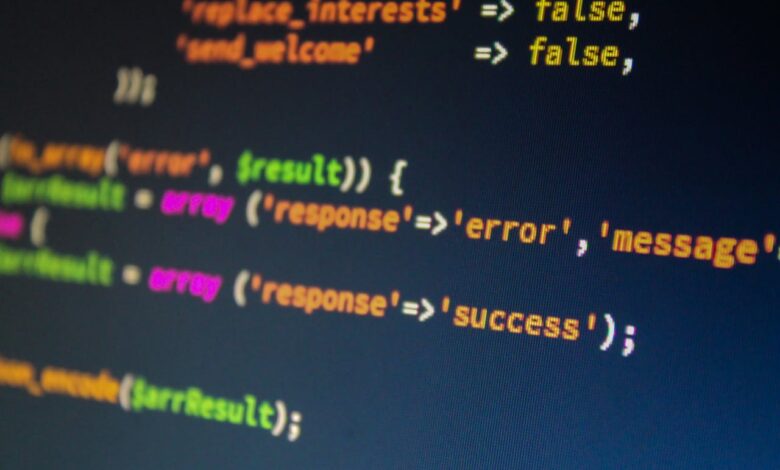
Sam Altman predicts that 2025 is the year where AI will step up to work alongside us. But is bringing AI into the workforce like hiring a bunch of interns – eager but unpredictable – or a bunch of geniuses?
Let’s dive into some of the realities of hiring AI workers versus virtual workers, focusing on holidays, training, sick leave, and pay.
“AI workers don’t need holidays”
The lack of down time is often touted as a big plus of virtual workers. We even hear it from business leaders, “AI doesn’t need to sleep, it can keep going when real workers, can’t” And sure, there’s a kernel of truth there. AI doesn’t book flights to Ibiza and clock off for two weeks, but it does require plenty of maintenance and updates.
Think of system downtime as the AI equivalent of a holiday. Planned maintenance or unexpected outages can disrupt operations just like a human taking time off. The promise of 24/7 availability isn’t entirely true if your infrastructure isn’t up to par. According to a recent report by Splunk, unplanned downtime costs the world’s largest 2,000 companies a combined $400 billion per year, averaging $200 million per company. These costs stem from lost revenue, regulatory fines, and hidden costs like slower time to market and worsened brand reputations. So, while AI doesn’t need a beach break, ensuring its continuous operation requires significant investment and planning.
Beyond downtime, AI models also require continuous updates to stay relevant. Without regular fine-tuning, models risk deteriorating in accuracy, leading to outdated or incorrect outputs. The recent shift towards retrieval-augmented generation (RAG) is an attempt to combat this, but it still requires ongoing oversight. AI might not need a vacation, but it certainly doesn’t run itself.
“AI workers don’t get sick”
AI doesn’t catch the flu, but it often suffers from bugs and, surprisingly, hallucinations. An AI hallucination occurs when a model generates outputs that are nonsensical or incorrect, just like a human seeing things that aren’t there. For instance, in 2017, Microsoft’s AI chatbot Tay had to be shut down after it began generating offensive tweets less than a day after its launch.
In the same way as humans are susceptible to illnesses, different AI models have varying degrees of robustness. For instance, OpenAI, Anthropic, and Google are all racing to reduce model hallucinations, but even the best models remain unreliable at times. The challenge is not just in preventing errors but in detecting them before they cause harm. This makes monitoring and governance essential, an ongoing responsibility equivalent to managing employee performance.
“AI workers are a one-time investment”
Think hiring AI is a pay-once, benefit-forever deal? Not quite. The cost of deploying AI solutions isn’t set by a wide-open market; it’s influenced by major players like Sam Altman and the CEO of Google, Sundar Pichai. As AI models evolve, businesses may find themselves needing to invest in updates or entirely new systems to stay competitive. This ongoing investment can be likened to providing pay raises or professional development for human employees.
For example, ChatGPT recently launched a business-tier solution, and companies are already integrating AI co-pilots into their workflows. However, these tools are often tied to evolving licensing fees, cloud compute costs, and retraining requirements. The AI you implement today could be defunct in two years, requiring additional investment to maintain the same level of efficiency.
”AI workers don’t need training”
Just like humans, AI systems need proper training to perform effectively. This involves feeding large language models vast amounts of data to learn from. For example, developing exceptionally complex models may require at least 10 million labelled items. The quality and relevance of this data are crucial. Poor training data can lead to poor performance.
Grounding AI, which means linking abstract knowledge to real-world examples, improves its ability to produce better predictions and responses. Retrieval-augmented generation (RAG) is one-way companies are working to enhance AI accuracy, by allowing models to pull from verified external sources instead of relying purely on pre-trained data.
One thing’s for sure, training AI isn’t just a one-off task. As regulations tighten, companies must ensure compliance with data protection laws, bias mitigation requirements, and industry-specific standards. For instance, financial firms using AI must align with regulations like the EU AI Act, ensuring models are transparent and accountable. This means ongoing audits, governance, and retraining. Basically, a continuous investment in AI’s “education.”
Watch out for DeepSeek
DeepSeek is emerging as a serious contender in the AI space, potentially disrupting the dominance of OpenAI and Google. At the time of writing, DeepSeek is 96% cheaper than OpenAI and unlike its closed-source counterparts, DeepSeek’s open-source model gives businesses unprecedented flexibility to fine-tune AI for their specific needs. Open-source AI models have the potential to shift power away from tech giants, enabling enterprises to customize and deploy their own AI systems without relying on expensive API access.
However, open-source AI isn’t without its risks. While businesses gain more control, they also assume greater responsibility for security, bias management, and performance tuning. DeepSeek’s rise signals a broader shift in AI development, one where companies may increasingly prefer in-house AI solutions over external platforms.
Open-source models like DeepSeek could be the key to breaking up AI’s current monopoly, but businesses must be prepared to take on the additional burden of maintaining and securing these systems themselves, which is no easy feat.