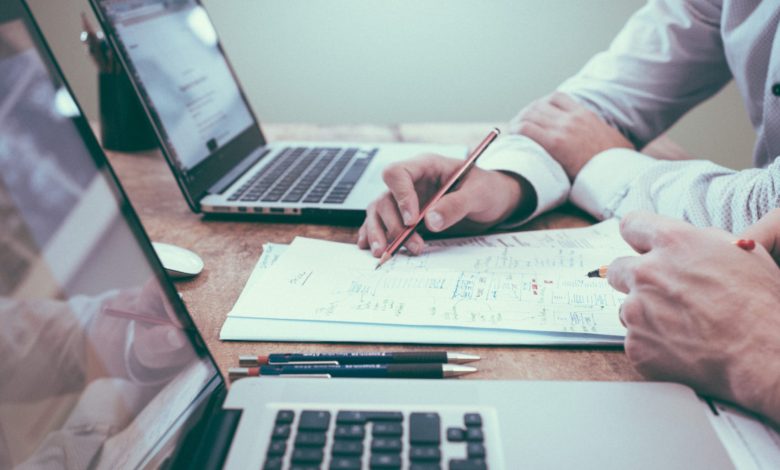
About a month ago, I was invited to participate in the panel discussion at the October 2019 AI World Summit in Amsterdam. This was an event of a global magnitude, attended by “who-is-who” of the AI community. Some of the luminaries included the likes of Werner Vogels and Stuart Russell delivering keynote speeches.
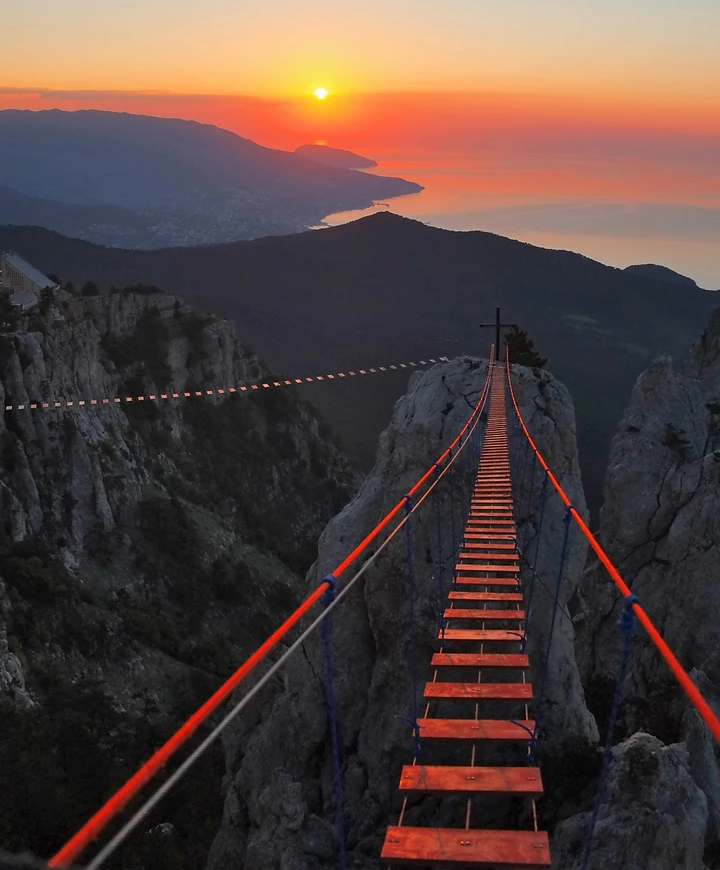
In Amsterdam, I have presented my views on the AI opportunities and the state of AI in retail, absorbed tons of information, and have corroborated my key conviction that AI is not a crutch, it is a bridge. This, not to be cute, it’s the paraphrasing of a well-known quote by Jaggi Vasudev –“a Guru is not a crutch, he is a bridge“
Truly, AI is a means to an end – it is not a goal, an objective, nor it is a silver bullet.
In fact, 90% of real-life problems are best solved with common sense and good-old descriptive business intelligence.
Using AI to answer most run-the-business questions, to validate the linear hypothesis, is like going duck-hunting with a cannon. Moreover, AI does not substitute an intuition, nor it can drive the decision on limited, incomplete datasets.
So, why then AI, by far, is the most exciting intersection of technology, data science, and business today?
Why is there so much energy, interest, development, and investment pouring into the field of Machine Learning (ML)?
The reason is clear: it is that remaining elusive 10% of business problems that can be answered with the help of AI that is going to separate winners from losers.
No company can remain competitive without a full-scale enterprise AI adoption. And, I am not only talking about the technology and data science, of course – the whole company must evolve to with this adoption.
Since more and more companies realize its significance, the AI market today is over-hyped, full of exaggerated claims, and PowerPoint-ware, leaving many corporate observers bewildered and confused, unable to fathom the true relevance, benefits, approaches, and complexities of AI implementation.
Incidentally, this is true across all the market verticals, not only just retail.
One of my favorite quotes that I cite in the Quotes page of this website is from Sophocles: “Nothing vast enters the life of mortals without a curse.”
Enterprise AI certainly fits the bill.
So, let’s explore some true immediate and strategic opportunities and applications for AI today.
When I think of a “blue ocean” state for a modern large retailer, I think of the 5 essential criteria:
- Consistency of Intelligence an User Experience at Scale. It would be super cool for a large company to locally have a customer insight and enjoy a good-will of a neighborhood “mom & pop” store where an owner knows its customers, their tastes, children’s names, dog names, wedding anniversaries, etc. These businesses are often perceived not as a for-profit concern, but as an indelible part of the local community. And very often they truly are;
- The economy of Scale that includes well-integrated service modalities, multi-presence capabilities, purchasing power, brand leverage, and reach of a national retail brand, operating thousands of stores across the country;
- Corporate Culture of a Software or Internet Company. Don’t think as a traditional retailer! You are not there to sell stuff. You’re after to change the world. Do away with the traditional separation of IT and business, “IT for business” type of mentality. IT is business! The retailers who are going to dominate are indistinguishable in the way they think from the leading high-tech companies; In fact, the most successful retailer in the world is the software and SaaS/PaaS company. So, this is not really debatable;
- Deliver Hyper-Personalized Experience that includes transparent and consistent Services, relevant Assortment, appealing Promotional Offers. These are to be accompanied by a low total cost of ownership (TCO), reduced friction, and product lifecycle strategies that incentivize seamless subscription or upgrade cycles.
- Transformation to a Direct-to-Consumer(D2C) model, building digitally-native vertical brands that are distinguished by complete control of customer experience and product lifecycle. These brands experience explosive growth, greater customer affinity and deliver a 5x to 10x margin of traditional retailers.
This is not a quixotic state of affairs. For some of the visionary retailers, like Warby Parker, Thread, Dollar Shave Club, MTailor, StitchFix it is a reality.
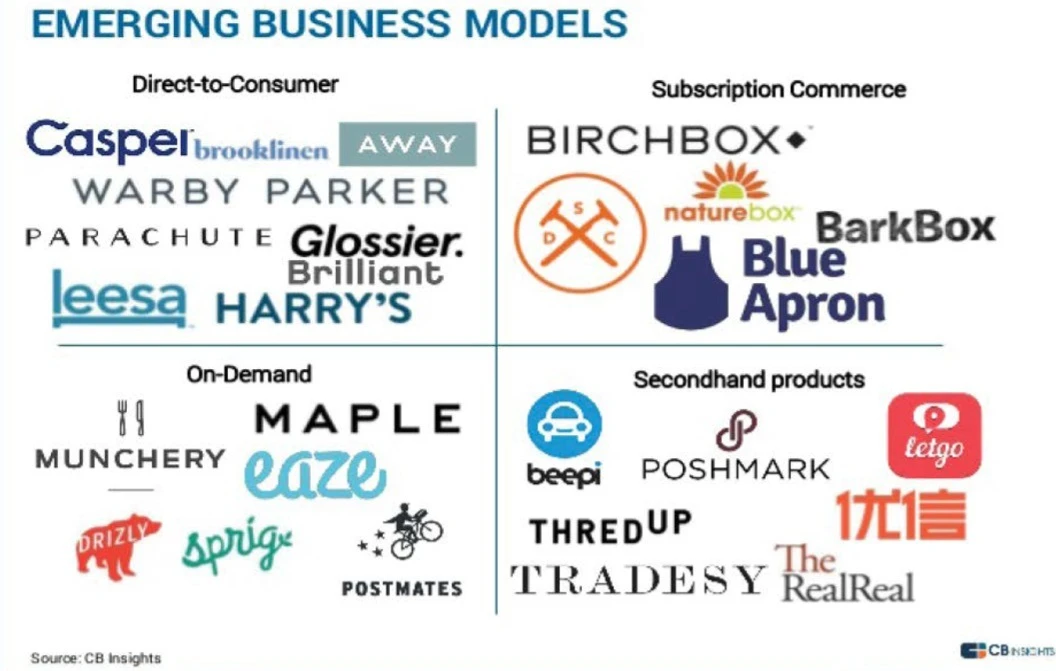
Their secret sauce – a. maniacal focus on customer and b. pervasive and increasing adoption of AI to drive all aspects of business workflows.
Let’s expose then the real-life applications for AI for retailers. From the top, there are two primary domains of AI implementation:
- To gain incremental improvements, find new operational efficiencies, create better customer-relevancy in the scope of the current business model. I am going to refer to it as Boost.AI
- To discover game-changing, disruptive opportunities, to re-invent and to transform the current business model – Disrupt.AI
The Boost.AI
In our first “bucket” we find the most typical applications of AI today, generating a multitude of scoring models (also referred as prediction models.) They all convert Input data into Output, based on the predictions (scoring) model assigned to the Output data. The latency may differ from batch to near-real-time to real-time. This is where 99% of current implementation and AI adoption takes place. Representative ML models use-cases are built for the following AI problem set:
• Customer Propensity to Buy;
• Customer Propensity to Churn;
• Fraud Detection Pattern;
• SPAM/SCAM/Fishing Patterns;
• SKU-level Assortment, Merchandise Planning, Demand Forecasting;
• Anomaly condition detection: outlier performers, etc.
• Static Customer Segmentation;
Then, somewhat further down the line, most forward-thinking companies use ML to:
• Create ontologies between items and categories to drive sales;
• Gauge data source signal accuracy;
• Improve Data Quality, including reduction of data duplication, creating inferred definitions on poorly defined datasets

These outputs are typically visualized in content-rich dashboards, providing data scientists and business users with an innate insight missing from the legacy metric-driven reports.
The state-of-the-art of AI in the enterprise is realization that wide and deep-level operationalization of ML is totally mandatory to maintaining a long-term competitive edge.
I am convinced that any company under-investing in AI will be out-competed. And NOT in the long-term – the rate of change is ever accelerating, and AI is going to be like turning-on afterburners to get away from the bad guys, aka, competition.
Then, good, we have our recipe.
Not really.
A simple run-down through the use-cases above suggests that none of them, taken separately, creates profound process changes that could lead to uncovering long-term opportunities leading to strategic transformation of the business model, to “blue ocean”. Here is where our second “bucket” comes in.
Disruptive AI Enterprise opportunities
The promise of AI carries bigger, more strategic opportunities to deliver the game-changing transformation of current business processes, foster better organizational alignments, and create new business models. Let’s start from the most obvious:
Replacing NPS scoring with a Contextual/Dynamic Customer Affinity models inferred from comprehensive datasets continuously tuned to ever-changing customer journeys. Indeed, to think of simplistic NPS as a sufficient metric for the measurement of customer satisfaction is, to put it mildly, myopic. One must develop a comprehensive set of pre-built models that can be invoked to measure how well marketing offers and product features to match customer’s expectations.
To maintain full control of customers’ journeys, to meet or exceed customers’ expectations, to foster relationships, not transactions, we need to be less verbose, more relevant, more attuned, and much more optimized.
We need to hash-out all aspects of company engagement, customer social media activity, post-sales, and pre-sale journeys.
We need to be obsessive about our data acquisition and sweat details, such as cart abandon rates, the elasticity of our price functions, prior store visits, and online sessions, competitors’ actions.
We need to understand how well our product catalog and search results match a customer’s expressed interests, and how to modify our products and marketing offers to be relevant and useful.
Only relevance, low friction, and consistency (a.k.a. trust) create strong customer brand-loyalty that elevates the relationship from that initial purchase to upgrade and then to subscription.
These are the three objectives is what our ML models are to assess and to improve. The datasets feeding these customer-centric ML models cannot be solely comprised of the parochial system owned by an enterprise.
They have to include strategically acquired external data sources along with marketing and data-augmentation services provided by data aggregators and market analytics companies.
The bottom line: create dedicated to Customer-Affinity ML Models to evolve a crude NPS into a sophisticated, and contextually-aware customer expectation management platform that measures and manages customer experiences through a set of well-integrated interactive services driven by dynamic customer affinity ML models.
Understanding Cause-Effect relationship
Prescriptive Actionable Intelligence is where the state-of-the-art is. Nowadays, it is quite uncontroversial to suggest that Descriptive Analytics offers very little insight.
Yet this is where most of the business intelligence efforts are spent today – we’re creating and pushing around a myriad of reports that tell us how we did – not what should be done, basically, of close to zero value-adds.
In my opinion, one of the key perpetrators in the current state-of-affairs, and by no means the only one, is the lack of understanding of the causation behind the events.
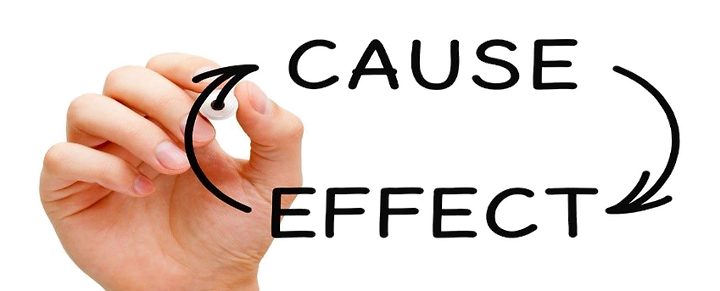
The ML is absolutely a game-changer here, as it allows us to create (train) millions of hypotheses and to reject 99.9% of bad ones, in a matter of minutes or hours.
Furthermore, we must contextualize our models by correlating external events with the model’s biggest influencing parameters.
This is important – the external signal’s content become the features of our models, organically extending event-driven architecture with enterprise AI. Therefore, we build microservices around our models and build models to support our applications.
Just keep in mind the following – the real goal of data-driven next-gen services is NOT to maximize transaction value but to improve Customer’s Value Perception!
For the former, you don’t really need enterprise AI, as far as the latter, you can’t do without it.
Now, if you think this controversial, I totally get it.
In fact, there are very few retailers that think in this fashion. But, I am convinced, enterprise AI is the one that will be dominating the market in the next 3-5 years.
Let’s take a look at an example of enterprise AI in Retail Services:
- A customer is browsing store isles, while her biometrics are captured by the in-store face-recognition enabled interactive displays. Her identity is positively identified by CNN-based model deployed in one of the clouds, and the profile is successfully confirmed and updated;
- Profile information and historical content are streamed to another AI model, say RNN/LSTM-based neural network. The content may include clustered-by-Search keywords queries, navigation history/ browsing session information, time spent per online page, products reviewed, reviews read, wish lists, structured navigation, abandon basket, in-store browse history, online & mobile browse history, in-store purchases across different categories, today’s in-store travel map, and product interactions. Our ML models process the input data and infer customer’s propensities, actual Intent, and what are the barriers to accomplishing it. That’s all! That’s is quite different from the predominant in traditional retail thinking of maximizing sales amount, pushing more and more items into the basket right there and then, overselling and offering a discount on the product that the customer has looked at. Not that it may not be the right course of action sometimes – it just can’t be the default course of action. Current thinking is too tactical, too transaction-focused, and it can be accomplished by good-old expert systems without much machine learning;
- Going back to our example. The next step is for the deployed retail microservices is to invoke product Ontologies and to correlate the resulting graph with the Customer search parameters, profile, and previous purchase history. Subsequently, the product search results are narrowed down and sorted according to the strong relationships between the items expressly sought by the customer or those that could be inferred as of interest to her.
- The results are sent as parameters to the Promo Optimization model. The parameters are the dimensions of item attributes, purchase history, “frequently bought together” graph, fulfillment strategies (offering a discount for picking up in-store vs. shipping), product hierarchy ontologies, the current availability of sales associates on the floor, etc;
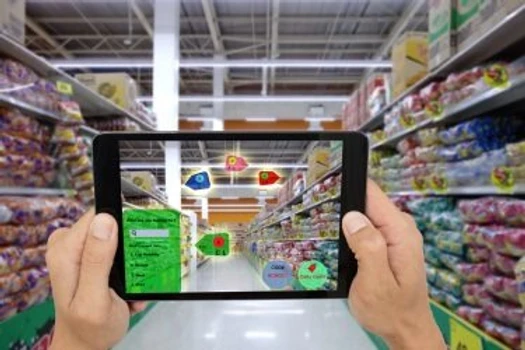
- The resulting course of action may vary, from dispatching a store associate armed with pertinent and extensive information to assist the customer, or to creating the most appealing complex deals, promotional or upgrade offers that are presented on the customer’s mobile application.
- There is, of course, an opportunity to significantly enhance this experience through the 3rd-party social commerce aggregators, creating a 2-sided marketplace, thus breaking-through the brick and mortar store “ceiling” of on-hand inventory.
- Naturally, every step of this interaction, every ML hypothesis executed by AI, is being recorded, analyzed by ML recurrence modules, and further streamed into the next interactions.
The bottom line: our services and ML model are custom built and are dynamically invoked to create highly-personalized strategies that are consistently exceeding customer expectations.

Enterprise AI enriched Retail Services
Deep Behavioral Detection
A prior example has touched on a very interesting subject of the Interpretability of customer’s intention. This is more art than science and here, like nothing else, AI can help interpreting customer expressed and inferred signals.
This Deep Behavioral Scanning intelligence can provide sophisticated detection of genuine changes in customer’s behavioral patterns.
This could become one of the key differentiation for digitally-native retailers, as it can drastically lessen current dependency on the high fidelity signals, it will cut through the signal noise, and improve rejections of false positives.
It further enables us to uncover false negatives and to interpret customer behavior across dispersed datasets. The established behavioral templates (expressed, perhaps, as finite-state machines) will help to measure and continuously improve the detection of outlier behaviors, based on both, expressed and duplicitous external signals.
The customer journey events are the parameters/events feeding this model.
They could vary from recent store-visit history (including competitors) to recent life-changing events, to the frequency of product returns, recency of call center contacts, to the number of voice-recognition assistants or chatbot interactions ending up in frustrating abandons.
Hey Siri…😊. This, clearly, has to be done at both, at a market-trend and micro-level of a single customer.
Macro-trends are self-explanatory and require listeners of social media, search engines, tech trends, etc. They typically include social metrics of the volume of mentions by product category, location and temporal dimensions, demographic profiling, sentiment analysis, influencers.
At a micro-level is where things do get truly intricate.
To make sense of it, we need to measure micro-level price sensitivity, product usage as well as fulfillment experiences for subscription-based products. The detection is triggered by statistically significant deviations from pre-defined or historically observed customer journeys.
Customer is calling call center a few times, one after another, same day, after the purchase of products, and then hangs up at one of the IVR prompts – should we proactively send mobile notification and offer to directly connect with a call center agent or a store associate?
The customer has moved – will this lead to a termination of subscription? Or vice-versa, the customer has terminated the subscription – does that mean that the customer is planning a life-time changing event or simply is no longer (permanently or temporarily?) has a use for the product?
The customer has children but stopped buying children’s clothes? Does all that mean a “change of heart” towards our brand?
Is it a zero-sum game and another retailer got the sale or does it simply mean that the kids are all grown up?
The customer has upgraded the kitchen and is researching an appliance but didn’t buy from us – did the kitchen upgrade went over-budget and the customer will surely buy that appliance from us once the credit card balances are more manageable, or a competitor made the delivery?
It is likely that the answers to our queries cannot come from just one of the signals, but may come from processing dispersed, disconnected signals that may weave a recognizable or completely new pattern. That task is greatly facilitated and enabled through enterprise AI.

Discovery of a new Business Model: Enterprise AI
Now we come to the most interesting and most disruptive opportunity. I suspect that the numerous insights of broadly operationalized AI could lead to the data-driven discovery of new business models.
This creates unparalleled opportunities for incumbents, whose business is disrupted, to reinvent themselves. And, today, none of the models offers more upside for retailers than the Direct-to-Consumer (D2C) model. The D2C companies are completely disrupting current dominant players.
Gillette’s share of the U.S.men’s-razors business fell to 54% in 2016, from 70% in 2010. Both Dollar ShaveClub and Harry’s combined U.S. share rose to 12.2%, from 7.2% in 2015.
J&J’s Acuvue: +8% YOY; Bausch & Lomb: +6% YOY; Hubble Contacts: +20% monthly
Although most people define D2C as companies with a great degree of supply chain control and no middleman between them and consumer, I think of them a bit different. D2C delivers unique benefits to consumers and retailers-alike thanks to a very different philosophy of execution which is characterized by:
1. Innate understanding and pro-active management of Customer Needs and Wants;
2. Full and Complete control of Customer Experience;
3. Complete rethinking what your Product is. In my earlier blog, The Positive Trigger Upgrade Framework, I’ve defined Product in a manner congruent with this new understanding. The product is NOT only a collection of features – it is a combination of features, pre-post buying experiences, TCO, understanding of real and perceived benefits.
Unfortunately, one cannot just stumble upon a new D2C model, unless you’re lucky to have Steve Jobs or Travis Kalanick working for you.
I suggest a different approach for the rest of us – to implement ML to discover leading categories most amenable to D2C and to model user expectations from the myriad of datasets buried at the bottom of the Data Lake.
Clearly, without AI it is neighed to impossible to discover these opportunities and then to create data-driven workflows to implement D2C.
Of course, the transformation is not trivial and it requires much more than a team of data scientist creating insightful data points.
Incumbents, by definition, can’t adapt – their business processes were designed around an older value prop.
They have a real challenge of discovering and reacting to new business opportunities. In addition, per my previous post, advocating a need for a separate Chief Data Officer function, most incumbents experience a significant organizational misalignment of data, technology, and processes.
To truly explore D2C opportunities, transformative retailers need to summon a new breed of internal and external talent – Growth Hackers.
Jointly, the focus should be to create a new form of retailer – Digitally Native Vertical Brand.
According to Andy Dunn, founder of Bonobos: “It is not e-commerce, it’s vertical commerce. The product gross margins are at least double that of e-commerce (e.g. 65% versus 30%). The contribution margins can be 4x –5x higher (e.g. 40–50%versus 10%). The digitally-native vertical brand (DNVB) is maniacally focused on customer experience. The digitally-native vertical brand drives a lot more customer intimacy than it’s competition. The data is better because every transaction and interaction is captured. It’s one CRM. It’s one store, where everybody knows your name; Deeper data on the consumer drives enables the DNVB to stay closer to the customer than its brick and mortar have driven peers, and the ownership of the brand end-to-end fuels more affinity for a vertical commerce brand than even the best e-commerce experiences; While born digitally, the DNVB need not end up digital-only.”
Final Thoughts on Enterprise AI
Adoption of AI within the enterprise is not a choice but a given. Without it, no company can sustain a long-term advantage against a competitor that fully embraces AI. This is true for Boost.AI delivering incremental improvements to the current business model, but even more so for disrupted retailers seeking to transform into new business models.
Strategic transformation is not purely a technical challenge. It never is.
What is needed is a complete rethink and re-alignment of organizational lines and technology objectives, and aggressive efforts to operationalize Big Data platforms, AI, internal SMEs, and external growth hackers.
This is a very exciting, ever-evolving topic, and it deserves its own post.
Suffice to say that without rethinking how business, technology, and data aligned today, Prescriptive Business Intelligence that underpins future opportunities for Digitally Native D2C retailers, will remain elusive.
This is great article!
[…] Enterprise AI: It’s not a crutch, it is a bridge […]