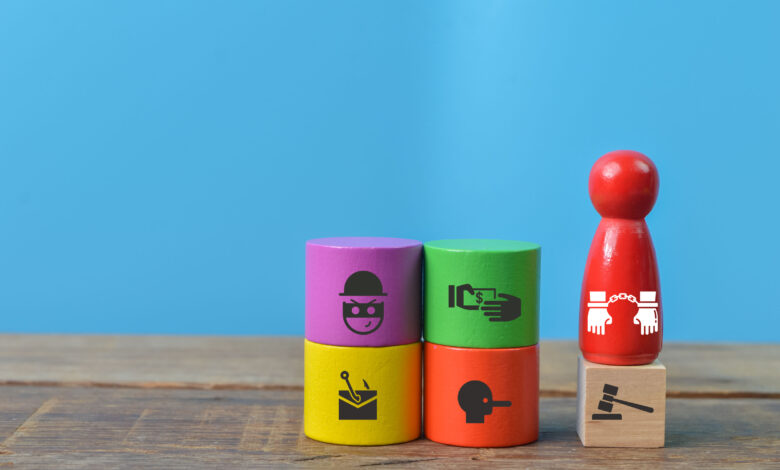
Staying one step ahead of money launderers has long been a headache for compliance teams. Even for highly qualified data analysts working with advanced machine learning systems, the challenge of identifying complex illegal transactions that are designed to blend with legal ones, is considerable. To detect illicit financial flows and patterns in a tsunami of incoming data, with limited human resources, makes countering the increasingly sophisticated methods employed by criminals all the harder.
It doesn’t help that the two main machine learning models currently in play in the compliance landscape – supervised and unsupervised – each have their own downsides.
Unsupervised models, commonly used for detecting known and unknown anomalous patterns, although speedy, return considerable volumes of false positives, soaking up hours of valuable staff time as teams must wade through vast amounts of data to identify those transactions that may be suspicious.
Equally, supervised models present problems from the outset, since they require labelled transaction data to train from – but this is notoriously difficult to gather for reasons of data privacy and client reluctance – making them slow and resource-intensive to populate.
However, these downsides are now being counterbalanced as progressive software innovators continually endeavour to build solutions that solve such compliance challenges while being scalable, adaptable and update-able enough to outpace the changing behaviours of launderers.
Marcus Markland-Montgomery, a Data Scientist at Napier, which is a provider of advanced, AI-led financial crime risk management and intelligent compliance tools, together with a team of academics at Politecnico di Milano, the largest technical university in Italy, set out to distil the best qualities that supervised and unsupervised machine learning models can offer.
Dubbed ‘Amaretto’, the resulting model is an active-learning model that tackles transaction monitoring in a manner as assumption-free as unsupervised approaches yet needs minimal data points to deliver an effective supervised system. The longer that active learning model operates, the better it gets at identifying anomalous transactions, capturing changes in behaviour in real time, and delivering an effective “analyst-in-the-loop” risk detection framework.
The project’s academic paper, published earlier this year by the Institute of Electrical and Electronics Engineers (IEEE) after detailed peer review, proved that Amaretto outperforms state-of-the-art solutions, improves the True Positive Rate (TPR) by up to 50%, and reduces the overall compliance cost by 20% in the most realistic scenario the team looked at. The decision to make this academic paper free to access was mutually agreed upon by Napier and Politecnico di Milano, true to the team’s collective desire to push the field of machine learning forward.
Synthetic data generated to simulate the profiles of clients trading in international capital markets was used to test AI selection strategies to identify the most appropriate subset of transactions for investigation. Thereafter, the team’s research focused on how to make the most efficient use of the feedback provided by human analysts, to ensure that the system uses such feedback intelligently in the future.
Amaretto pre-processes raw transactional data, converting it to aggregated vectors. The unsupervised and supervised models take the vectors as input and compute an anomaly score for each one, from which samples are selected by the system for analyst review. Finally, the labels assigned to these samples by the analyst are used to train the supervised model which computes the final risk score.
Using Isolation Forest as its unsupervised system, the Amaretto prototype outperforms other systems by 20%, while the supervised set, using Random Forest, delivers a 30% improvement in performance. These uplifts were seen when the team performed the entire experimental evaluation using multiple approaches on the same dataset.
“Amaretto works more effectively over time, as more analysis is fed back into it. It needs remarkably few data points at the outset, thus overcoming the issue of client resistance in providing information, and it uses every piece of analysis to improve performance,” says Markland-Montgomery.
“And while supervised models are effective in identifying historical money laundering methods, unsupervised approaches quickly pick up new gambits, so it is designed to outpace even the most innovative money laundering techniques.”
Amaretto delivers lower investigation costs because of the smaller number of daily transactions to be examined, and fewer false positives and false negatives. In a comparison with a state-of-the-art active learning fraud detection system, Amaretto achieved better detection performances and lower costs in all the analysed scenarios.
Markland-Montgomery is currently working on Napier’s next-generation machine learning solutions, which cross-reference data for unstructured text screening, helping compliance teams to quickly identify sanctioned individuals as the beneficiaries of funds, even when these instructions are hidden in paragraphs of text.
And while for the foreseeable future compliance departments will certainly require a balance between machine learning and human analysis, Markland-Montgomery anticipates advances in the way humans and machines interact. Adding explainability to the systems, thereby making the rationale for the AI selection and analysis of datasets more accessible to everyday users, will again reduce costs and improve compliance performance.