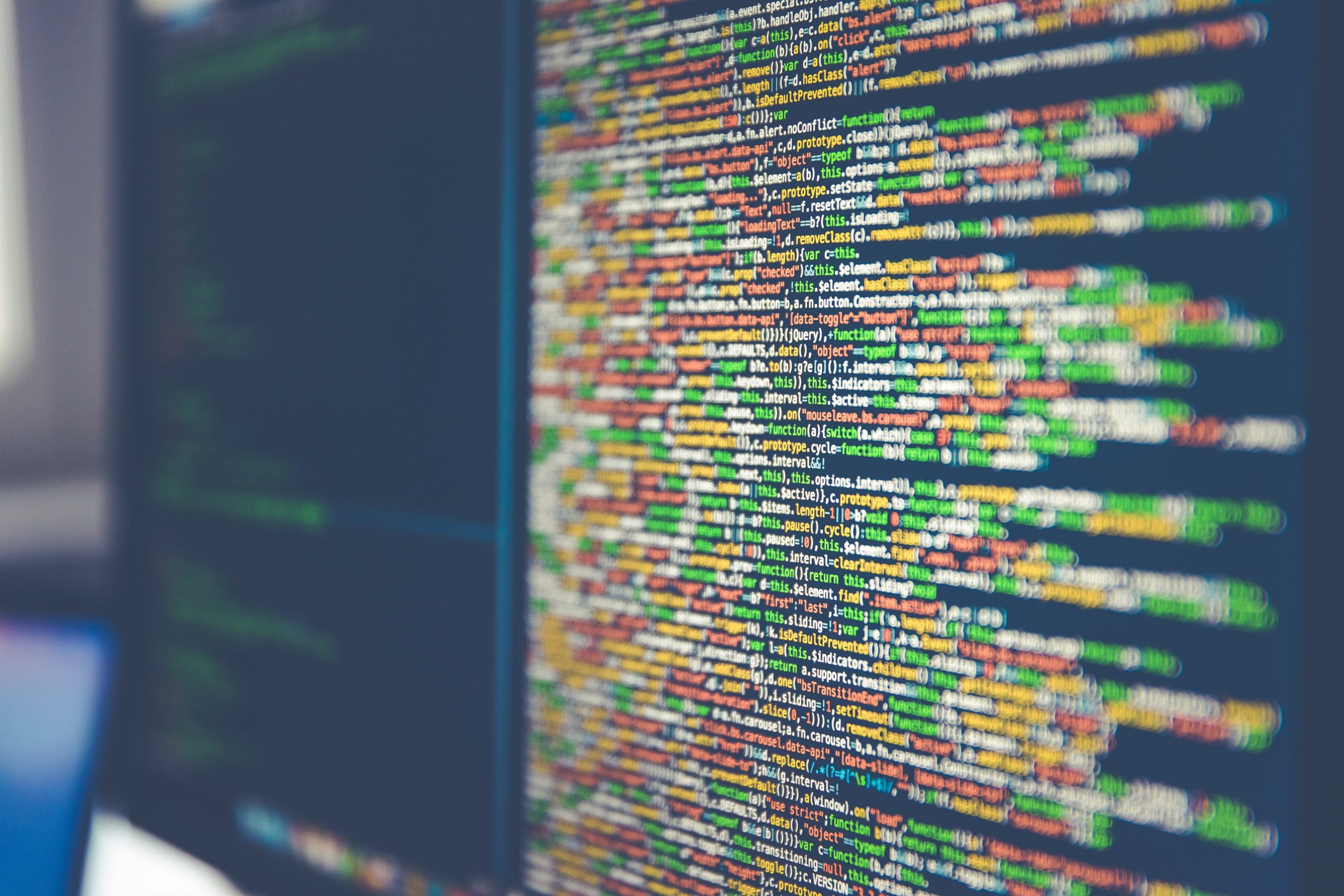
AI is a rapidly flourishing discipline in organizations today, driven by the advancement of AI development tools, the growth of available data and the clear opportunities that AI offers to capture value through more efficient operations, new products and services and better customer tailoring.
Moreover, the role of data scientist is currently one of the most in-demand jobs in the market. However, this enthusiasm for AI is being tempered and opportunities are being blocked by a harsh reality: not many machine learning (ML) models are making it to real-world use.
A survey conducted by the Bank of England suggests that while two-thirds of financial company respondents have adopted AI in some form, the median firm had just two live AI applications. Another survey from 2019 showed that only 13% of AI projects ever make it into production. This gap between development and deployment is known as the “AI chasm,” the unfortunate spot where most projects end up, never to be heard from again.
Why the AI chasm is so large: the problem of trustworthiness
There are multiple reasons for this AI chasm and some of them, such as the difficulty of reaching initial success in the lab, are already being addressed through improvements in development tools and training, as well as improved access to adequate data sources.
However, there is one major reason that many models don’t make it beyond the lab and into production, which deserves greater recognition – the problem of model trustworthiness. Even when a model is regarded as successful in the development and training phases, data scientists still often hit a wall when it’s time for the approval to get their model deployed.
This obstruction is often due to several factors:
- Data scientists or approvers aren’t confident about their model quality and accuracy, and lack the tools to be able to deeply and robustly analyze the model’s fitness and performance
- Data scientists often cannot fully explain to regulators or internal stakeholders how the model works
- There is no means by which to monitor models in production, which is necessary to ensure that they continue to work as intended, once deployed in the real world
Improving the odds of success: four key factors
Over the last year, my team and I have worked with dozens of major enterprises and data science teams to get a better understanding of how they can improve their success with AI and machine learning.
Success is measured, not just in terms of technical success i.e. creating models that work, but also in ensuring that those models achieve their organization’s goals, such as driving greater business value, improving customer satisfaction, or creating new markets.
Through our experience, we have established that there are four key approaches that can significantly help improve the odds of success with machine learning and get more models into live production:
1. Harnessing advocates from both technical and business leadership
The overall organizational goals of any AI project need to be well understood, and the technical scope of the project needs to be appropriate to these goals. Data science teams need to work closely with and have sponsorship from business leaders so that there is absolute clarity through all the development and approval phases on the project. The key to this is that internal stakeholders can voice their concerns early on, so that approval requirements are well understood and can be dealt with early.
2. Developing projects that support the organization’s core business model
There is often a temptation to experiment with a wide variety of projects to gain experience with tools or data, even when those projects do not necessarily impact the business in a meaningful way. Alternatively, there may be a desire to avoid the risk of failure on a project that has high visibility at an enterprise-wide level, out of concern that it could taint the data science team’s ability to do bigger projects in the future. However, this approach can often result in the opposite of the desired effect – instead of proving their mettle on small projects and working towards more significant projects, the data science team may be seen as a ‘mad scientist’ lab and a cost center with little practical value, thereby negatively influencing their perceived ability to work on more significant projects. On the other hand, those teams that tackle projects that have clear benefits to the business are more likely to have a faster path to getting necessary resources, achieving model success and securing approval from stakeholders for full deployment.
3. Proactively addressing AI risks to enable deployment at scale
Organizations that are further along the machine learning maturity curve have learned from experience that there needs to be a thoughtful, multiparty workflow regarding model testing and validation, approval, monitoring, and ongoing debugging. This approach creates higher-quality models that get deployed. It also adds efficiency across the model development and maintenance lifecycle, making the upfront effort and investment very worthwhile.
4. Viewing the building, deploying, and monitoring of ML models as both an art and a science
Although AI development is growing and the number of practitioners in the field is continually increasing, the development of ML models is still often viewed as a mysterious art form that is hard to evaluate and understand for those who are not data scientists. While it is true that it is a combination of expertise and experience that does create good models, this view often makes it very difficult for non-data science stakeholders to evaluate and trust the models created. In other words, model approval is often seen as risky and can be based on a personal judgment of the individuals who created it, not necessarily a judgment of the model itself. Successful data science teams adopt standardized data science practices and tools and make these core to explaining models and providing analyses so that that they clearly demonstrate a model’s effectiveness and appropriateness. In this way, trust is built into the models themselves, due to more transparency and better performance analytics.
The right tools to increase trust: focusing on AI Quality
In addition to following the above best practices when approaching ML projects, it’s imperative that data science teams are also equipped with the right tools to drive AI Quality. While there are many options to choose from in terms of ML model development, many AI stacks are still missing a critical component – AI Quality systems.
These provide core capabilities that enable data scientists to easily explain how their models work and can evaluate the fitness of any model and its associated data across multiple key dimensions (for example accuracy, reliability, stability, and fairness). Only when capabilities like these are in place, are a data science team well-equipped to overcome the key components of the AI chasm mentioned earlier, since they can:
- Deeply analyze model fitness and performance, and demonstrate that the model achieves its objectives
- Demonstrate that the model is fair, compliant, or non-discriminatory
- Show how the model works and indicate the key drivers of model results
- Monitor performance of the model while it is in production, thereby ensuring that it continues to meet its targets over time
AI Quality systems create the transparency and multi-stakeholder workflows that allow organizations to implement best practices in driving high-quality ML models. This in turn increases the trust in the models and accelerates the path to production approval and long-term model success. In all likelihood, AI Quality systems are the most critical components of the technical bridge needed to cross the AI chasm.