation workflows and processes lead to greater stability and business resilience. Organisations can leverage modern AI technologies (e.g., ChatGPT) to generate suggested automation workflows. These AI-generated automation workflows, once a pipe-dream, are becoming increasingly accurate at determining the right path to resolution and acting as a level zero responder.
Removing these manual processes not only improves the developer experience, but also results in a positive impact on customer satisfaction that can show up in reduced churn and increased revenue.
Operational maturity for AIOps and automation
Investments in AIOps and automation can empower teams and accelerate operational maturity and resilience. This comes with several additional benefits. Work hours become more consistent when teams are more operationally mature. Attrition and burnout rates improve with more consistent work. Response times are reduced, and staff get to use their time more intelligently and meaningfully. In a time where the business is under pressure to maximise resourcing – more engaged, productive employees will have better capacity to deliver stronger results for the business.
Success requires a team effort and a committed, staged approach. Operational and developer teams should agree on KPIs and develop a systematic method for tracking them. They must identify noisy services and look for opportunities to tune systems and tools. Teams must uncover repeatable steps in operational processes to automate redundant manual tasks. Tools must enrich and add context to events so that incidents are easier to troubleshoot and remediate. Insights should power smarter operational and strategic decision-making. With this in place, well-connected and properly orchestrated systems and teams can better conduct intelligent and coordinated responses to incidents.
Doubling down on AI-driven efficiency will support organisations in withstanding whatever demands are thrown at the businesses. A focus on AIOps can strongly boost resilience and save human time for the innovation that drives growth – even during an uncertain economy.