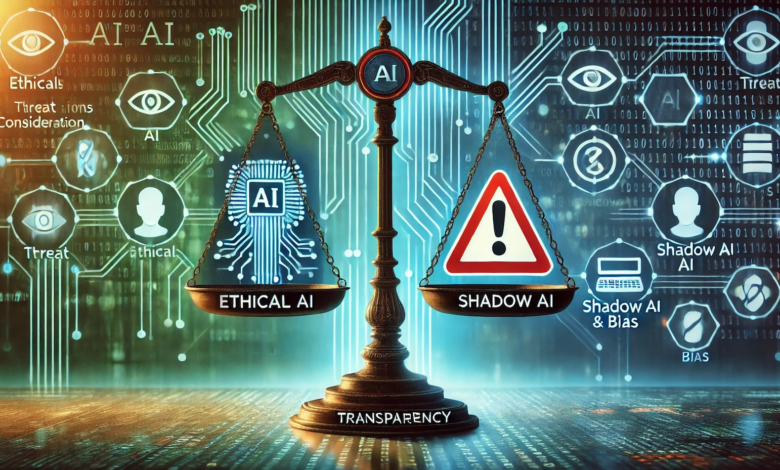
While AI is making waves across industries, its application in cybersecurity isn’t novel. For over a decade, machine learning has been integral to real-time threat detection, analysis, and mitigation. It has proven invaluable in identifying ransomware, phishing attacks, and even insider threats by distinguishing between normal and abnormal network activity. These AI systems are trained on data of known threats and behaviors, employing heuristic models to flag suspicious activities and alert enterprises to potential risks.
The risk of generative AI becoming shadow AI
Fast forward to 2023, and the newest form of AI – generative AI – has catalyzed widespread adoption across sectors, both formally and informally. Its ability to rapidly create diverse content has made it very attractive in both personal and professional spheres. However, this swift adoption echoes the early days of mobile phones and cloud apps, giving rise to a new phenomenon: shadow AI. Similar to mobiles and cloud apps, today generative AI tools are being adopted without proper approval, training, or usage guidelines, potentially putting enterprises at risk.
A proactive, transparent, and responsible approach to AI integration (and not just generative AI) within enterprises is needed, complete with the proper cybersecurity guardrails and frameworks so that AI doesn’t become another form of Shadow IT.
Challenges in AI-driven cybersecurity
Adopting AI in cybersecurity presents several significant challenges. First and foremost is the ethical consideration. Ensuring responsible development and use of AI technology is paramount. AI systems must be built with inherent security measures, adhering to the principle of ‘security by design’.
When adopting external AI security tools, enterprises’ scrutiny of their trustworthiness, limitations, and controls is essential. Questions such as “Do your AI tools use trusted models?”, “Are the third-party entities trusted providers?”, “What disclosures are being made about the limitations of the tool?”, and “Are there technical controls that prevent the AI system from being used for purposes other than what it is designed for”?, are all valid and should be carefully considered.
An analysis of responses to such questions will not only help determine whether the principles of ethics are baked into the solutions, but also enable enterprises to determine tools’ risk profile. Let’s face it, even reputable AI models can have vulnerabilities. In 2023, a bug in an open-source library caused ChatGPT to expose users’ personal information and chat queries. This incident underscores the importance of rigorous security measures, even in bleeding-edge, widely-used AI systems.
Addressing bias and privacy concerns
Bias presents another significant challenge in AI-driven cybersecurity. In cybersecurity, the bias is around false positives. AI systems may generate false positives or miss new attack vectors. This is because security solutions developers typically use data from specific geographies or jurisdictions, which can lead to biased results. This situation demands an active feedback loop with solution providers to address data quality concerns, improve transparency, and enhance explainability. Such an approach ensures continuous refinement and can help reduce complexity in algorithm design and data modeling techniques, leading to better understanding and eliminating the typical ‘black box’ quandary.
Data privacy and confidentiality have become paramount concerns for enterprises due to the use of unsanctioned AI tools. The infamous Samsung incident, where employees inadvertently leaked source code through ChatGPT, despite OpenAI advising users not to share sensitive information with the tool. This event highlights the risks associated with using public AI tools for sensitive information, of course, but more importantly, the value that ChatGPT delivered to the users outweighed the security risk – even for software developers who understand the technology and security landscape better than most.
At the time, many organisations initially responded by banning such tools. However, is outlawing the use of generative AI tools the answer? History shows that prohibiting shadow IT is rarely effective. A more realistic approach might involve deploying endpoint security measures to constrain the data that can be input into public generative AI tools.
The evolving regulatory landscapes
The good news is that – perhaps for the first time – regulation is more or less keeping pace with the rapid development of AI. Comparing AI regulation to that of cloud computing, in the case of the latter, it took a good six to seven years before specific controls were integrated into regulatory frameworks.
The EU’s AI Act is incorporating generative AI, focusing immediately on high-risk applications and datasets, and emphasising transparency and accountability. There’s the NIST AI Risk Management Framework (AI RMF), and the ISO 42001, which offers a promising approach to AI governance. Additionally, ISO 42001 will bring a level of consistency and reliability across borders.
The complex and dynamic nature of AI technology necessitates equally dynamic and complex governance. Current frameworks will likely evolve significantly within as little time as a year. As this technology advances, so too will the frameworks designed to govern it. However, the foundational principle guiding this initial and future phases is clear: responsible AI development and deployment.
Enterprises’ own guard rails
To foster responsible AI adoption, enterprises need to create an environment and culture that supports it. Employees must know what generative tools and apps are allowed and which ones aren’t. Managers need to be cognizant of how their teams are using these tools, what data they are sharing, and how the outputs are being deployed to ensure that the ethical use of AI is embedded in the organisation.
An enterprise can believe in ethical AI, but ethics don’t implement themselves. Enterprises must proactively determine their own rules and operationalise their execution and governance, taking into account security, business, legal and regulatory risks. To inform this approach, conducting a business impact analysis of the AI tools in the context of use case and risk-benefit, can be helpful.
While it’s true that AI is a game-changing weapon in the adversaries’ attack arsenal, it is also an equally impactful tool to defend against their attacks. Embedding AI as part of a layered security approach is the most effective way of successfully counteracting the technology’s weaponisation by bad actors. Take business email compromise (BEC), a tactic that continues to be relentlessly deployed by criminals, with a large proportion of the BEC emails being AI-generated, making them extremely difficult for employees to spot. By deploying AI routinely for mapping email usage and behavioral patterns, enterprises can identify which emails are suspicious, flagging them for further investigation.
AI security awareness and training are key components in building a robust defense too. With vigilant employees, enterprises become well-positioned to effectively move towards self-service cybersecurity. They can provide Natural Language Processing-based tools to enable employees to identify fraudulent activity independently and escalate only those threats to infosec teams that need expert intervention.
Balancing AI and human expertise in cybersecurity for ethical use, privacy, and compliance
Regardless of how advanced or sophisticated a technology might get, it’s crucial to remember that no technology is ever fool-proof, and AI is still in the very early stages of its evolution. Periodic and timely human oversight is essential – to ensure that the ethical principles set out are being duly followed, the technology and algorithms are properly functioning, and that the enterprise is complying with all the necessary regulations. To truly harness the full potential of AI in cybersecurity, a balanced framework along with checks and safeguards to mitigate risk is indispensable.