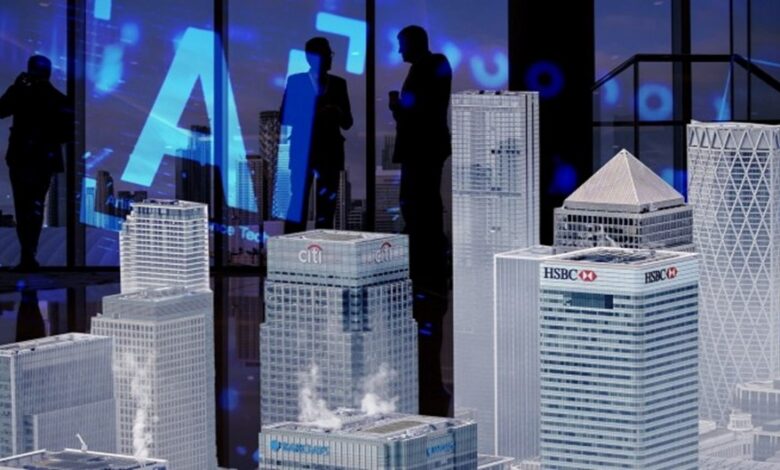
With the emergence of national and international AI regulations over the last couple of years, the pros and cons of regulating AI technologies have been hotly debated. For many, regulation is perceived as a tedious yet necessary measure to manage the applications of emerging technologies and prevent their misuse. Meanwhile, others argue that regulation stifles technological innovation and hinders AI adoption, particularly in highly regulated industries such as the financial services, healthcare, and the public sector.
In this article, we discuss the impact of regulation on AI adoption and innovation in financial services sector. While the high stakes of the financial services mean that AI adoption comes with greater risk, it also comes with the potential for greater gain – and financial organisations are not letting this opportunity pass them by. In Nvidia’s 2024 State of AI in Financial Services report, approximately 43% of financial organisations are currently using Generative AI, a rate of adoption which actually exceeds that of the retail industry, a less regulated sector which has a current rate of AI adoption of 42%.
This indicates that regulation is not necessarily the enemy of AI adoption. In fact, according to some experts in the financial services, existing regulation and established protocols in the financial services could actually be helping to facilitate AI adoption and foster innovation. This sets a hopeful precedent for the increasing AI governance expected to come into force over the next few years.
Below, we consider how regulation is driving AI adoption and innovation in the financial services industry, focusing on two key strategies: 1) the development of responsible AI, and 2) the creation of open-source standards for AI. These strategies are boosting investment, fuelling innovation, and increasing collaboration. This has positioned the financial services industry as a leader in AI adoption, challenging the perception that regulation is an obstacle to AI innovation, and illustrating some of the benefits that regulation can bring to organisations as they embrace the emerging technology.
The development of responsible AI
In the financial services industry, the inherent limitations and risks of Generative AI technology have been a significant barrier to its widespread adoption.
While many financial organisations have been using various forms of machine learning for several decades in backend operations, data aggregation, and fraud prevention, the industry as a whole has been slower to roll out customer-facing applications for the technology. These applications include AI-enabled chat agents, automated credit-scoring and loan approval services, and personalized banking services.
According to Asim Tewary, former Chief AI Officer at PayPal, this is because such applications are subject to far more regulatory scrutiny, as he explained at the 2024 MIT FinTech Conference, reported in a MIT article.
“You have to be able to explain why certain decisions were made — why a credit limit was set at that amount, for example. There’s an absoluteness that’s expected from regulators about being able to explain how the decision was made. Anytime you impact the consumer or introduce a system risk, regulators get very concerned.” – Asim Tewary, former Chief AI Officer at PayPal
In light of such requirements, the lack of transparency/explainability in the outputs of LLMs has been a significant obstacle to the adoption of Generative AI in the financial services, particularly in terms of validating the provenance of the data that LLM models are trained on.
Furthermore, the lack of transparency in LLMs has accentuated the risk of bias and/or hallucinations occurring, whereby GenAI models produce inaccurate responses to queries due to training on unreliable or biased datasets. In the context of financial services, these inaccuracies could lead to severe damages for both companies and their customers if left unchecked.
Yet even if the data used to train a GenAI model is not inaccurate or ‘biased’ according to standard evaluation, problematic outputs can still easily occur in financial services applications. For example, a PwC report highlights that an AI model could automatically reject a credit application on the basis of arbitrary factors that have a statistical correlation, such as a prospective applicant sharing the same surname as customers who have a high probability of insolvency.
In such cases, the problematic outcomes are not caused by biased or inaccurate datasets, but just the limitations of the technology. Unless specifically trained for a particular use-case, statistically-based data processing (which underpins all AI systems) typically lacks the sufficient nuances to differentiate between relevant and irrelevant factors for many more complex queries. Thus, AI models currently lack the capability to independently oversee several of the more complex tasks involved in highly regulated financial operations.
To reduce the likelihood of biased/inaccurate outcomes, and mitigate the risk of their potential occurrence, many financial services providers have already implemented (or started to implement) several measures to oversee the use of AI models:
- Human-in-the-loop systems: Retaining human oversight over AI systems is essential to ensure the ethical and regulated use of AI technology in financial applications, and to oversee the handling of more complicated cases that may require nuances in judgement that the AI model is not capable of.
- Domain-specific models: Fine-tuning LLMs is becoming an increasingly standard practice across industries. It is particularly important in the financial services industry due to the specific tasks and specialized protocols involved in its services.
- Federated learning: This refers to the training of AI models on a decentralized platform, reducing the need to transport data. This is crucial for the development of domain-specific models, and helps financial services to integrate specific data with powerful LLM models while remaining compliant with restrictions on sharing confidential financial information with external service providers.
- Confidential computing: This provides an extra secure cloud computing platform for the processing of highly sensitive data, or with greater compliance restrictions. By isolating the data in a protected CPU enclave, it provides exclusive access control for the company who owns the data, and means that even the cloud provider cannot access the data.
Federated learning and confidential computing are both ways of helping financial organisations meet standard regulatory requirements such as GDPR, as well as industry or location-specific ones such as the Fair Credit Reporting Act (FCRA), the California Consumer Privacy Act (CCPA), and the UK’s Financial Services and Markets Bill (FSMB).
According to Nvidia’s latest research, more than 12% of financial organisations are now assessing the use of one/both of these methods to improve their data security and privacy policies, despite the fact that these technologies have only emerged in the last few years. This indicates that increasing data security is a priority for financial organisations, driven by the regulatory requirements for this industry. Ultimately, this equates to a competitive advantage in the financial services, where enhanced data security goes a long way to winning customer trust and loyalty, as well as investment.
Developing responsible AI practices is therefore an essential practice for financial services providers, not only to ensure compliance with industry standards and local/global regulations, but also to foster trust among their customers, partners, and investors.
Case Study: KPMG
For KPMG, one of the ‘Big Four’ financial services companies in the world, trust is a crucial part of their vision to create a bold, fast, and responsible AI strategy. One of the first companies to roll out a regulatory framework for the development of AI in the financial services, KPMG illustrates both what the development of responsible AI looks like, and how regulation is helping to foster AI adoption and innovation.
In an interview with the AI Journal, KPMG’s Head of AI, David Rowlands, explained the company’s vision for developing responsible AI, acknowledging that this often means openly acknowledging and embracing the challenges that the emerging technologies bring.
“Our firm-wide strategy is a trust and growth strategy. We think you can only grow in professional services if you’re trusted – and if you want to be trusted, you have to step forward towards a problem. I believe that knowing the challenges involved is a really important part of this because you can’t just ignore them. If you say that there’s no risk, that there are no challenges, everyone knows you’re not being honest. Another really important benefit of this strategy is that it reassures customers that they’ve got a partner who’s really going to tackle the core problems, and face up to the reality of this emerging technology.” – David Rowlands, Head of AI at KPMG
Rowlands also pointed out that KPMG’s background in helping clients navigate various forms of risk management within the regulated environment of the financial services sector has placed the company in a strong position to navigate the new complexities that AI is bringing to the table in this sector. More generally, this is a key strength for many companies operating in highly-regulated industries such as the financial services, who are likely to have robust adoption strategies in already place to ensure comprehensive oversight for new technologies.
“With AI, you’ve essentially got this genie that isn’t going back in the bottle. Organisations like KPMG help organisations of many different industries with various forms of risk management, whether this is financial risk, credit risk, conduct risk, etc. We’ve helped clients process and manage these risk with greater transparency or more effective mitigation strategies. One of the things that AI is bringing to the fore is that this formula [of trust and growth] is becoming more and more pervasive across all organisations; you know, you can’t be in banking unless you can do both those things. You can’t be in health and life sciences unless you can do both of those things at the same time. We’ve been doing it for 150 years, often under the gaze of the regulator, helping organisations process risk and governance questions, whilst at the same time also helping them with their performance challenges. Now, with the pace that everything’s moving today, you can’t just put in all of the checks and balances and governance, and then start out on the AI journey. So what we’re helping our clients to do is to first experiment, and then from there build out the core capabilities of being trusted in AI at the same time.” – David Rowlands, Head of AI at KPMG
KPMG’s regulatory framework for responsible AI is based on the following 10 core capabilities, many of which are shared by other companies.
- Governance & accountability: establish clear accountability and oversight at the executive level for AI initiatives.
- Strategy & objectives: align AI initiatives with the organization’s strategic objectives and ensure they contribute to achieving business goals.
- Risk management: identify, assess, and manage risks associated with AI, including operational, compliance, and reputational risks.
- Data management: ensure high standards of data quality, accuracy, and integrity for AI models.
- Model development & validation: adopt best practices and standards for AI model development, including documentation and version control.
- Monitoring & reporting: implement continuous monitoring of AI systems to detect and address issues promptly.
- Ethics & bias management: establish and enforce ethical guidelines for AI development and use, promoting fairness, transparency, and accountability.
- Compliance & legal: ensure AI systems comply with all relevant laws, regulations, and industry standards.
- Transparency & explainability: ensure AI models are interpretable and their decision-making processes are transparent.
- Human-in-the-loop & change management: maintain human oversight in AI processes, ensuring critical decisions are reviewed by humans.
For Rowlands, taking the time to build out these core capabilities in a company is crucial to the development of responsible AI in more highly regulated sectors.
“Taking your time to put in place all of these 10 capabilities is crucial. If you as an organiser haven’t gone through building all of these 10 capabilities out, adopting AI can be a radical programme of change, that in certain sectors, I wouldn’t just leap into action on.” – David Rowlands, Head of AI at KPMG
Taking the time to invest in such measures sooner rather than later is especially wise given the coming implementation of the EU AI Act over the next couple of years, which will force all industries to oversee the use of AI at all stages of development and implementation.
A big advantage of emerging standardized regulatory frameworks such as the EU AI Act is that they will fuel investment in AI development, Rowlands points out. This could be particularly beneficial for companies leveraging AI for more unusual, bold, or risky use-cases. Without a regulatory compliance/risk framework, investors may not be confident enough (or even legally permitted in some cases) to invest in such applications for the technology.
“Regulation of AI is maturing, and it isn’t yet in place. This means that investors in regulated sectors have to work a lot harder in the absence of that regulation. But, when you look ahead at the coming regulations, such as the EU AI act, you can see the direction of travel. [The EU AI Act] is about a risk based framework – the riskier the situation that you’re using AI in, the more the demands on transparency, the higher the expense of regulation. It’ll get embedded into the development of AI solutions, it’ll get embedded into the governance over the top of them, it’ll get embedded into the ongoing review of these solutions. [This risk-based framework] is probably going to be the dominant form of regulation, and as it as it is put into place for AI, it’ll give people a lot more confidence.” – David Rowlands, Head of AI at KPMG
Open-source standards for AI
The creation of open-source standards for AI reflects an increasingly popular strategy for AI adoption and innovation in regulated sectors. Switzerland, for example, has just passed a new law mandating the use of open-source software in all public sector organisations, and for all new government code to be published under open-source licenses.
But before we delve further into the open-source strategy, first – what does open-source even really mean these days?
In the traditional sense, open-source means that all the code used to run a program is published freely online, and thus made accessible to the public and competing organisations. Back when software code was really the only intellectual property (IP) of the tech industry, the publishing of this code was as open-source as you could get.
However, with the development of Generative AI, and the foundational LLMs at its core, data has become the core IP for not just the tech industry, but nearly every business as they set out to adopt the vast potential of this technology in their operations. Today, data is recognised as one of the most valuable commodities of businesses and individuals alike, triggering numerous IP wars between the tech giants and copyright owners.
The rising prominence of data as a core aspect of IP, along with its key role in determining the performance of LLM models, has raised the question of how ‘open’ open-source software really is, given that most open-source AI models (such as Meta’s Llama, Google’s Bert, and GPT-Neo/J) have published the algorithms used to train the model, but not the data it was trained on. This has given rise to the concept of ‘open data’ to differentiate between the sharing of data for model training, and the sharing of code/algorithms.
Due to privacy and security restrictions, the sharing of data used in model training (particularly in fine-tuning) is just not possible in many more regulated industries. For example, while Switzerland also instigates an open data policy, which requires the publication of all data used and held by governmental organisations, this only applies if the data is non-personal and does not compromise security. This policy aims to promote innovation in AI by making it easier to access reliable data, while also making data governance more transparent.
More generally, open-source is a term that is coming to be used more generally to refer to a decentralized approach to technological innovation, typically involving open, accessible, and collaborative workflows. In the financial services, there are several emerging collaborative ‘open-source’ type initiatives that are aiming to foster AI innovation and adoption with this sector.
For example, FINOS is an open-source organisation under the LINUX Foundation, a non-for-profit decentralized innovation hub. It forms an open community of academic researchers, industry specialists, and technology developers cooperating on a range of collaborative projects to bring more accessible solutions to financial services businesses, helping them to adopt best practices for AI innovation and adoption in this industry.
To understand the benefits that open-source brings to the regulated financial services industry, we talked with Gabriele Columbro, Executive Director of the FinTech Open Source Foundation (FINOS), and General Manager of the LINUX Foundation, Europe.
“The financial services is an industry that can benefit a lot from open-source. For example, understanding that not every piece of software that they write is a competitive advantage and differentiator from competitors, can encourage organisations to collaborate, helping them to innovate faster and focus their investments and productivity on the important stuff. I would say that overall, open-source collaboration, where it makes sense, allows financial institutions to innovate faster and in a way that allows it to catch up with big tech.” – Gabriele Columbro, Executive Director of FINOS
Through collaboration and resource-sharing, FINOS also helps financial organisations navigate challenges from regulatory compliance requirements to talent shortages to applications for the technology itself.
“There are areas where it just simply makes sense to collaborate and have a shared innovation strategy. Regulation is the one that always comes to mind. All financial services organisations have to interpret that, they all have the same requirements because they all have to comply with the regulations. It is a huge item in the bottom line for all of these organisations, so it is an area where it really makes sense to collaborate. In fact, we have pilots that we’re running right now to interpret regulations with the help of GenAI. There is also great work coming from academia in this space, although it might not be fully enterprise-ready. Academia is really looking for enterprise use cases to sort of grow their research efforts into something that can actually be used in production. On the other hand, financial institutions are in dire need of AI talent, so FINOS also acts as a sort of connector between the world of academia and the world of finance.” – Gabriele Columbro, Executive Director of FINOS
Columbro also highlighted the commercial benefits of open-source collaboration for individual businesses and the commercial expansion of financial markets. Such benefits are mostly clearly demonstrated by Musk’s removal of Tesla’s patents for electric car technology in 2014. This was not so much a charitable move as it was a strategic one that expanded the commercial markets for electric vehicles.
“An open collaboration, whether it be software or standard, is there to drive the goals of individual businesses, it is not open source for charity, or for open-source’s sake. Open source really fundamental to driving market expansion – if you think about what Google, Microsoft and Amazon do with open source, this is really about commoditizing a certain area of the business, or targeted commercial displacement.” – Gabriele Columbro, Executive Director of FINOS
Since launching in 2018, FINOS now supports over 50 open-source projects, and has over 80 member organisations, recently welcoming several new globally recognized organisations including DTCC, Intel, AWS, FactSet, and JSCC. This reflects a growing recognition of the value of shared standards and collaborative initiatives, both in highly regulated sectors such as financial services industry, and more generally as businesses prepare for the implementation of the EU AI Act.
Along with the adoption of open-source and open-data governmental policies in European countries such as Switzerland, it looks like open-source standards will emerge to play a key role in the future of AI. Not only are they helping to accelerate AI innovation and adoption, but they are also contributing to the development mainstream industry standards and regulations that will help usher in a more sustainable, responsible, and democratized technological landscape.