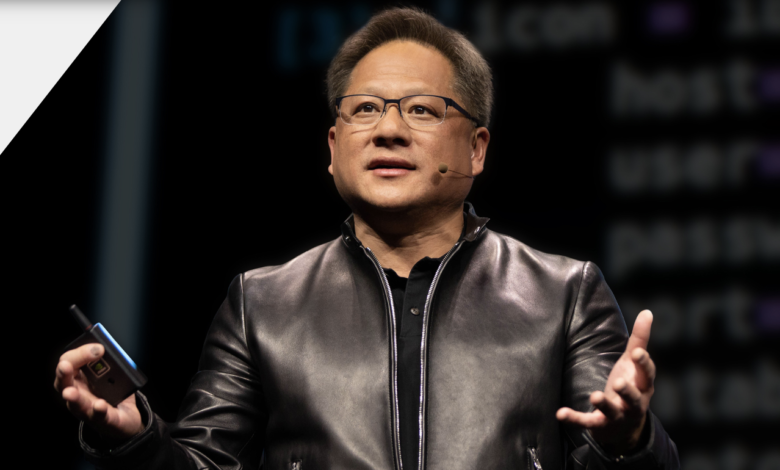
Earlier this week Nvidia hosted their GPU Technology Conference where they outlined some of the success they’ve had for the year already and what’s to come in the future.
Nvidia reported in their Q1 2020 earnings that their revenues were up 39% to a total of $3.08bn by the close of Q1 on April 26 which are higher than the forecasted amounts thanks to the use of their AI and Cloud capabilities.
Nvidia’s datacenters have played a key role in achieving the growth with the datacenter’s revenues up 80% from the year before to $1.14bn making up close to 35% of Q1 total revenue.
To put the growth in perspective, in 2019, Nvidia’s revenues for the first quarter were $2.22bn.
Data Centres
Nvidia’s CEO Jensen Huang explained in his GPU Technology Conference keynote speech that “in the next decade datacenters scale computing will be the norm and data centers will be the fundamental computing unit” with that being the rationale for buying Mellanox, a maker of key technologies for connecting its chips in data centers and world leader in high scale networking, for $7bn earlier in the year.
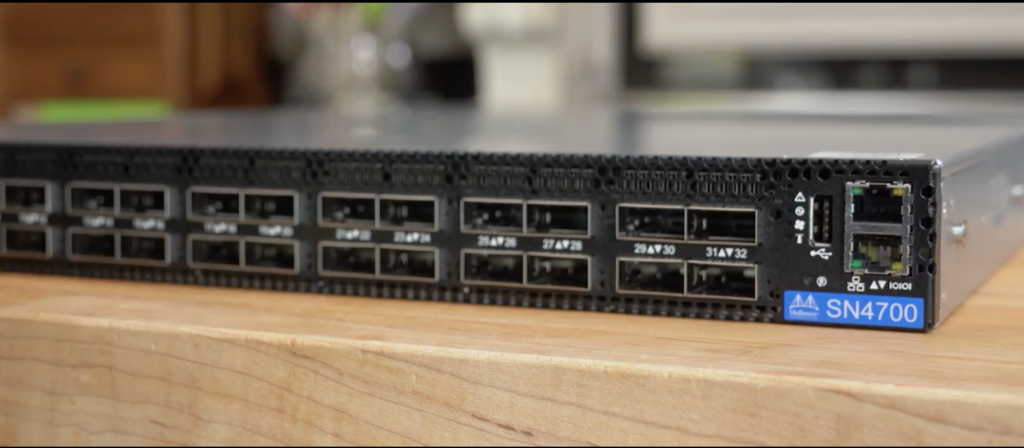
Huangs GPU Technology Conference keynote speech was viewed 3.8 million times in the first three days and had more than 55,000 registrants who participated in the online-only event to see the reveal of their upcoming initiatives usually hosted in a physical event setting
The keynote speech touched on last week’s launch of the Ampere-based A100 GPU, an enormous AI chip with 54 billion transistors at the GPU Technology Conference.
Fighting COVID-19
Huang opened his keynote at the GPU Technology Conference by saying thank you to first responders, health care workers, nurses, doctors, truck drivers, warehouse workers, service workers, and all of the people who are doing everything to keep the world going and who are fighting COVID-19.
Huang explained how Nvidia are doing their part to help in the fight against COVID-19 by saying how they are working with scientists across the entire spectrum from containment, mitigation, treatment, and tracking and monitoring.
Nvidia’s is helping scientists do their work faster across the spectrum above through examples such as using real-time infection analysis, screening 1bn drug compounds in one day, and using an AI model to classify COVID-19, and using thermal imaging for body temperature, among others.
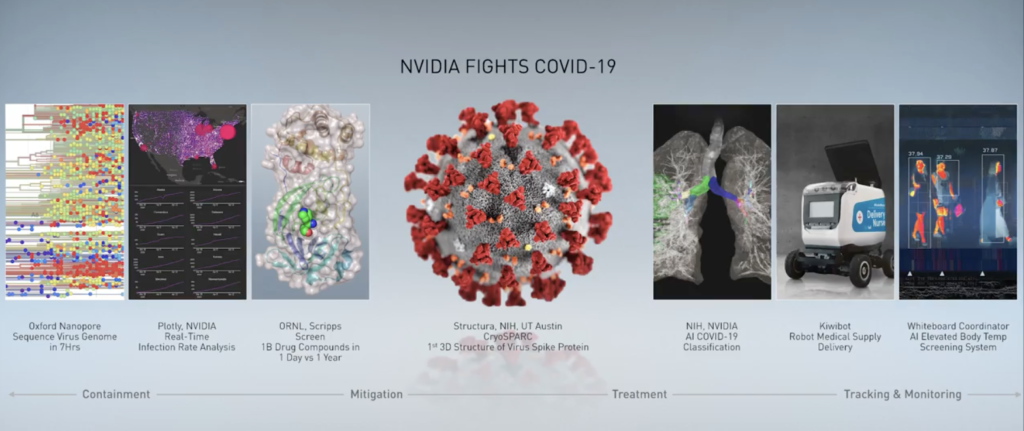
Nvidia has made donations on that front to help with the effort which have amounted to more than $10 million from team members alone.
Gaming is on the rise
The game industry revenues for Nvidia’s chips have also grown with the gaming revenues totaling $1.34bn, up 27% from the same period a year earlier with more than 100 new model laptops now using Nvidia GeForce GPUs with RTX technology.
At the conference, Huang explains the power of the Nvidia RTX chip by saying it’s a “new era in computer graphics” thanks to their ray tracing, deep learning, and AI capabilities.
The chips got a demonstration of their power by looking at the same still shot of a game on different frames such as Ground Truth 16K, DLSS 2.0, and Native 540p with leading game title Minecraft showing the results of better shadow and lighting effects.
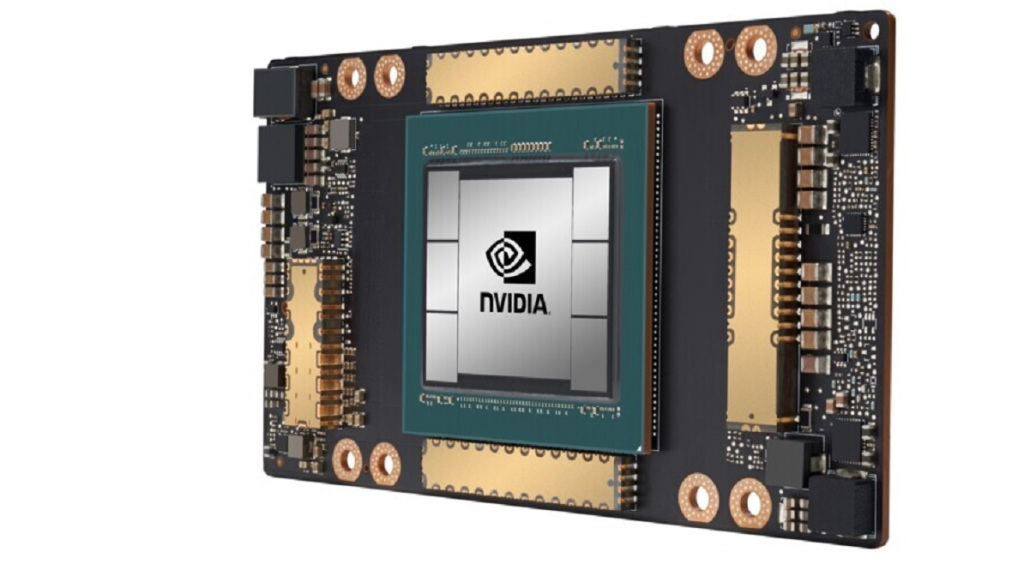
The chips are supported by the Nvidia Omniverse which has capabilities that include a design workflow collaboration platform, a built on USD universal scene description, a built-in interactive renderer with materials, and physics while being supported on PC and Linux allowing for streaming on Macs and Androids.
Conversational AI
Nvidia introduced a new application framework or conversational AI called Jarvis and showed how it’s functioning through a multimodal conversational AI services framework and the machine learning method the 3D conversational AI model is using.
This way you can use the model you’ve trained through machine learning and implement it into your chatbot so it can mimic its mouth movements to what it’s saying and when answering your questions.
The capability will be useable across a range of industries and technologies including video conferencing, call centres, in smart speakers, for retail assistants, and in-car assistants.
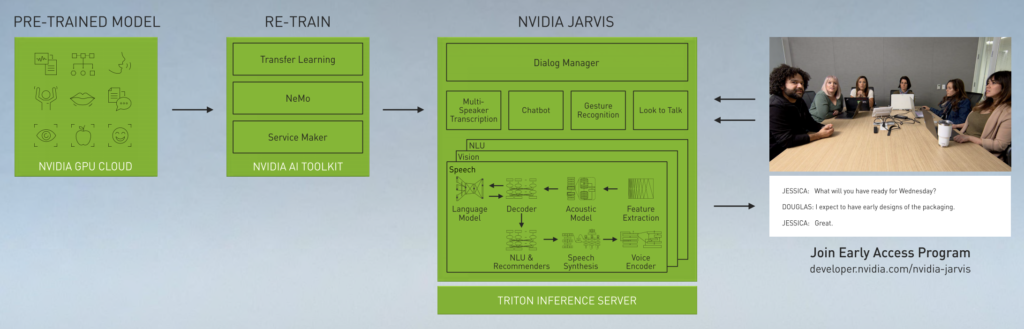
Huang explains how the ability to have an interactive conversation requires a conversational AI to process the speech, the natural language understanding, synthesis, and also render the graphics as fast as possible.
The entire pipeline has to be processed end to end from speech recognition through to language understanding all the way through to generating and driving the computer generated graphics in just a few hundred milliseconds so the end user feels like they are having an interactive conversation.
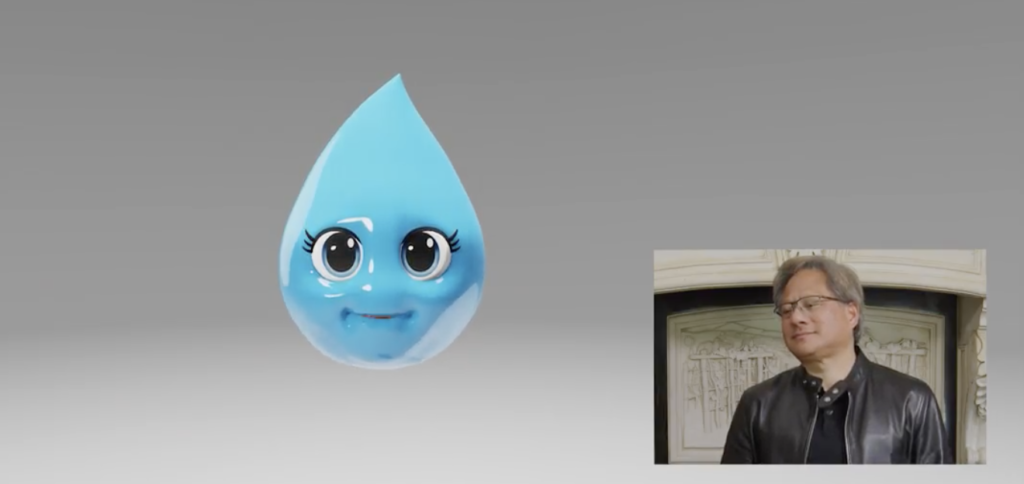
Jarvis allows for this by having state of the art models above that simplifies the creation and development of conversational AI services similar to the way Facebook is using and designing its conversational AI.
Autonomous Vehicles
Nvidia’s data centres are being implemented into cars which Huang refers to “data centres on wheels” that have to make decisions at such a rapid rate making it one of the greatest computing challenges to Nvidia due to 10 trillion miles being driven each year.
Huang announced that the Ampere Architecture is going to be available on the Nvidia Drive that allows it to scale up a range of new capabilities to the entire range of everything that can currently move.
They gave attendees to the GPU Technology Conference a glimpse into how the software might work in the real world and how quickly the system could pick up faults through sensors and provide safe autonomous driving practices from trucks through to delivery bots.
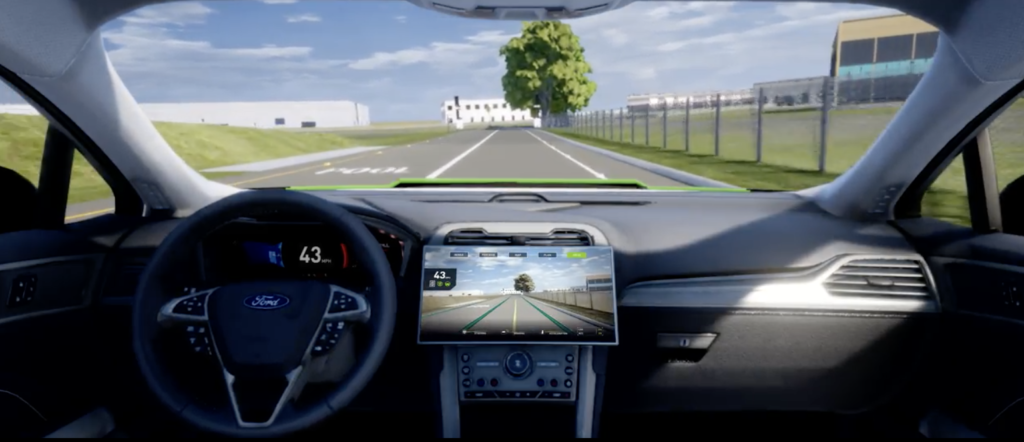
They showed off their opinion of how the smart revolution is going to affect a wide range of products, services, and connected devices and that BMW will be using AI and IoT devices powered by Nvidia Isaac Robotics to create their next-gen factories.
These types of capabilities include allowing for a powerful in-car computer for a full self-driving autopilot car, having a driverless robot taxi, to being able to teleport yourself into the mind of the car so you can remotely control the car.
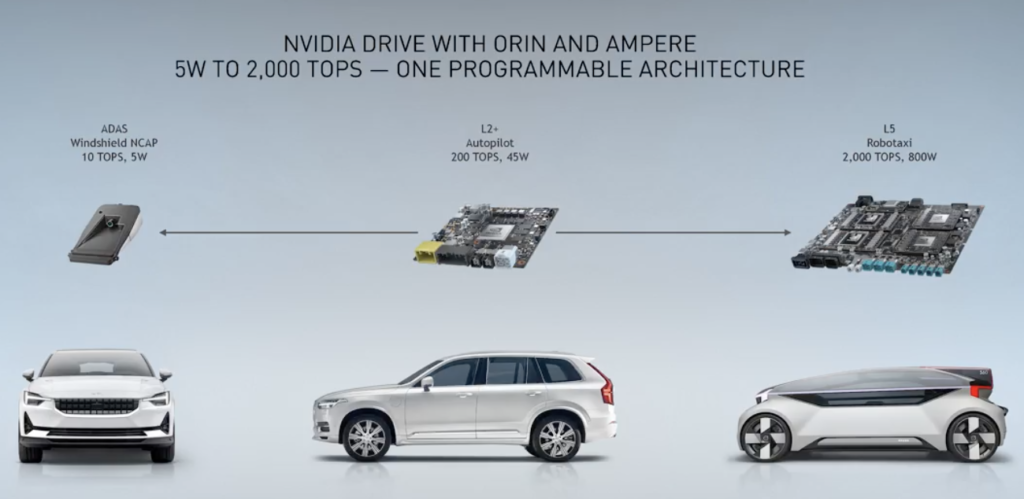
Nvidia outlined how they’ve thought of every step in their drive system by using a software-defined AV platform that goes through a controlled quality check process from collecting the data all the way, simulating it, machine learning the simulation for improvements, and then testing it on the cars.
The end to end pipeline and end to end infrastructure will be used across a range of available vehicles and suppliers including cars, trucks, suppliers, mobility services, startups software, mapping, and simulation.