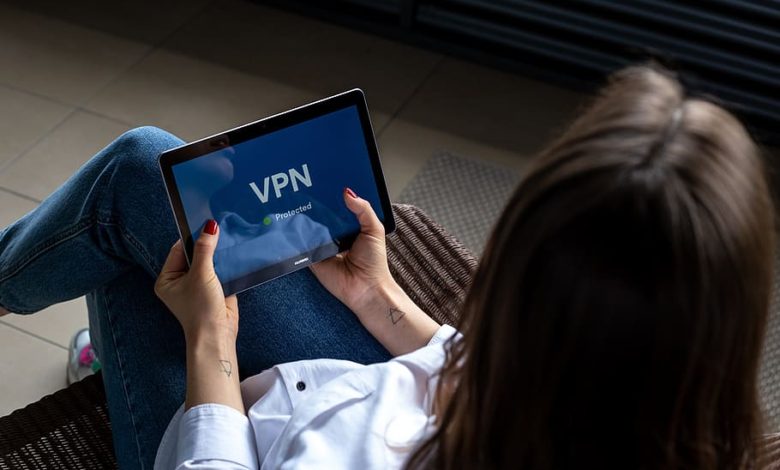
Digital identity is a rapidly evolving area that raises a host of issues around privacy, security, compliance, and inclusion.
Both the personal data attributes that go into creating a digital identity, and the metadata around them, provide fuel for sophisticated big data analytics run on machine learning systems.
Digital identity is also a technology domain where the judicious application of ethical AI can make for dramatic improvements in capabilities, but also where misapplication of AI can unintentionally produce the opposite effect.
We are going to explain in this article key concepts, issues, and frameworks for improvement.
What is digital identity?
A digital identity is an artificial construct, a digital representation of an entity with certain attributes that uniquely describe it.
If it’s a digital identity of a person, those attributes could be demographic data like age or gender, psychographic or behavioral data such as brand preferences or media viewing habits or financial actions such as purchase data, and other personal data like name, address, or unique government identifier.
Credit bureaus like Equifax and Experian create and sell identity profiles, as do marketing services firms like IPG, technology companies like Google, Facebook, and others.
Facebook infamously has constructed “shadow profiles” of people who aren’t users, but who they have been able to derive identities through other digital breadcrumbs.
This is troubling for several reasons, not the least of which is informed consent.
An actual Facebook user can correct information or opt-out or even “be forgotten” (if covered by regulation like GDPR), but if you don’t know that Facebook has a data profile on you, how can you request to opt-out?
Governments also craft digital identity databases, and some of them have begun compiling biometric data like fingerprints and facial scans. These machine learning-driving recognition systems are statistical in nature.
Individually, they might have an accuracy rate of 90% or so to identify an individual, although more sophisticated multimodal approaches can improve dramatically on that.
With advancement of artificial intelligence, particularly in applications with respect to digital identity and personal data, we are losing the anonymity of large numbers. In the old days, you could walk down the street in a large city and be assured that no one person could pick you out of a crowd.
Today, with CCTV, cellphone cameras, and deep learning-powered facial recognition systems, it’s possible to uniquely identify everyone in a crowd.
Even with masses of billions of credit card transactions, privacy researchers have determined that with even 4 points of supposedly-anonymized data, a person can be uniquely identified from millions of other people.
It’s a statistical model with a 94% accuracy rate, but it still shows the limits of privacy and some of the issues when we begin disseminating large volumes of data collected around people.
Issues with AI-driven Digital Identity
AI lets you derive not just data about people, but metadata.
But how is that being collected, secured, stored, and governed?
One common example of metadata derived from digital identities is the credit score.
Credit, at its heart, is an identity metadata attribute.
Using the variety of information accumulated about an individual, a probability is calculated of default. Old-style credit scores were notorious for inaccuracy and a “rearview mirror” capacity, making them of limited use for predicting future events.
The new AI models are intended to address deficiencies of the older linear-regression based FICO credit scores.
However, new, AI-driven models around behavior very rapidly can teach themselves to discriminate against certain protected classes. For example, the new Apple/Goldman Sachs credit card product got bad press for reportedly giving women lower credit lines than men. And these models still are susceptible to identity theft and synthetic identity theft, where someone can simulate your identity, obtain credit, and then ruin your credit score by defaulting, although new biometric identity and credit models much less so.
This issue of AI algorithmic discrimination has been arising with increasing frequency. Early facial recognition efforts, for example, trained the AI systems on pictures of people very much like the early-30s, white male engineers who built them.
A recent National Institute of Standards and Technology study found a high rate of false positives in facial recognition in one-on-one matching for Asians, African Americans, and native groups such as Native Americans and Pacific Islanders.
Many facial recognition systems have become really effective at recognising white people, including for facial biometrics, but effectiveness varies considerably system-to-system when recognising people of colour, depending on the training data set.
There are also issues around false positives and false negatives – the issue of single mode biometrics. People are under the false impression that because it’s done by a computer, it must be better – that the AI must be right.
But in fact, there’s an error rate. Facial recognition typically is only about 90% accurate “in the wild”, although can achieve higher rates under certain controlled conditions, and it can be spoofed or hacked.
A better framework for ID and identity
There are better alternatives for both constructing digital identities and the AI systems that help construct them. Below we will discuss three areas of opportunity:
Biometrics
We advocate strongly for multimodal biometrics, incorporating behavioral biometrics (both geospatial movement behaviors and biomechanical attributes), in lieu of any single mode such as fingerprint or facial recognition.
Multimodal biometrics are more robust, more secure, and can be made to be adaptive so that if you have an injury or something else that changes your profile, the system can adaptively adjust to your new behavioral characteristics.
It’s also much harder to forge or hack than either knowledge-based authentication (such as password or challenge questions) or single-mode biometrics (such as just fingerprint or just facial scan).
Distributed Systems
We believe that identity data is too sensitive to be accumulated in a giant honeypot for hackers to steal, and definitely should not be copied over and over again.
We advocate for an edge model, where more information is stored on the device and regenerated dynamically if you lose your device.
There are also proposals as to how to store critical biometric data in an encrypted form in a distributed database, which again would be more difficult to compromise than centrally stored information.
Responsible Innovation
Our frequent collaborator at Mastercard, Ajay Bhalla, the president of cyber and intelligence, talks about the imperative for “responsible innovation”.
As AI use in digital identity and personal data becomes more and more widespread, along with it comes calls for greater ethical thinking embedded into the very architecture of the AI systems.
Some researchers, such as Luciano Floridi and Joshua Cowls, have advocated for a common ethical architecture around how AI systems are designed and implemented, advocating for the integration of societal values at the early stages of AI development.
There are numerous other areas in which digital identity and AI systems could be improved, but these three areas offer high-impact starting points for ensuring that these AI-enabled systems work for the benefit of societies and for individuals within societies.
[…] such as Experian and Equifax create and sell identity profiles which creates ethical risks and considerations for businesses when using this […]