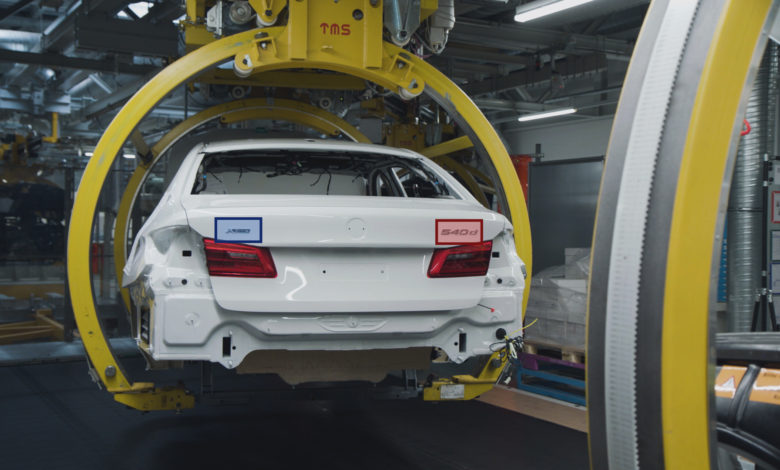
The BMW Group has implemented several Artificial Intelligence (AI) solutions in the production system.
Especially in quality work, AI brings great advantages.
This article presents the human-centered approach the BMW group follows when implementing AI solutions in production.
The continuous strive for absolute premium quality drives automobile manufacturers to invest significant effort in quality control.
Up to now, conventional computer vision methods have been used primarily for this purpose.
In addition, manual checks have been carried out to ensure quality – the so-called four-eye principle is used here.
Automated quality inspections are mainly carried out in so-called camera portals.
These camera booths work with pixel-based target-actual comparisons of photos.
The requirements for constant basic conditions are very high: The positioning of each vehicle and the lighting must always be exactly the same.
Such traditional camera portals are comparatively error-prone and result in fixed points in production, which undermine the principles of lean production.
Manual inspections turn out to be tiring, non-value-adding activities.
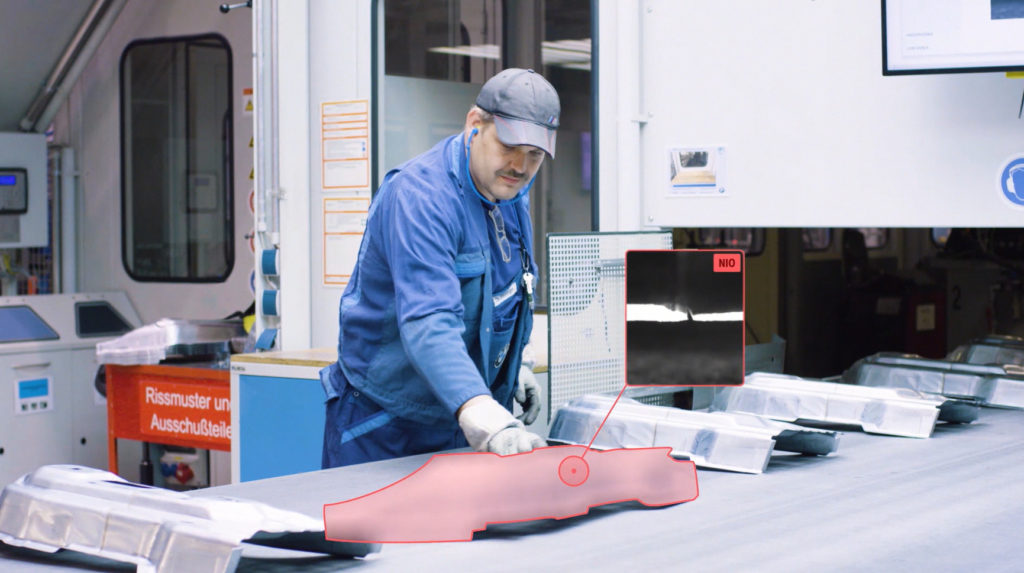
Artificial intelligence, AI, is one way to overcome these insufficiencies in quality work.
The advantages of artificial intelligence manifest themselves in particularly robust and very flexible solutions – for example, for optical inspections in the production system.
Neural networks enable computers to learn to extract certain features from photos.
In production, for example, this can mean that a neural network is able to determine the type plate, as can be seen in this video.
The corresponding press release presents further information on the application of AI in the BMW production system.
The advantages of almost arbitrary positioning, any illumination, and the robustness against other parameter variations become obvious.
Behind these neural networks lies the technology of Deep Learning – a method of Artificial Intelligence.
The following aspects proved to be elementary for a successful organizational and technical integration of these new methods during the implementation in the BMW production system:
Transfer Learning
For applications in production, it has proven to be useful to use so-called pre-trained neural networks and to adapt them to the particular application.
This technology of “transfer learning” offers the advantage that the required effort is comparatively low: In this case, an initial data set of photos is required to train a specific neural network.
Since the basic architecture of the network already exists and is quasi adapted, use cases can be successfully realized based on a few hundred to about 1,000 photos.
This approach results in a significant gain in efficiency: For the complete training of a new network from scratch hundreds of thousands or millions of initial photos would be needed.
Cyclical optimisation of neural networks
The training phase for the creation of a specific neural network in supervised learning always consists of the aggregation of the image data set and the subsequent labelling of these images (the addition of meta-information to the data).
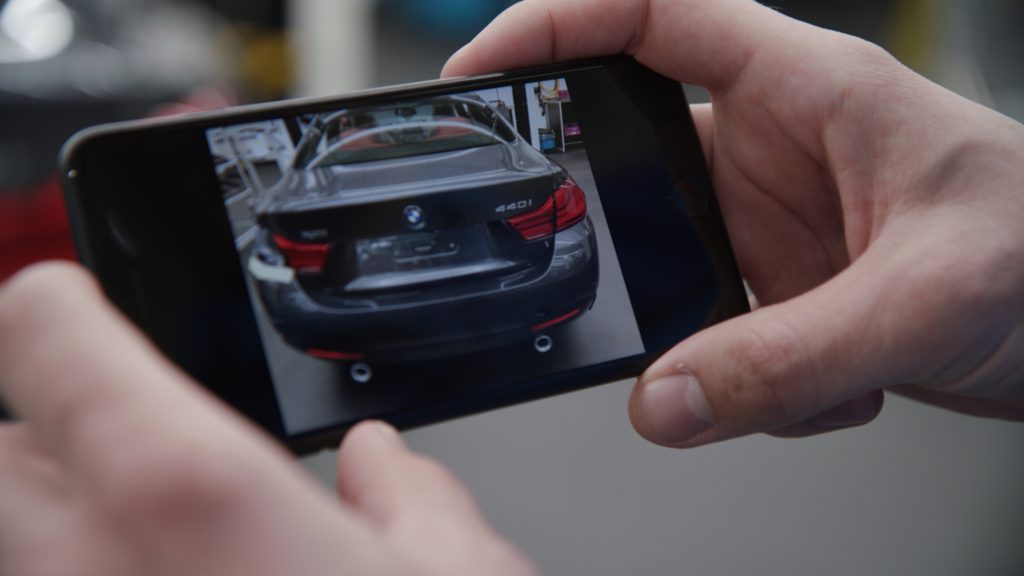
The labeled training data set serves to optimise the neural network until it achieves good results on the training data set.
In such AI applications, it is of eminent importance to convey to users whose main field of activity lies outside of computer science or mathematics that the described optimisation can be carried out in a multi-stage process.
Thus, for very few applications there is a priori certainty about how many photos are initially required to achieve sufficient robustness of the neural network.
Therefore, a test for unknown (new) data has to be performed before using AI.
If the performance on this test data – according to whichever metric – is not sufficient, further photos have to be taken and the optimisation of the neural network has to be run through once again.
System integration of the information technology
The AI applications successfully implemented in practice show that it is possible to create a high-performance AI easily and quickly for countless production engineering applications – photos including the corresponding labels are required.
Practice also shows that much more effort has to be invested in the integration of IT peripherals.
For example, it is vital to retrieving the order information for each personalised vehicle, i.e. the concrete individual configuration of the respective product.
Likewise, the information on the quality audit must be stored in a database and saved for the long term.
These integration tasks should not be underestimated, especially when dealing with an information technology brownfield.
User-friendliness for the established quality expert
Eventually, technologically innovative solutions such as the AI systems presented above will find their way into production once the production workers see the added value of the innovation and are able to operate the system.
This effect, in particular, is highly relevant.
Basically, the BMW Group’s mantra is that people are the focus of interest.
In concrete terms, in the production system, this means that all AI systems for object detection have been designed and developed in such a way that they do not have to be operated by IT experts, but that the production experts can easily operate these innovative systems – without any need for training.
This aspect was decisive for every implementation and resulted in a high proportion of in-house work in the IT implementation.
The tasks involved in modelling an AI solution for quality inspection, therefore, include taking training photos and labelling them. This also includes the step of the acceptance of an AI solution.
This means that the production or quality specialist himself is able to intuitively set up his AI solution for his specific production task using photos and the labeling of these photos.
It is explicitly his task to evaluate the resulting AI model and inspect it during the running operation as detailed in the video below.
Summary
AI can help to significantly increase the robustness and flexibility of optical quality inspection systems.
In the production system, the BMW Group is focusing on the organisational integration of this technology.
AI is made operable in an intuitive way for every production employee so that he can create his application for exactly his individual use case.
The focus is on this self-service idea – the goal is not to create thousands of data scientists in production but to bring AI as a possible new tool to the people on the shop floor.
[…] How the BMW Group involves the production staff into the implementation of Artificial Intelligence i… […]