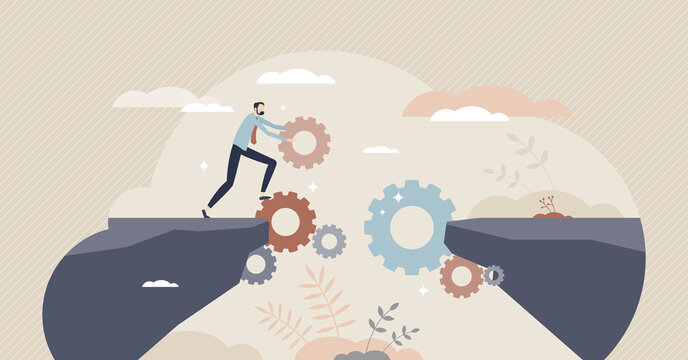
In the fast-paced world of artificial intelligence (AI), a critical challenge persists the disconnect between data science metrics and real-world business impact.
The root of the problem is data science metrics don’t consider the impact of an error. Imagine a marketing use case where we are trying to predict whether a potential customer needs a promotional offer to make a purchase.
Let’s delve into the complexities of AI evaluation and propose a shift in how we measure AI success to solve this problem.
The Disconnect Between AI Metrics and Business Impact
AI has long promised to revolutionize business operations, but there’s a fundamental problem: the metrics used to evaluate AI models don’t translate directly into business impact. This disconnect can lead to situations where AI models with excellent data science metrics destroy economic value when implemented.
To understand this paradox, we need to recognize that traditional data science metrics focus on the accuracy of predictions without considering the real-world consequences of those predictions. It’s like judging a chef solely on how precisely they follow a recipe, without ever tasting the food.
Consider this: A model might be 95% accurate in predicting customer behavior, which sounds impressive. However, if the 5% of mistakes it makes are extremely costly, that model could be detrimental to the business. This is the crux of the disconnect – data science metrics don’t inherently account for the impact of errors.
A Real-World Example: AI in Marketing
Let’s dive into a concrete example to illustrate this disconnect. Imagine a marketing scenario where an AI model predicts whether a customer needs a promotion to make a purchase.
There are four possible outcomes:
- True Positive: The AI correctly predicts the customer needs a promotion to buy.
Business Impact: Revenue from the sale, minus the cost of the promotion.
- True Negative: The AI correctly predicts the customer will buy without a promotion.
Business Impact: Full revenue from the sale, no promotion cost.
- False Negative: The AI incorrectly predicts no promotion is needed, and the customer doesn’t buy.
Business Impact: Loss of potential sale and future customer value.
- False Positive: The AI incorrectly predicts a promotion is needed when it isn’t.
Business Impact: Unnecessary promotion cost, reducing profit margin.
Traditional data science metrics like accuracy, precision, and recall don’t consider the very different business impacts of the different types of correct states and errors. They only care about whether the prediction was right or wrong, not the business consequences of each scenario.
But from a business perspective, these outcomes are far from equal. A false negative might cost a company a valuable customer, while a false positive might only marginally impact profit on a single sale. The AI model with the best data science metrics might not be the one that generates the most business value.
Different Stakeholder Perspectives
To complicate matters further, different stakeholders within a business may evaluate the same AI model’s performance very differently. Let’s extend our marketing example to illustrate this:
- Marketing Department: Focuses on maximizing sales while efficiently using the promotion budget. They might prefer a model that minimizes false negatives (missed sales opportunities) even if it results in more false positives (unnecessary promotions).
- Supply Chain Team: Evaluates models based on their ability to predict demand accurately. They might prefer a model that balances false positives and negatives to avoid both stockouts and excess inventory.
- Finance Group: Focuses on overall financial performance. They might prefer a model that optimizes profitability, balancing the cost of promotions against potential revenue increases, while also considering the impact on inventory carrying costs.
Each stakeholder’s unique constraints and priorities affect how they value the AI’s predictions. An AI model that the marketing team loves might give the supply chain team nightmares, and vice versa.
This divergence in perspectives highlights another limitation of standard data science metrics – they don’t account for the multifaceted nature of business decision-making.
From Traditional AI to Generative AI: The Evolution of Challenges
As the field of AI rapidly evolves, we’ve seen a significant shift towards Generative AI (GenAI) in recent years. While traditional AI models focus on tasks like classification and prediction, GenAI can create new content, from text and images to code and music. This shift has brought exciting possibilities, but it has also introduced new dimensions to the challenge of aligning AI metrics with business impact.
The fundamental disconnect we’ve discussed in traditional AI persists in the world of GenAI, but with added complexity. Let’s explore how the landscape changes as we move into the realm of GenAI.
The Generative AI Conundrum
GenAI models like Gemini 1.5, GPT-4, Mistral7B, and their successors have captured public imagination and are being rapidly adopted across industries. However, evaluating these models presents unique challenges that echo and amplify the issues we’ve seen with traditional AI.
- Evaluation Metrics Mismatch:
Popular data science metrics for evaluating GenAI, such as ROUGE-L and METEOR, focus on how closely the AI’s output matches a predetermined “golden answer.” However, these metrics often fail to capture the actual accuracy, relevance, or usefulness of the AI’s response.
Let’s break this down with an example:
Question: “Does the United States have the highest GDP in the world?” Golden Answer: “Yes, the USA has the highest GDP in the world.”
· AI Response 1: “Yes”
o This is correct and concise but would score low on ROUGE-L (0.18) and even worse on METEOR (0.05).
· AI Response 2: “The United States does not have the highest economic output of any nation in the world.”
o This is incorrect but would score significantly higher on ROUGE-L (0.38) and METEOR (0.39).
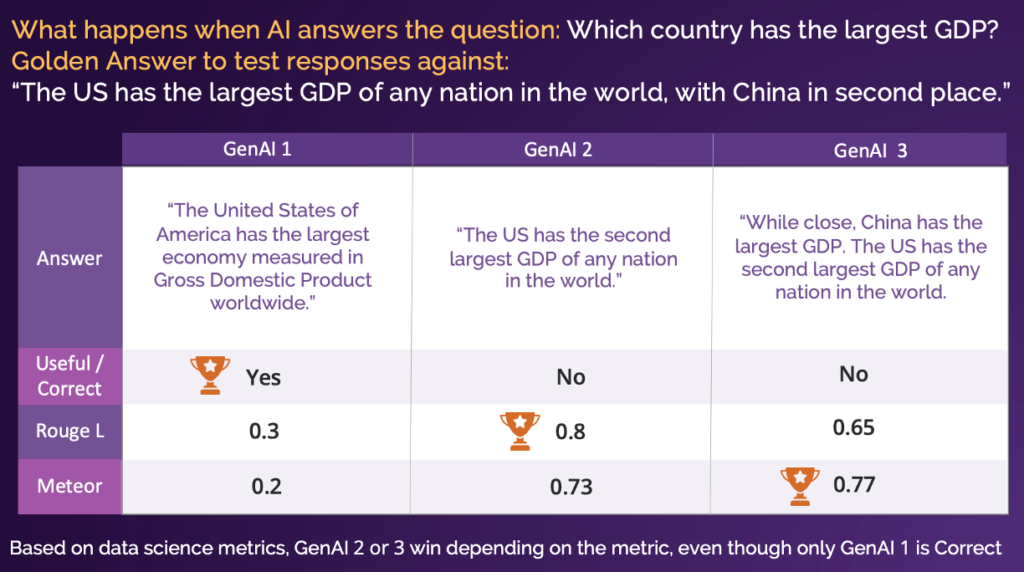
This example illustrates a critical flaw in current GenAI evaluation methods. They can favor verbose, inaccurate responses over concise, correct ones, simply because the longer response shares more words with the “golden answer.”
- Creativity vs. Accuracy: Unlike traditional AI, GenAI models are often valued for their creativity and ability to generate novel content. However, measuring creativity is inherently subjective and can be at odds with traditional metrics of accuracy or relevance.
- Context and Relevance: GenAI models can generate responses that are grammatically correct and semantically coherent, but completely irrelevant to the business context. Traditional metrics might not capture this lack of relevance, leading to high scores for responses that provide no business value.
- Ethical Considerations: GenAI models can inadvertently generate biased, offensive, or factually incorrect content. Evaluating models on their ability to avoid these pitfalls is crucial but not easily quantifiable in traditional metrics.
- User Preferences and Expectations: The output of GenAI models often interfaces directly with end-users. User satisfaction becomes a critical metric, but it’s highly subjective and can vary widely based on individual preferences and contexts.
- Consistency and Brand Alignment: For businesses, it’s not just about generating good content, but content that consistently aligns with brand voice and values. Evaluating GenAI models on their ability to maintain this consistency adds another layer of complexity to the assessment process.
- Integration with Existing Systems: The true value of GenAI in a business context often comes from its integration with existing systems and workflows. Evaluating this integration and its impact on overall business processes goes far beyond traditional AI metrics.
Moving Forward: Aligning AI with Business Goals
To bridge the gap between AI metrics and business impact, we need a fundamental shift in how we approach AI development and evaluation. Here are key strategies to consider:
- Develop Business-Centric Metrics: Create new evaluation methods that directly measure the business impact of AI models. This might involve simulating the model’s performance in realistic business scenarios and measuring outcomes in terms of revenue, profit, or other relevant KPIs.
- Holistic Evaluation Framework: Implement a framework that considers multiple stakeholder perspectives when evaluating AI models. This could involve weighted scoring systems that balance different business priorities.
- Contextual Testing: Test AI models in varied business contexts to ensure they perform well across different scenarios and can adapt to changing business conditions.
- User-Centric Design for GenAI: For generative AI, develop evaluation methods that consider factors like accuracy, relevance, and user preferences, not just similarity to a predefined answer.
- Continuous Feedback Loop: Implement systems for ongoing monitoring and adjustment of AI models based on real-world user feedback and changing business needs.
- Cross-Functional Collaboration: Foster closer collaboration between data scientists, business leaders, and end-users throughout the AI development and implementation process.
Future Implications:
As AI continues to integrate into core business processes, addressing this misalignment will become increasingly crucial. We can expect to see:
- More sophisticated AI models that can balance multiple business objectives simultaneously.
- A shift in data science education and practice to emphasize the business impact and end-user preference alongside technical proficiency.
- The emergence of new roles bridging the gap between data science and business strategy.
- AI systems that can explain their decision-making process in business terms, enhancing trust and adoption.
The disconnect between AI metrics and business impact is not just a technical problem – it’s a fundamental challenge that needs to be addressed for AI to truly deliver on its promise in the business world. By recognizing this disconnect and taking steps to bridge it, we can unlock the true potential of AI as a transformative force in business.
As we move forward, the most successful organizations will be those that can effectively align their AI initiatives with their business goals and end-user preferences, creating systems that not only perform well on technical metrics but deliver real, measurable value to the bottom line.