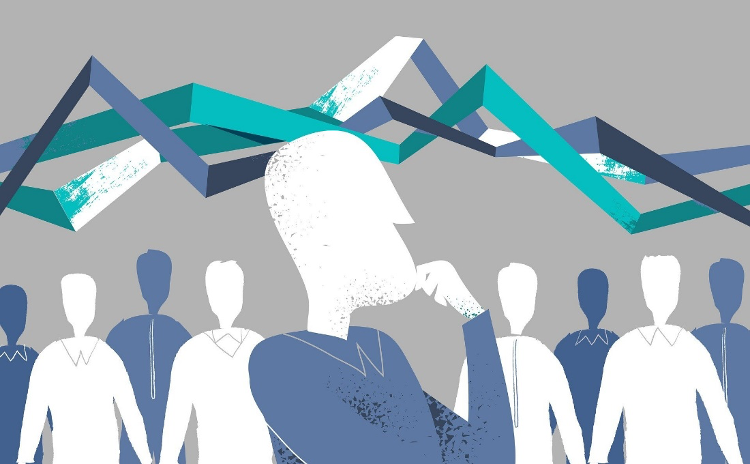
Market research has used statistical methods to analyse people’s opinions for many decades. These long-lived data sets and research methodologies have made the industry reluctant to change, as consistency is vital to the proposition. However, market research must change as people’s lives and behaviours change: becoming more digital, more spontaneous and always-on, more glued to mobile phones, and competing for attention with social media and mobile games. The pandemic has only accelerated these disruptive trends as face-to-face contact became impossible and people’s day-to-day behaviour drastically changed.
Building AI models is one way of rapidly adjusting to this new world, as they can address the collection, verification and analysis of digital data. In the same way that advertising became AdTech, industry leaders in market research are becoming ResTech. There is an explosion of innovation in the space to enable organisations to hear their stakeholders’ views more clearly, more often and with greater speed.
Using AI to connect the right audience with the right survey at the right time
With people always on or attached to their phones, the collection of data through mobile-friendly surveys is increasing rapidly. The challenge is to reach out to the right people with relevant questions at the right time. Just as in the online advertising industry, AI models are used to determine the best match between people and surveys and the most favourable time to reach out for their opinions.
Some people like to give answers in their coffee break, some on their commute and some later at night. This diversity of preference can be tamed using ML-trained recommendation models that offer an individual experience when the audience is most available and receptive. Matching pet owners for a survey is different from matching car owners; with limited data about a person, AI models can predict a person’s likely profile against exacting survey specifications without asking endless questions. Even with incomplete data, this precise targeting gives a better user experience and, therefore, better answers to questions.
Verification of user data is essential when collecting information online. Survey respondents often earn rewards for their time and attention, which inevitably can lead to bad behaviour. With increasingly sophisticated attackers, deciding if a person is ‘good’ or ‘bad’ has become impossible without an AI-based behavioural model. Only an AI-based model can detect the subtle signs of a purely economically driven actor in real-time and with vast numbers of people.
Understanding people’s preferences are often challenging when they may not have a conscious reason for those preferences. Historically, most surveys have asked people to explain why they behave or think a certain way on a scale of 1-10. Often people’s opinions are not self-consistent or strong enough for these types of questions to give real insight. Therefore, the most advanced surveys use AI models to answer these questions directly – based on ranking and choice preferences.
How might this work in practice?
In one such survey, people were asked to choose between different combinations of colour and design of sports shoes. By picking preferences from a limited set of options at each stage, people help create a data set used to train an AI model to predict sports shoe preferences for each age group. The AI design model can then be used to predict the popularity of any design presented to it. In this way, entire generations of sports shoes are created without having to test each specific combination. The preference model is updated easily by repeating the survey process with a new training data set for people to select preferences.
Significantly, the model doesn’t need to know why people preferred one choice to another or having a precise but arbitrary score for each criterion. The time taken by the user to make each decision and the areas where they zoomed in to check the details are all crucial training data that would have been impossible to gather in the past. This simplicity coupled with good survey flow and design appeals to people, truly engaging them in the task.
Deepening insight with facial sentiment analysis
Webcams are being used to analyse facial expressions during a survey. The encoded video data does not rely on the person actively answering questions. Reactions to videos, images, or text can be analysed using facial expression models. The impact of an advertisement can be measured by looking at the respondent’s micro-expressions while watching the advert. The change of expression can show how the user is engaging with the content while tracking eye movement and blinking can show if the user is focusing on the content.
This process then replaces the traditional method of showing an advert and asking individual questions how you felt around each part of the advert. It reduces the human resources needed to process the data and unnecessary bias. It can rapidly process a large pool of samples to evaluate if an advert has pleased its intended audience or if it has rough edges that could damage a brand’s reputation and hurt customer feelings.
Summary
Machine learning algorithms are being used in all parts of the Market Research industry in response to people’s changing behaviour and the desire for more insightful data. Those companies embracing the AI revolution are already disrupting the industry, allowing more and better insights at speed and with reduced cost.