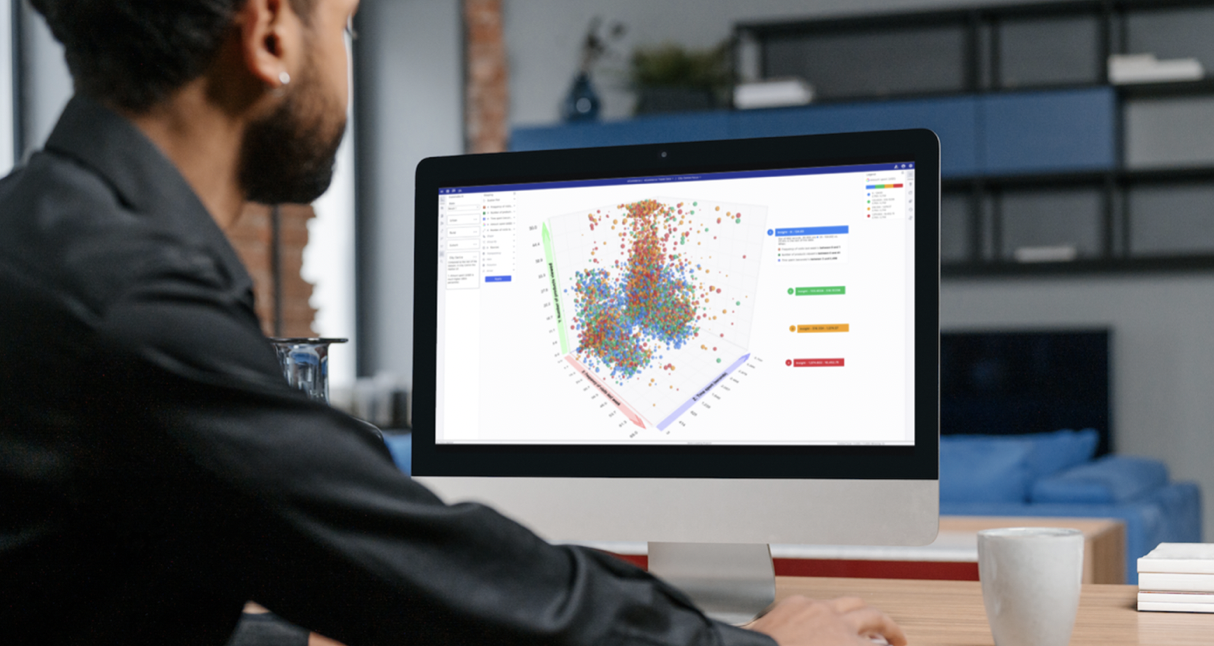
Tools like ChatGPT and Bard are called large language models (LLMs) for a reason. These generative AI applications deliver answers to conversational questions in the text. Intelligent exploration, on the other hand, can make sense of text, numerical information, proprietary data, and lots of other multidimensional data visually.
Pairing these two AI technologies together will result in major advances in analytics. Data analysts will soon be able to seek and deliver answers from huge amounts of data using everyday language. The outputs—visualizations, reports, recommendations—will become more understandable to decision-makers who don’t have a background in data science or data analytics. And this will make taking action on findings easier and faster.
Let’s look at an example from a fictional financial services company to see how conversational AI and AI-driven intelligent exploration will work in tandem to figure out how to best market a new product.
How Financial Services Companies Can Benefit from AI-Driven Strategies
A robust AI solution can help businesses figure out new offerings, which prospective customers to target, what marketing and ad language to use, and when and where to push these messages.
In the financial services sector, investment advisors can analyze customers’ financial profiles, investment histories, goals, and risk tolerance to personalize recommendations such as socially responsible or sector-specific investments.
Life insurance companies can define policies that are tailored to customers’ specific health conditions, lifestyles, and needs, with pricing based on accurate risk.
Or, imagine that a bank wants to assess how to best market a new credit card targeting frequent travelers, determining the offers that will be most compelling. An AI platform capable of ingesting heterogeneous data types can accommodate analysis of demographics, transaction history, browsing behavior, past interactions with the company, sentiment from chatbot conversations, email messages, and social media interactions. Then, analysts could leverage generative AI to ask questions about prospective customers and find answers in the data in plain language.
Questions could be very specific, like, “Would it be better to focus offers on travel insurance features or low foreign transaction fees?” Or they could be more open ended: “What marketing offers will bring in the most revenue?” The latter approach, getting AI’s help to identify priority areas to further explore, offers the advantage of avoiding the introduction of biases that can lead an analysis down a false or skewed path.
Intelligent data exploration uses embedded AI routines to arrive at answers to these questions. Findings can be produced in a Knowledge Graph or a 3D network graph, that can be interactively visualized, analyzed, and explored.
A data analyst could sit with a product marketer and ask the system, “Tell me what customers would be most likely to purchase a frequent traveler card.” Intelligent exploration engines produce intuitive visualizations that group customers into segments based on their interests and behaviors, connections to each other and lots of other parameters. Examples of groupings for this query might be business travelers, those who have searched on credit card comparison sites or an airline ticket aggregator site, or people with a big birthday or anniversary coming up.
Analysts could ask the AI solution, “Which prospective customers are due for travel?” to identify a niche audience. They could get a list of sample marketing messages based on LLMs’ knowledge of billions of web pages, code and books, and hundreds of billions of parameters. AI systems with LLM and intelligent exploration capabilities could automate a lot of the work of translating complex analyses into concise findings, while also providing transparency into the recommendations the LLM makes. It’s only going to become more important for AI platforms to “show their work,” something currently lacking in ChatGPT and other LLM tools. Explaining how conclusions are reached is crucial for increasing trust in AI models and may eventually be required for regulatory compliance.
Already, intelligent exploration allows users to quickly produce engaging reports, complete with graphics and written in simple language. Couple that with generative AI, and it’s going to become easier and faster for more people throughout a business to understand findings, assess caveats, and determine the best course of action.
Intelligent exploration and generative AI bring data into focus
This approach avoids one of the biggest pitfalls of using data in decision-making: narrowing the scope of inquiries in the early stages. One reason AI is such a game changer for data analytics is the ability to look at the full universe of information that’s available. It’s like being able to look at the full vault of a starry sky, getting guided to the patch that’s most important, then zooming in to find new discoveries.
So many organizations are sitting on a wealth of data they can’t make sense of. They may have a shortage of people qualified to sort through it. Or their leadership finds it hard to trust AI findings because conclusions aren’t backed up by explainable AI. They might lack an AI engine powerful enough to ingest all the relevant types of data, from sales reports to PowerPoints to webinar transcripts to spreadsheets.
Using generative AI with intelligent exploration will help companies move beyond these challenges. These AI technologies use natural language and visuals to tell the full story hiding in data. They are poised to automate much of the work of data analysis, so analysts can do more.