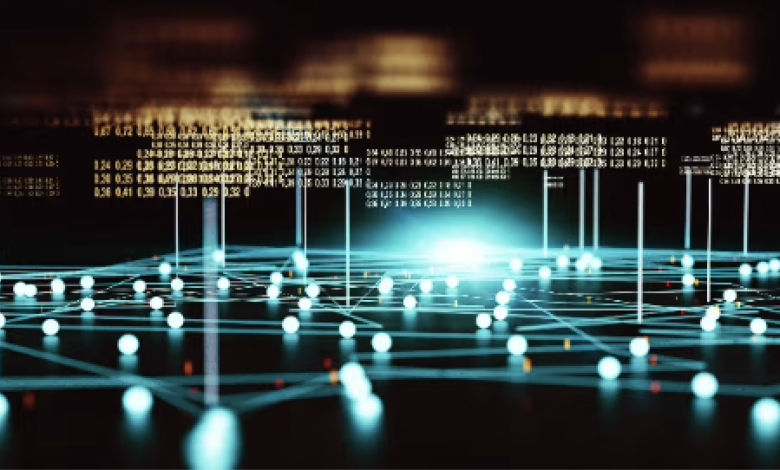
When it comes to cutting-edge AI technologies, the spotlight often shines the brightest on Generative AI (GenAI) because it is the most widespread set of AI tools used today in the public domain (i.e. content or image generation). However, the race to more powerful and larger GenAI models started to raise concerns about the energy needed to train them and apply them in daily operations.
In fact, this may represent one of the critical intersections that executives face today: if we want to use AI responsibly, how to make sure that we use the most appropriate AI tool, considering not only the quality and precision of the outcome but also the compute cost and the associated carbon impact?
The AI trap executives fall into
As the first ever Chief Artificial Intelligence Officer (CAIO) at Schneider Electric, together with my team, we help today’s decision makers understand which AI technology suits best to their needs and ensures the most responsible, and ethical use of the technology.
Our goal is to help businesses to ensure they don’t systematically fall in the trap of using the newest tool, just because it’s the most recent and shiny one. Instead, they should select solutions crafted for their needs, maximizing the output and minimizing the associated computing, energy and carbon impact. Considering the dire energy and climate crisis we find ourselves in, companies must assess different scenarios and choose the most appropriate AI tool.
One of the biggest mistakes executives make is to try to apply AI everywhere without focusing on real-life problems and business value. Making many pilots projects that doesn’t deliver more that an experimentation opportunity, may contribute to unnecessary energy usage and increased carbon footprint.
When to Choose AI
When thinking about implementing AI-enabled solutions, the first thing you must consider is something quite simple yet often overlooked – how they contribute to the company mission and strategy.
Let’s look at an example of how we measured and assessed AI use cases before GenAI entered the mainstream. First, we initiated a meticulous review process involving internal and external stakeholders where we examined over 200 potential GenAI applications. Those aligning with our responsible AI framework, while offering substantial customer value, were greenlit. The resulting applications, now in operation, are either enhancing internal productivity or directly advancing the core mission of fostering sustainability.
Now let’s dig into some examples of the applications that were put into motion and the direct impact they had.
1. Using AI to transform buildings into more sustainable facilities, starting with our homes.
An example of when AI is the right choice is when it comes to empowering homeowners to reduce the two of the biggest power loads in the home – water heaters and electric vehicle chargers. By enrolling high consumption equipment into an energy management solution, homeowners can greatly reduce electricity costs without compromising on their comfort or having to constantly monitor energy prices. It works by processing real-time weather forecasts, variable tariffs, solar production data and user preferences in its predictive algorithm that proposes an optimized plan of energy management. The Al then creates smart schedules for enrolled devices and limits the usage of these devices when electricity prices are high, while following user’s habits.
The result? In lab testing, homeowners with solar panels saw predicted annual savings of between $450-550, and homes without solar panels saw savings between $100-160. While actual savings may differ from estimated savings depending on home installation and energy provider, it is clear that there are some real tangible savings on the table.
2. Using AI to optimize electro-intensive processes.
The cleanest energy is the energy we don’t use, and through advanced AI algorithms, companies can reduce energy consumption and carbon emissions by optimizing energy-intensive processes such as HVAC systems in buildings, water desalination, district heating, and more.
Therefore, creating a decline in energy usage has a direct impact on lower carbon emissions. For instance, a district heating utility in Croatia that serves over eight thousand citizens, applies a district energy system for hydraulic modeling with an AI Load Forecaster module. Thanks to AI, they can forecast district heating needs and better optimize the amount of energy needed to heat the district.
It allows the utility to implement energy-saving policies and optimize the operations of chillers, boilers, and energy storage systems. As a result, both operational and maintenance costs, and carbon emissions can be reduced.
What about Machine Learning and Deep Learning?
Beyond GenAI, other AI applications can contribute significantly to Environmental, Social, and Governance (ESG) commitments. More traditional Machine Learning algorithms (ML), for instance, will likely be one of the biggest tools in our toolbox to aid us in the renewable energy transition. By delving into large datasets, ML uncovers patterns, forecasts demand, and optimizes energy consumption. As a result, we can better manage the shift from fossil fuels towards sustainable energy sources.
Especially Deep Learning (DL) algorithms, a subset of ML, prove to be useful in domains like demand response optimization and renewable energy forecasting. Notably, DL’s application in Smart Energy Management Systems (SEMS) catalyzes energy conservation in early building deployment phases. Through innovative techniques like Cold Start method, described in our recent AI at Scale podcast, DL empowers accurate energy consumption forecasts, curbing waste from inception and enhancing environmental stewardship.
Analytical AI like ML, and specifically DL, with their lower computational and energy demands, are robust alternatives to resource-intensive GenAI solutions. And all these examples are just scratching the surface of how AI can help us better optimize energy usage.
Moving Forward
To ensure each AI project or solution you deploy is the right decision, you must be critical in analyzing if, and how, it serves a meaningful purpose. By meticulously evaluating and balancing the energy demands of AI implementations with how they could reduce energy consumption, organizations can chart a course towards responsible, more sustainable innovation. Ultimately, this thoughtful approach not only drives efficiency but also positions organizations to lead in both technological advancement and environmental stewardship, ensuring AI is put into practice where it has the biggest and best impact.