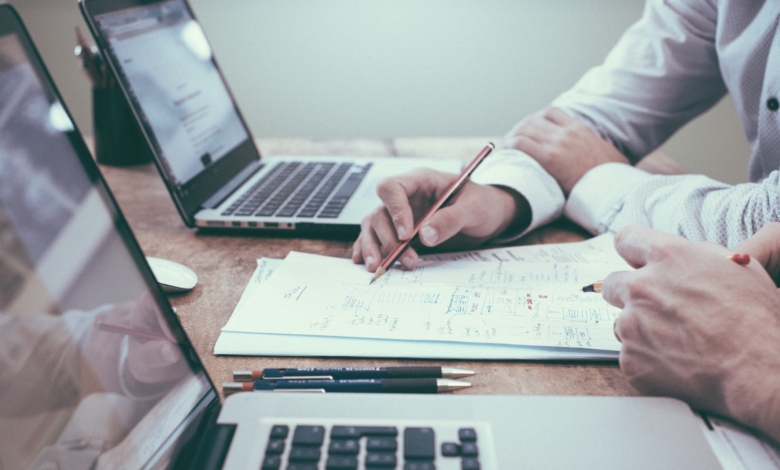
AI is being implemented into almost every facet of the business, across industries, increasing efficiencies and saving time and resources. However, recently the ethics of AI have been called into question, especially surrounding the potential for AI bias. When high-profile cases of racist and sexist AI systems came to the public attention, business reputations were damaged. There were red faces across the board, from Amazon and its CV-sifting software to America’s prison service and its COMPAS software.
AI is too valuable to abandon. As a labour-saving productivity tool, it is becoming increasingly useful (Microsoft seems to think so with its investment in OpenAI) and AI spending across all sectors is expected to more than double by 2025. However, unless the problem of AI bias is actively addressed, it could become the greatest threat so far to workplace DE&I.
What went wrong with AI and bias?
In the early stages of AI development and adoption, one of the technology’s major selling points was that computers can’t form opinions. While they can be programmed to learn and make decisions based upon the trends of the enormous swathes of data entered into them, they can’t actually take a personal dislike to someone. So, a person’s skin colour, gender, age, or sexual preferences shouldn’t, theoretically, sway any decisions reached by AI programmes.
Unfortunately, what wasn’t taken into account was the fact that the data initially used to train AI programmes could be biased. The data used to train AI models is based on historically labelled data eg CVs and the decision reached on hiring: In this way, the biases of the people who initially labelled the data unintentionally influence any decisions the model is capable of reaching. This bias already exists in rules-based systems (eg in home insurance, where your postcode counts for everything). Due to the need to have independent human verification of the initial data selected for training, removing that human element from AI models is not possible. So, what does this mean for DE&I?
How does biased AI impact DE&I?
The fact that the capabilities of AI are advancing hugely has been broadly celebrated. Deployed in an increasing array of scenarios and arenas, it can make startlingly fast ‘informed’ decisions that are changing the way in which a range of sectors – security, manufacturing, healthcare – work.
For example, AI has become so advanced that machines are now capable of diagnosing cancer with a high degree of accuracy, trained on images and medical outcomes. However, this technology can do something that no human doctor can do – predict a person’s race-based merely on an x-ray. In this case, that outcome might be benign, but it opens up a significant question as to what personal and profiling data can be gleaned from seemingly anonymous and innocuous data. Unless we find a way to remove this potential for bias, the repercussions for DE&I and the potential for accidental discrimination could be high.
What do we need to do to tackle AI bias?
Right now, there is no way to train AI models without the use of human-labelled data. There are approaches such as self-supervised learning which reduces the need for this labelling, but it does not remove the need completely. So we have to take steps to understand where the bias is in our data and look to remove it.
Explainable AI (XAI) allows us to determine how software reaches any given decision. Was this person really the best fit for the job, or were they only the white and male applicants? By creating a system that allows each decision to be investigated and the decision-making process reasoned through, we can definitively answer that question every time. This way, we can look to see which elements of the training data unduly influenced the decision, and amend the item or remove it from the training data. We can retrain systems on a case-by-case basis – correcting the data and the methodology – to enable the eventual removal of unintended bias and abuse.
Explainable AI goes beyond system repair and enables justification of decisions on an individual level, a fundamental requirement of legislation like the UK and EU General Data Protection Regulations (GPDR). If AI is used to determine pay increases, promotions, or recruitment, individual requests for feedback can be honestly responded to. If mistakes were made – if a person’s gender or skin colour or anything else not strictly related to performance influenced the outcome of the automated process – reparation can be made, thus restoring faith and trust. Equally, however, if the decision was based upon genuine factors, such as performance, managers are equipped with the data they need to explain why the decision was made. This will help to guide the individual concerned towards more satisfactory results in the future, creating a workplace based on openness and trust.
We are becoming increasingly reliant upon AI for a whole range of labour-intensive processes, and we are still a long way from uncovering its full potential. As our dependence on AI grows, it’s imperative that we ensure it is used correctly and ethically and this can only be achieved if debiasing remains a key priority.