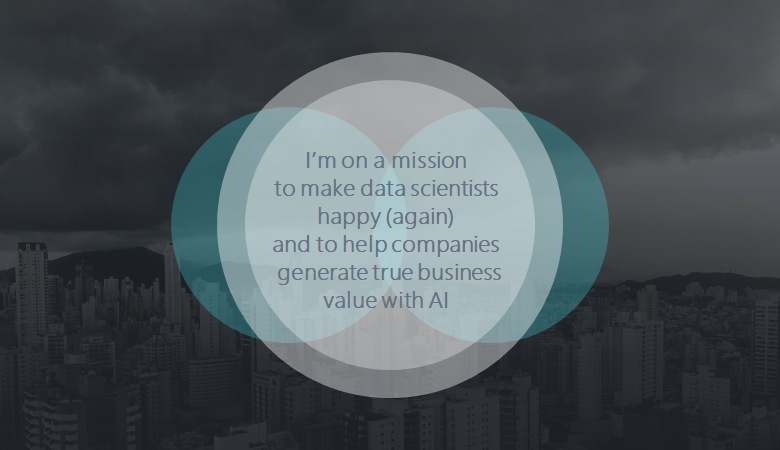
Note: This was written originally written on towards data science, please click the link to read more by the author and to see more articles.
And at the same time to help companies generating true business value with AI… This is a summary of a talk at the Applied Machine Learning Days at the EPFL in Lausanne Switzerland in January 2020. You can find the talk on YouTube as well.
How I got to this mission
Before the year 2018, I was an unhappy data scientist myself. I was working on projects that were not generating true business value. In my team, we missed the right skills and tools for doing the right things. We didn’t know how to build a pipeline or to build a productive system. And at the same time, there was often no real business interest in what we were doing.
I was frustrated because I prefer to work on topics that have an impact.
I wasn’t the only one in the market with this frustration, and that is when I realized there is an issue at hand.
And the first couple of months that I was working with this issue, I thought that project management for data scientists would be the solution to the problem. End to end how to bring your data project into production.
Whilst speaking to people about this topic, I realised that it is not enough. Here are a few anecdotes from data scientists in the field…

And anecdotes from those who work in AI, but are not practitioners themselves…

This made me think, is there a mismatch between data scientists and the rest of the company?

Collecting Semi-structured evidence
At one point I started to realise it is all about getting the company ready for generating business value with AI.
Yet, there are still some open questions, here are three hypothesis I further explored and collected some initial data around.
Hypothesis number 1
Data Scientists that are unhappy in their job work in a firm that have more trouble generating business value from AI.
Hypothesis number 2
The main reason for companies not generating business value with AI is the lack of AI Literacy on management level.
Hypothesis number 3
To generate true business value from AI, transformation is required on multiple levels in a company.
I used these hypotheses to engage with people and I asked the following four questions.
Question 1
What makes you as a data scientist happy in your job?
Question 2
What are the main reasons your company is able to generate business value with AI?
Question 3
What are the main reasons you are not happy in your job?
Question 4
Can you tell me why your company is not able to generate value with AI?
Results
The happy data scientist has freedom in their job.
They work on projects where they can solve problems. And they work on interdisciplinary projects across the company.
Is this a surprise?
For me it is not, the first two points are the things that data scientists are often accused of. Perhaps it is just important to land the right problem on the table of the data scientist?

The reasons that are listed for companies to be successful with AI is, first of all, having a clear business value of the projects that are worked on, so they are working towards a goal.
If you ask yourself that right now this should be clear?
Well, I have seen multiple projects start because they were cool or simply interesting.
Management support is very important, working towards a purpose on the one side and another side also the required attention and ability to provide the right resources for the project. Without management support, it will be difficult to get the resources required to build a fully productive system.
Companies are not able to generate value with AI due to missing skills and resources. The skills on the one side to make things work end to end. And on the other side the resources, meaning the budget, technology but also the time of the different stakeholders such as IT or the business.
If there is no end to end commitment, so basically to move things forward after a proof of concept, then it will be very difficult to get actual value for the end-user and to generate a bottom-line impact.
What also was mentioned often was the understanding of the counterparts on the one side what AI or machine learning is.
More often than once people are requested to do ad-hoc reporting or build shiny visualizations. And at the same time, I felt the frustration that people don’t understand what it takes to “do AI” and that a prototype cannot go live tomorrow. This lack of literacy actually results in wrong expectations which then again will lead to frustration on both sides.
What we also see is that unhappy data scientists are unhappy for the same reasons that companies are not successful. It is literacy, the commitment of the management, and the availability of the right resources.
Revisiting the Hypothesis
With this newly gained information. Let’s revisit the hypothesis.
Hypothesis number 1
Data Scientists that are unhappy in their job work in a firm that have more trouble generating business value from AI.
This is true on the one side, but actually there are happy data scientists working in companies that do not generate business value yet.
When we focus on what makes companies successful with AI; we work at the same time on those things that make data scientists unhappy. This means we have one problem to solve.
Hypothesis number 2
The main reason for companies not generating business value with AI is the lack of AI Literacy on management level.
I am not convinced yet this is the main reason. It could be that when management is AI Literate, they would be able to provide the commitment, and the missing skills and resources. But is that enough?
Hypothesis number 3
To generate true business value from AI, transformation is required on multiple levels in a company.
We need AI transformation.
Yes! This is something I am convinced of. The issues will not be solved overnight, and definitely it is not enough to hire just data scientists.
I have yet to find a person who has the time and skills to build a solution that works within larger corporations. And at the same time deliver what the business truly needs.
When we speak about transformation, we can think of our operating model. We need to include the necessary parts to prepare the company and data scientists.
- This is about a vision and strategy, what kind of company do you want to be when it comes to AI and how will you get there?
- What technology will you need for that, and will you buy or build? What does it mean for your data strategy and structure?
- What people will you need, how will you recruit and develop them?
- What processes do you need to put in place to make sure your projects run smoothly?
- Change Management. How will the rest of the company be prepared for what is coming?
- Are the things that you do compliant with regulations such as GDPR, and are you doing ethical things?
- And lastly, preparing the governance around all of this to make it work.
I am convinced by now that all of these topics are relevant for AI to work in real life.
In Summary

We can put what we have learned on a timeline. We start with this one data scientist working with the leadership in a company (1). The data scientist is unhappy, until the leadership sees the light, and becomes AI literate (2). They will put an action in place to get everything necessary mentioned before sorted (3). And then we will be able to make happy data scientists (4).
If there is one part you’d like to take away or pass on from this article. Let it be this…
“Leaders in companies that want to use AI to the benefit of their organization should educate themselves in AI and become AI literate. This means not only to understand the possible applications but also what it takes to bring AI to success in their organization.
“Once they do, they will be able to provide the commitment beyond POC it takes and provide the resources and skills it requires to generate true business value with AI.
“And finally our data scientists become happy again.”
Of course, this is not where it ends. You can keep updated on my journey by signing up for my newsletter.