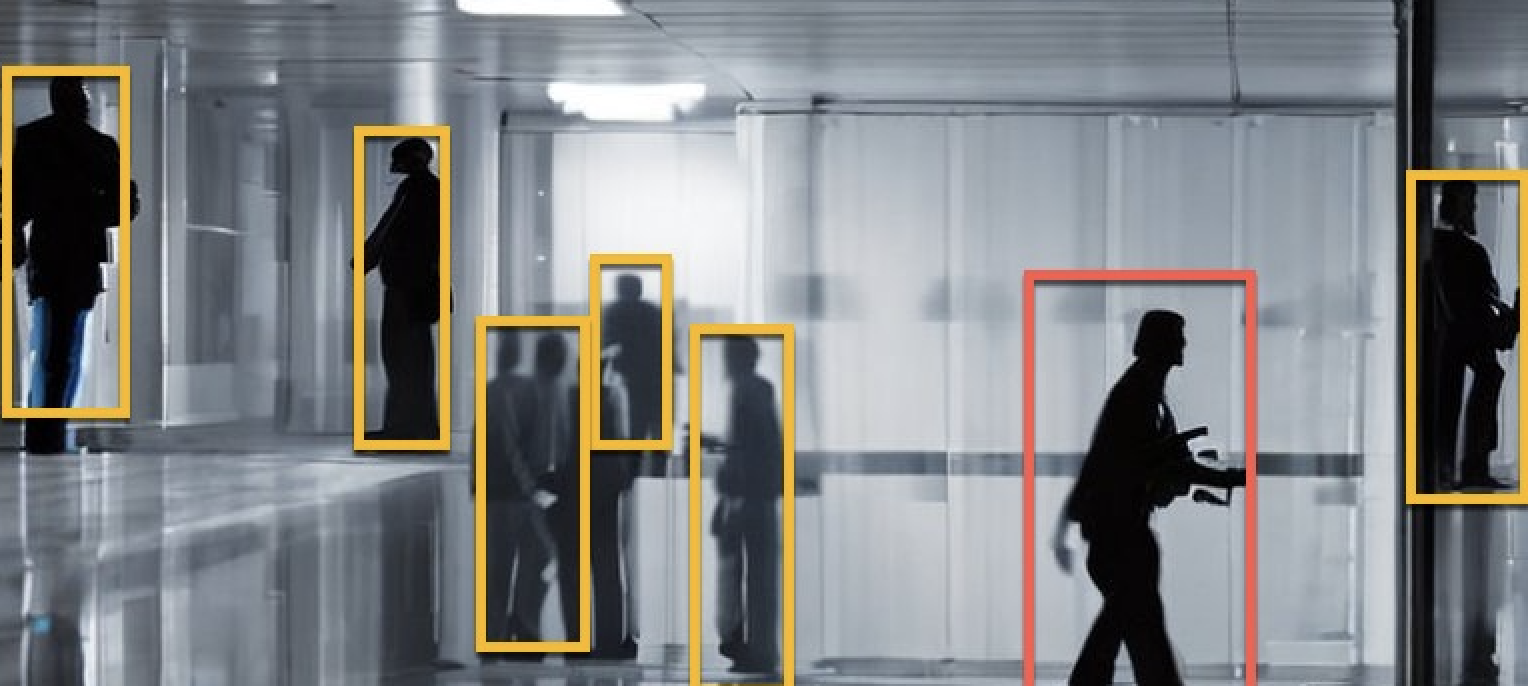
While artificial intelligence (AI) is being eagerly adopted in many sectors, mistrust of this nascent technology is growing. Much of this distrust comes from suspicion generated by claims that AI can emulate human intelligence, especially when associated with the recent onslaught of generative AI applications. Fears of fake news, fake people, and other fake forms of information conveyance abound. But there are many other types of AI applications whereby reliability and accuracy are essential, which revolves around the specific application and how its AI models are developed and deployed. Take edge AI applications, for example, as these are often deployed in mission critical environments where accuracy and reliability could be a matter of life or death.
In business environments, key stakeholders are often wary of claims about the accuracy of AI decision making. In 2019, a Deloitte Survey found that 67% of executives were uncomfortable using and accessing data from advanced analytic systems like AI. Unfortunately, that kind of mistrust can stop businesses from taking advantage of the substantial commercial value offered by an AI system. Without that crucial element of trust, AI projects might not get the necessary funding or organizational support, and existing AI applications could be mothballed.
Building trust in AI projects and systems requires transparency about how AI models make decisions; how accurate those decisions are; and what can be done to maximize their accuracy. Setting realistic expectations around accuracy will help establish the trust that AI projects need to move forward.
Even in mission-critical applications, don’t expect 100% accuracy
The first thing that must be understood is that AI technology is fundamentally based on probability. In most cases, 100% accuracy is a practical impossibility. Ask ChatGPT multiple times to write a song about how to write a song, and the words will never come out the same. Or an edge AI application like airport surveillance can make a pretty good guess on the identity of a person, but it might only be 99.4% certain. Most use cases will never need 100% accuracy, so not only must stakeholders comprehend this to manage their expectations, but studies also show that being clear about accuracy levels will improve trust in AI projects.
A 2019 Microsoft Research paper found that showing people the realistic accuracy of a machine learning model can help increase their trust in its predictions. The paper’s authors note, “These results highlight the need for designers of machine learning (ML) systems to clearly and responsibly communicate their expectations about model [accuracy], as this information shapes the extent to which people trust a model.”
AI may be only as accurate as the training data
The success of AI models in making accurate decisions depends upon the quality of the data that they’re built on. This is why one of the most important factors when developing an AI model is to think about how to collect the training data. Commercially available datasets exist for training AI models, but some find it more advantageous to use their own proprietary data, or at least use commercially available datasets to supplement their existing data. However, data quality has several implications. In the case of generative AI applications based on foundation models, the quality of data could be good, bad, or ugly. In the case of edge AI applications that focus on specific tasks, the quality of data must be more tightly controlled, i.e., labelled correctly to eliminate or reduce bias relevant to the specific task.
Accuracy may require time for improvement
Although the accuracy of a particular AI application might not be perfect from day one, what makes AI such a ground-breaking technology is its ability to learn. As it collects more data in a live setting and faces potentially unexpected situations or different perspectives, developers can use that data to retrain their models and improve accuracy over time.
Data augmentation, another process to increase the quality and quantity of data for model training, works by generating new data points from existing data. And generative AI can also be used to generate synthetic data, which is especially useful in situations where collecting a suitable amount of data is difficult.
The accuracy of an AI application can also be improved by replacing the original model with a more advanced one. The state-of-the-art for AI models is constantly changing, with improvements in both computational performance and accuracy. Although training a new model from scratch can be a monumental and expensive task, the result can be highly advantageous.
Prepare for the unexpected
One of the fundamental qualities of an AI system is its ability to change. It will be sensitive to environmental changes (e.g., weather, dirty camera lens) and thus organizations must prepare for the unexpected as they develop their models and most of all, as they field deploy. This is especially true when going from testing to live deployments, where organizations could experience large drops in accuracy within their AI systems.
AI won’t replace human decision making
While AI technology shows great potential in emulating and sometimes exceeding human intelligence, it is unlikely to take over the ultimate decision-making process in most cases. Rather, it is most likely to serve a more advisory role. Take, for example, an AI model that is meant to spot tumours. While the model might be able to spot tumours better and faster, doctors will likely still retain the diagnosis job. This is important because AI models won’t have the doctor’s domain knowledge and AI systems can and do make mistakes. (So do doctors!) Basing final decisions on human expertise is often an intuitively and legally better option.
Trust requires transparency
AI technology is rapidly transforming the world and a degree of mistrust of the new and unknown is understandable. To create that trust within an organization, AI projects must set realistic goals and expectations about what can be achieved. Transparency about these issues isn’t just about limiting expectations, it’s also about engaging stakeholders and enabling them to understand the tangible benefits that AI projects can deliver. A 2021 review of studies around AI trust from the University of Queensland noted that businesses “…will only trust AI-enabled systems if they can fully understand how AI makes decisions and predictions.” However, this could present a real challenge. We don’t even fully understand how even the simplest AI models make decisions. Quite often, it’s not based on science, but purely trial and error. This situation is further exacerbated by large generative AI models, some of which contain hundreds of billions of parameters.
Whether or not organizations currently recognize the importance of trust around accuracy, they may soon be compelled to. In April 2021, the European Parliament released proposals about AI regulation in Europe. These proposals state that AI projects must clearly document expected accuracy – and the metrics by which they measure accuracy. Failure to do so, the proposal states, could result in fines of up to 10 million Euros or 2 percent of the developer’s global turnover – whichever is higher.