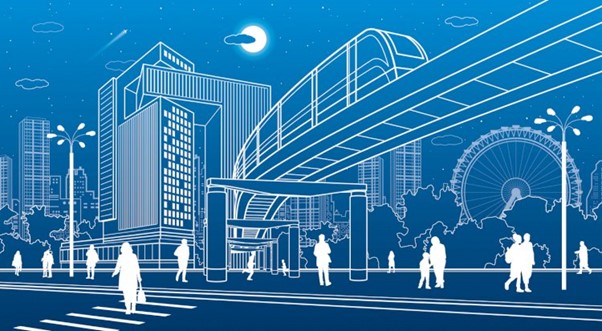
For many of us, the concept of AI is one that is strongly connected with our digital operations, office work, or virtual presence. What we do not typically associate with AI is the mundane route to work on our morning commute, the time we have to wait for the traffic lights to show a green man so we can cross the road, or the constant supply of our favourite brands at our local supermarket.
Yet these small details which make up our everyday urban experiences in the physical world are just as much AI-powered systems as the chatbots or augmented reality gaming experiences that we might interact with in the virtual world.
Whether we like it or not, AI is becoming increasing fundamental to the basic fabric of everyday urban life. In fact, it has already been underpinning many background city operations, such as waste removal, energy distribution, and traffic management, for several years.
As humans across the globe fight for the preservation of current ecosystems and the future of human civilisations, the adoption of AI in urban development offers a beacon of hope for the sustainability of urban life – and this is not just a flimsy notion. Innovative businesses across the globe are demonstrating the tangible benefits of AI in reducing the environmental toll from the heavy demands of urban life, while also making cities cleaner, more accessible, and safer places to live.
In this article, we focus in one particular area of application for AI in urban development: transport management. This is a key-use case which demonstrates the rich benefits of AI technology in urban development for businesses, citizens, and the environment. We also consider the key obstacles to the adoption of AI in transport management, and how the emerging AI trend of personalization could shape the future of urban life more generally.
Why AI is the ultimate traffic warden
Transport management might not seem like the most exciting aspect of urban development compared to the more glamorous areas of city life. Nevertheless, transport management systems are absolutely crucial within the bigger picture of urban development, and, as we explore below, there is quite a bit more to them than meets the eye.
Traffic is widely seen as one of the great downsides of city life, creating pollution, safety hazards, noise, and general frustration. Effective transport management systems are not only crucial in ensuring public safety and convenience, but also play a fundamental role in the daily operations of thousands of businesses, whether they are based in a city or not.
These systems also illustrate how AI is becoming indispensable to modern urban life, quietly improving the operations of the mundane yet essential public services that we typically take for granted on a day-to-day basis.
In this section, we look at four key use-cases for AI in transport management systems: 1) GPS route optimization, 2) smart parking services, 3) smart traffic light systems and 4) obstacle detection. These use-cases illustrate how AI’s application in transport management system is having a tangible impact on daily life in the city, demonstrating the potential of this technology as a positive and transformative force that can be harnessed for the greater good.
GPS route optimization
Ever wondered how useful your GPS really was when it just directed you straight into a long line of traffic on your way back from work? Well, hold onto your seatbelt, because GPS technology is about to get a whole lot more intelligent.
AI is now being used to optimize GPS route recommendations with more accurate, comprehensive, and real-time data, helping vehicles avoid particularly congested routes and areas.
Traditionally, the efficacy of GPS has been restricted due to lack of integration with real-time data, limiting its ability to account for the constantly changing levels of congestion on roads, and other changeable factors affecting car travel such as weather, road closures, and accidents. Today, thanks to more powerful 5G networks, along with more accurate road surveillance systems which are enhanced with computer vision, deep learning, and AI-enabled video analytics, GPS systems are now connected to reliable sources of comprehensive, real-time intelligence about road activity.
Another factor which has reduced the accuracy of traditional GPS route recommendations is the lack of detail in satellite imagery for roads. This has limited the ability of such systems to comprehensively account for various road attributes, i.e. one-way systems, lane count, speed limits, spatial dimensions, and road surface composition.
RoadTagger, an open-source AI model was developed by researchers at MIT and Qatar Computing Research Institute to address this problem. Utilizing two key types of machine learning, Convolutional Neural Networks (CNNs) and Graph Neural Networks (GNNs), the model facilitates the propagation and aggregation of traditionally scattered pieces of information into one comprehensive dataset that can improve the performance of GPS.
Augmented Reality (AR) technology is also being used to enhance GPS by integrating satellite imagery and traditional maps with digital information about a physical location. This allows users to see navigational symbols and landmark features, optimizing ease-of-navigation and providing a more comprehensive field of view.
For logistical operations in particular, the insights provided by AI-optimized GPS can vastly improve efficiency, reducing the amount of fuel, time, and overall cost involved in crucial supply operations.
Take a lorry servicing multiple outlets with supplies, for example. Not only are these vehicles difficult to deftly maneuver in tight city spaces which the drivers may be unfamiliar with, but they are often tied to a tight schedule. This makes it especially critical for these vehicles to find the most efficient route possible. AI-enhanced GPS recommendations are thus an optimal solution to these particular problems, helping to direct these hefty vehicles away from tight, congested areas, ensuring that supplies get to where they are needed at the right time.
Smart parking
Similarly, AI is now being used to provide smart parking services, which collect real-time data to predict congested ahead of time and recommend alternative parking spots. This not only improves the travel experience for drivers and passengers, but also helps to cut down on emissions and energy consumption by reducing the amount of time drivers have their engines on, either waiting for a parking space to free up or driving around trying to find a free space.
Cleverciti, a provider of integrated parking intelligence solutions, utilizes a range of AI technologies including edge processing, data analytics, and computer vision, to direct vehicles to free parking spaces, and also monitor the legitimate use of different parking zones. This
Smart parking systems are becoming increasingly effective as they integrate with more and more data. Today, smart parking systems can utilize not only real-time data to monitor the current availability of parking spaces in urban areas, but also predictive analytics to flag areas of forecasted congestion. This can help people to plan their car-related activities in and around the city more effectively.
Predictive analytics harness the power of machine learning to analyse historical traffic data in order to identify how congested different parking spots are likely to be at different times. But to be optimally effective, they require data from a vast number of sources, including city councils, construction companies, and event organisers. This gives these systems a more holistic dataset of urban activity. It also provides data about upcoming activity taking place in a city, enabling smart parking systems to account for forecasted events such as concerts, parades, conferences, road maintenance, etc.
Smart traffic lights
As well as optimising GPS route-recommendations and enabling smart parking services, AI can also help manage congested areas by adjusting traffic light-timings according to real-time data. This reduces unnecessary wait times, and improves more critical city services such as healthcare and public transport by prioritizing emergency vehicles and public transit.
One of the leading providers for smart traffic light management is NoTraffic. This company utilizes AI-powered sensors and cloud computing to provide an autonomous, easy-to-install, end-to-end hardware solutions for traffic intersections all over the world.
CEO and Co-Founder of NoTraffic, Tal Kriesler, highlights the variety of benefits and opportunities that the virtual management of traffic signalling networks via the cloud brings to both road users, and pioneering companies working in this sector.
“[Our] sensors can analyze the vicinity of intersections at a human-eye level, detecting all types of road users in various weather and lighting conditions. It is now possible to manage the entire traffic signal networks via the cloud, setting customized policies for prioritizing different types of road users on specific arterials at certain times of the day. This technology also allows for measuring the impact and demonstrating improvements in congestion, safety, and sustainability. These advancements create a massive opportunity both in terms of impact on the physical space and for companies pioneering these sectors and utilizing these technologies to build market-leading companies.” ~ Tal Kreisler, CEO and Co-Founder of NoTraffic
Additionally, sensors now embedded into road surfaces can detect when someone is crossing the street, and adjust the timing of the signal change to real-time activity. This reduces the wait-time for both vehicles and pedestrians, and further enhances public safety by reducing the incentive for pedestrians to cross the road without waiting for a green man and for cars to skip red lights.
Traffic management services which utilize AI, such as smart traffic lights, smart parking, and enhanced GPS, are all part of a broader concept of creating intelligent transportation systems for cities.
So far, cities which have adopted intelligent transportation systems have seen tangible improvements. Phoenix, for example has seen a 40% decrease in vehicle delays thanks to its adoption of smart traffic lights.
Obstacle detection
Reduced delays are not the only benefit that AI is bringing to urban transport management. Enhanced pedestrian and passenger safety is another big incentive for city councils to invest in smart, AI-powered infrastructure for their roads and intersections.
Currently, vehicle collisions account for approximately 1.19 million deaths worldwide per year. In many cases, these deaths are preventable, and not just by imposing stricter restrictions on drivers – AI is already being used in obstacle detection systems, helping both drivers and autonomous vehicles to avoid collisions on the road.
For autonomous vehicles, obstacle detection systems are especially crucial. Autonomous vehicles must be fitted with both AI-enhanced sensors and a microprocessor. This facilitates the immediate processing of real-time, local data and informs the vehicle control system when to stop or start, slow down or speed up.
There are several different types of sensors that are used in autonomous vehicles, including laser, optical, and acoustic. Most obstacle detection systems use a combination of these types to provide a more comprehensive picture of the environment around them.
Automatic vehicles, driven by humans, are also fitted with ultrasonic sensors on the front and rear bumpers which can detect objects. However, these sensors are more limited, and can only detect nearby objects and measure the distance between the obstacle and the car, and so are mainly used to help drivers park rather than avoid collisions.
Fully autonomous vehicles, which have more advanced and longer-range obstacle detection abilities, are still being optimized for performance to guarantee public safety. Nevertheless, under the supervision of safety drivers, they can already be found cruising the streets of several US cities, and are set to be deployed in the UK in 2026. This is testament to the efficacy of AI-optimized obstacle detection systems, which are now nearly able to fully replicate and exceed human detection abilities.
Additionally, AI-optimised roadside camera surveillance is helping to improve long-range obstacle detection systems by collecting real-time data from numerous tracking points along roads, and sending this intelligence to vehicles ahead of time. This improves safety by giving the driver more time to stop, reducing the chances of the vehicle colliding with the obstacle, or swerving and crashing elsewhere.
Furthermore, by training the AI detection systems on local data (i.e. the species native to the local area), these sensors are helping to protect wildlife by alerting drivers of automatic vehicles to not only the presence of an obstacle in their path, but also what the obstacle is.
The additional intelligence on what the detected object (i.e. a fallen log as opposed to a wild animal or human) also enhances passenger safety by helping vehicles take an appropriate course of action in response to the type of obstacle detected.
Maurizio Rinaldi, CEO of SONDA USA, a global digital transformation company specializing Smart City projects around the Americas, identifies this as a key use-case for AI in urban transport management.
“An important yet easily overlooked use-case for AI that we have encountered is wildlife detection. This is actually the cause of a lot of car accident and fatalities in the United States. Cities are looking at how to manage this problem and find ways to coexist more peacefully with wildlife by utilizing AI to detect wildlife on roads and prevent accidents occurring from that.” ~ Maurizio Rinaldi, CEO of SONDA USA
Studies are also underway to implement AI-powered wildlife detection for railway systems, which can be a significant threat to wildlife populations in both urban and rural environments.
Barriers to AI adoption in transport management systems
Although AI is already being used for various use cases in transport management, its rate of adoption remains slow and cautious. This is due to the risks of adopting AI in public services where the liability for mistakes and accidents falls on governmental organisations, which have less freedom to take risks than private sector companies.
Furthermore, these systems have faced significant implementation challenges, particularly in modernizing transport management infrastructure on a widespread level to facilitate the data processing requirements of AI systems.
Cybersecurity concerns
In the digital age, cybersecurity is a major concern for many businesses and organisations. Cyberattacks are on the rise, posing a significant security threat to digital systems, which must now be more resilient than ever against the growing volume and sophistication of these attacks.
For transport management systems (and public services in general), the risk is even higher. Indeed, as AI is integrated into an increasing number of critical urban operations such as transport management, cyberattacks could disrupt daily life for civilians on a much vaster scale.
This is already evident, with Microsoft’s recent IT outage triggering widespread disruption across several services such as air/train travel, media broadcasting, and healthcare, with many organisations experiencing a temporary service restriction or cease of operations.
Such incidents, along with the potential for even greater disruption, could act as a deterrent to city councils and governments in further adopting AI for transport management systems, despite the fact that most digital transformation projects now have measures in place to protect systems from cyberattacks and minimize the potential for service disruptions.
For example, Rinaldi explains that strong cybersecurity systems are an integral aspect of SONDA’s smart city projects.
“Cybersecurity has been a major area of focus in city operations, not just for us, but for many corporations and governmental organizations more generally – this is even without taking AI into consideration. Technology is very present in many aspects of our operations, from the manufacturing process to the end-user experience. Security is therefore a big concern across the whole pipeline of our operations. When it comes to deploying smart city technology, we must operate under the assumption that all of our initiatives require strong security systems, not only in the technology, but also in the data.” ~ Maurizio Rinaldi, CEO of SONDA
Public safety
Cybersecurity is not the only risk that must be accounted for when deploying AI in transport management systems. There are also significant physicals risk to public safety, which can lead to catastrophic consequences if not properly managed.
For example, back in 2018, one of Uber’s fully autonomous vehicles killed a pedestrian in Arizona, while more recently, in April 2024, a Tesla car in full self-driving mode hit and killed a motorcyclist in Washington.
Such incidents are not only significant setbacks for the autonomous vehicle industry, but they can also undermine public trust in the safety of AI for transport management systems more generally. This highlights the importance of prioritizing human safety in the development and deployment of AI systems.
For city councils and public sector organisations, prioritizing human safety is particularly important due to their accountability to the general public. This means that the rate of AI adoption in public transport management systems is likely to remain on the slow and cautious side.
Furthermore, given that Generative AI is prone to hallucination, bias, and unpredictability, there is an inherent risk to public safety in using this technology in transport management systems without human oversight. For at least the near-future, this means that there will be limits on the extent to which AI can be deployed autonomously in transport management systems.
Outdated infrastructure
Intelligent Transportation Systems typically utilize a range of IoT devices which are installed in urban areas to collect local data, and facilitate connectivity within geographically dispersed transport systems. Edge processing also plays a key role in many of these systems, facilitating the processing of vast amounts of real-time data locally and immediately.
However, the implementation of these technologies has been hindered by the often outdated infrastructure used in traditional transport management systems. According to Kriesler, CEO of NoTraffic, the lack of digital communication infrastructure at road intersections has been a particularly significant barrier to the implementation of Intelligent Transportation Systems, which require significant investment for modernization.
“Traffic management is one of the last domains yet to undergo digital transformation. The lack of communication, data, and computing power in signalized intersections has posed significant challenges to their modernization. The massive investment required just to deploy communication in cities is immense.” ~ Tal Kriesler, CEO and Co-Founder of NoTraffic
However, Kriesler also points out that continual developments in technology are set to bring increasing ease of implementation for cloud-based and/or edge processing systems.
“Recent advancements in edge computing and AI now enable retrofitting and digitizing signalized intersections in less than a couple of hours with sensors equipped with built-in communications, powerful computing, and advanced AI algorithms.” ~ Tal Kriesler, CEO and Co-Founder of NoTraffic
Furthermore, strategic collaborative partnerships between digital transformation companies and computing hardware suppliers is also helping to overcome some of the implementation challenges for Intelligent Transportation Systems, by accelerating the development of purpose-built, end-to-end AI systems.
For example, SONDA has recently partnered with leading computing hardware provider, Intel, and Intelligent Security Systems (ISS), to offer cutting-edge video intelligence capabilities for traffic management systems, powered by advanced AI.
“Through our strategic partnership, Intel, SONDA and ISS are at the forefront of transforming traffic management and safety, leveraging advanced AI at the edge to deliver innovative and efficient solutions.” ~ Anthony Abuta, Transportation Global Director for Cities & Critical Infrastructure BU at Intel Corporation.
Is personalized transport management the future of city life?
One of the strongest trends that has emerged alongside AI is personalization. While this trend is primarily associated with the retail industry and the education sector, there is also potential for personalization to shape the future of urban transport management.
According to Rinaldi, AI could be used in the future to promote accessibility in cities through the personalization of traffic management systems, and also to improve the quality of urban life by personalizing public services more generally.
“For traffic management, the potential of AI is huge, and new applications of the technology can provide services that today the community are not experiencing. If you think about the standard traffic lights system, it doesn’t really take into account people with special needs, mobility restrictions, or hearing impairments. When an old person is crossing the street, for example, they require more time to cross. These situations can be managed in a different way to the benefit the community and everyone in it – and that’s what really matters. What most cities are trying to do with AI is to provide more personalised services and new services to the community so that the city is a better place to live in.” ~ Maurizio Rinaldi, CEO of SONDA
There is good reason to believe that personalization could enhance the quality of life for citizens. According to a 2021 McKinsey survey, 71% of consumers had come to expect a personalized shopping experience, with 76% becoming frustrated when it was not offered – and these statistics are likely to have become even higher since. This indicates that personalization, at least in a retail context, is generally very positively received and improves consumer satisfaction.
Nevertheless, there is arguably some inherent irony in the very idea of personalizing transport management systems. As centralized, city-wide systems, aren’t they are intended to serve the general public, rather than provide a personalized service that caters to the preferences and needs of each and every individual? In this sense, personalizing systems which are intended to serve the general public seems to undermine their fundamental purpose, i.e. providing a standardized, generic service that citizens can use anonymously.
More generally, we also have to consider the potential downsides of personalization in public services and across physical urban spaces. For instance, do we really want to live in a world where a central city billboard displays personalized ads targeted at the digital profiles of the audience detected to be walking by at any given time?
Implementing personalization in transport management
For argument’s sake, let’s say that the pros of personalization outweigh its potential cons. We then have to consider the plausibility of actually implementing personalization in transport management systems.
With traffic light systems, for example, there are a couple of key considerations that lead us to question whether these systems could really be personalized down to an individual level.
First of all, not all people with special accessibility requirements display detectable attributes. According to a Forbes article, over 42 million people in the US have a severe disability, and approximately 96% of them are unseen – and these invisible disabilities can have a significant impact on mobility, affecting ease of movement, spatial awareness, risk awareness, etc.
This means that even if traffic light systems could be personalized by using visual sensors to detect individuals with special accessibility requirements and adjust crossing time accordingly, a vast majority of these people would miss out on this due to invisibility of their condition. This could then raise ethical concerns about the equity of such systems, which may end up unintentionally discriminating against various types of disabilities which they could not accommodate a personalized service for.
Secondly, personalizing traffic light systems could raise significant data privacy issues due to the potentially intrusive level of public surveillance it could involve.
In the retail industry, personalization has already been subject to valid concerns over the collection and use of consumer data. This has led many commercial businesses to introduce more ethical data collection policies to respect consumer privacy, such as providing opt-out options on their web pages.
With the EU AI Act coming into force today (1st August 2024), such policies are now legally required for retailers engaging with the EU market. According to Steve Lester, CTO at digital solutions company, Paragon, businesses need to be prepared to re-evaluate the ethical standards of their AI practices, particularly those which involve personalisation and consumer targeting strategies.
“Businesses must prioritise transparency and ethical AI practices. For customer communications, this means clearly disclosing when AI is used and ensuring that targeting and personalisation strategies adhere to the Act’s guidelines.” ~ Steve Lester, CTO at Paragon
This would create further complications for the personalization of public services such as traffic light systems, given that users can’t exactly ‘opt out’ of crossing the road. Personalization of these systems based on analysis of visual data collected through public surveillance cameras would therefore be both unethical and illegal.
Indeed, for many individuals with special accessibility requirements, personalized services may neither needed nor wanted. This raises the issue of how ethical personalized public services would be, particularly in use-cases where users of the service would be unable to opt out of them. This underscores the importance of ensuring that individuals have a say in both if and how public services are personalized, even if this is for their supposed benefit.
Striking a balance
Overall, the complications we have considered above point to the implausibility of extensively personalising public services such as traffic light systems down to an individual level.
However, there is certainly a balance to be struck, and it is highly probable that transport management will see some degree of personalization in the near future. This would likely be based on more generic factors such as location, rather than intrusive public surveillance. For example, traffic light systems near hospitals, schools, and nursing homes could be personalized to have longer crossing times to account for the general needs of the types people likely to be in those areas.
Nevertheless, even these milder personalization strategies still face the risk of discrimination against minority groups, or forcing unwanted services on various groups of people.
In recognition of this, Rinaldi highlights the need for decision makers to involve local communities in the development of AI-powered urban management services, even though this could potentially slow the adoption of AI in some use cases.
“In the end, it is important that leadership is also about making sure that there is involvement of the local communities. You don’t want someone in an office defining what service or personalized systems are good for a community without involving the people that these systems would impact.” ~ Maurizio Rinaldi, CEO of SONDA