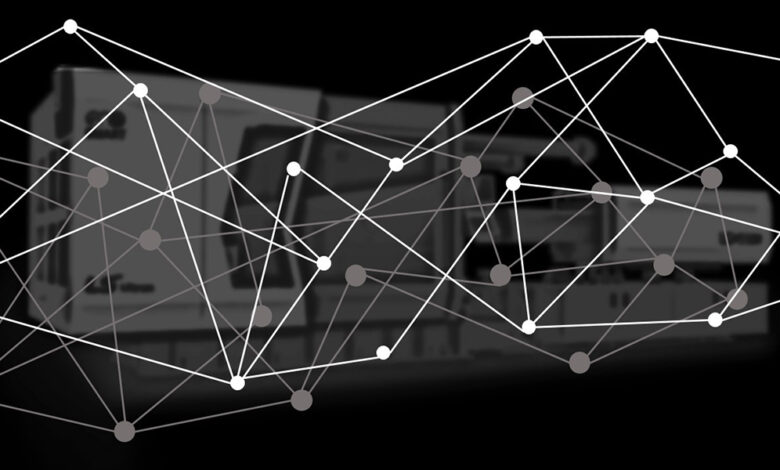
Injection molding is a process of making parts in large volume through the use of injected molds, and it is set to be revolutionized through the incorporation of artificial intelligence.
Among the many processes with significance in the contemporary manufacturing world, injection molding emerges as a vital step towards the production of numerous plastic parts. They vary from automotive products to electronics including steering wheels, brake pedals, seat components, home appliances, computers and gadgets, car steering wheels, car pedals, aircraft interior and exterior trims, car dashboard, car mirrors, furniture and fittings among others. Namely, at Kemal Manufacturing, which is a prominent company dealing with precision injection molding, the adaptation of AI to this process is changing the general approach to the design and manufacturing of components as well as their delivery. Kemal Precision Manufacturing possesses the skill, knowledge, and experience regarding the provision of injection molding services and making the whole whole process convenient.
Technological Exposition
This avant-garde AI technology pertinent to the injection molding procedure is delineated by two principal operational phases: the training phase (antecedent to production) and the inverse analysis phase (concurrent with production) (refer to Fig. 2). The training phase meticulously constructs a model of unparalleled precision, forecasting the caliber of the molded components derived from the attributes of recycled materials and molding parameters. This is achieved through an automated selection of an optimal algorithm from a plethora of machine learning algorithms, as delineated in the subsequent training database. Conversely, the inverse analysis phase extrapolates the ideal molding conditions from the predictive quality paradigm of the molded constituents and the attributes of the recycled material. This is accomplished by employing an inverse analytical approach to model optimization.
Fig. 2. Automated Optimization AI for Plastic Molding Processes
Configuring an Advanced Training Database for Predictive Modeling of Molded Parts Quality
Crafting an optimal training database is paramount for the generation of an exceptionally precise predictive model regarding the quality of molded parts. This endeavor necessitates an elevated comprehension of the molding process control. Kemal Precision Manufacturing has pioneered a technology to structure the training database such that it elucidates the interplay between the conditions of the plastic molding process, the resultant quality of the molded parts (weight), and the attributes of recycled materials.
The training database is bifurcated into a process database and a feature database, as illustrated in Fig. 3. Initially, the process database employs a design for experiments methodology, leveraging expert insights into molding processes. This involves molding parts while systematically varying molding conditions and batches of recycled material, subsequently inputting data that links these conditions to the quality of the molded parts. Thereafter, the feature database isolates and inputs characteristics correlated with the properties of recycled materials, based on pressure, temperature, and an array of sensor data gathered during molding under identical conditions for each batch of recycled material.
Kemal Precision Manufacturing has achieved a breakthrough by extracting relevant features from sensor data to optimize settings for inter-batch variability. This was accomplished through the application of extensive knowledge accrued in injection molding research and development, including technologies aimed at correcting plastic molding errors.
In essence, the configuration of this sophisticated training database marks a significant advancement in the realm of predictive modeling for molded parts quality, embodying a meticulous synthesis of experimental design and sensor-based feature extraction.
Fig. 3. Techhology to Configure the Training Database to Generate the Molding Quality Predictice Model.
AI-Powered Design Optimization
AI brings unparalleled efficiency to the design phase of injection molding. Through advanced algorithms and machine learning, AI can analyze complex design rules, material properties, and manufacturing constraints. This allows for the rapid iteration of designs, optimizing for factors such as material flow, cooling rates, and stress distribution. The result is a more efficient and effective design process that minimizes defects and maximizes performance.
Predictive Maintenance and Quality Assurance
One of the paramount advantages of artificial intelligence in injection molding is its capacity to foresee and avert equipment malfunctions. By perpetually scrutinizing the performance of injection molding apparatuses and dissecting data from a plethora of sensors, AI systems can discern patterns that signify potential issues. This predictive maintenance methodology ensures machines function at peak efficiency, diminishing downtime and curbing maintenance expenditures.
In the realm of quality assurance, AI can scrutinize products instantaneously, pinpointing defects imperceptible to the human eye. This guarantees that each component adheres to stringent quality benchmarks, thereby minimizing waste and enhancing overall product dependability.
Process Automation and Optimization
AI-fueled automation is revolutionizing the injection molding paradigm. Intelligent systems can orchestrate the entire production cycle, encompassing material handling, mold closure, and injection. By optimizing these processes in real-time, AI ensures consistent quality while truncating cycle times. This echelon of automation also fosters augmented flexibility, permitting manufacturers to transition between various products and materials with minimal reconfiguration.
Data-Driven Decision Making
The amalgamation of AI into injection molding endows manufacturers with a plethora of data that can inform decision-making. By analyzing data harvested from the production floor, AI can unveil trends and insights that propel continuous improvement. This data-centric approach empowers manufacturers to refine their processes, abate costs, and elevate product quality.
Enhancing Material Efficiency
AI also assumes a pivotal role in optimizing material usage within injection molding. By analyzing material flow and foreseeing potential issues such as air traps or weld lines, AI systems can recommend adjustments that curtail waste. This not only bolsters the sustainability of the manufacturing process but also diminishes costs associated with material wastage.
Customization and Flexibility
In an era where bespoke solutions are becoming ever more pivotal, AI furnishes the tools requisite for efficaciously addressing these demands. AI-infused systems possess the remarkable ability to swiftly recalibrate, producing limited runs of bespoke components without compromising quality or escalating expenditures. This adaptability proves particularly invaluable in sectors such as automotive and consumer electronics, where product variations abound.
Prospective Vision
The assimilation of artificial intelligence within our injection molding paradigms propels us towards unparalleled precision and efficacy. This avant-garde technology transcends mere enhancement of our extant competencies, instead establishing a pioneering standard for the industry.
FAQs
Q1: How does AI ameliorate the design process in injection molding?
AI optimizes design by scrutinizing intricate rules and constraints, facilitating rapid iterations and enhanced performance.
Q2: What function does AI serve in predictive maintenance?
AI monitors machine performance, discerning patterns that prognosticate failures, thereby reducing downtime and maintenance costs.
Q3: How does artificial intelligence elevate quality control in injection molding? Artificial intelligence conducts real-time inspections, identifying imperfections imperceptible to humans, ensuring rigorous quality benchmarks.
Q4: Can artificial intelligence aid in minimizing material wastage in injection molding? Certainly, artificial intelligence optimizes material flow and anticipates challenges, recommending modifications to curtail waste and lower expenses.
Q5: How does artificial intelligence contribute to personalized manufacturing in injection molding? Artificial intelligence facilitates the efficient production of small, tailored batches of components, enhancing adaptability while upholding excellence.
Balla