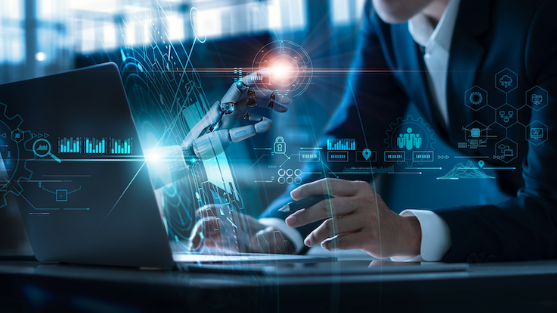
The financial industry is known for its reliance on vast datasets and complex algorithms to make decisions that affect millions of people. However, with the power of AI comes the responsibility to ensure that these models are fair, accurate, reliable and free from bias.
Rumman Chowdhury, Twitter’s former head of machine learning ethics, highlighted the impact of algorithmic discrimination during a Money20/20 panel in Amsterdam. She pointed out that AI models trained on data influenced by racial demographics can perpetuate bias.
For instance, she referenced 1930s Chicago, where “redlining” defined creditworthiness based on geographical areas of ethnic and racial minorities.
“Fast forward a few decades later, and you are developing algorithms to determine the riskiness of different districts and individuals. And while you may not include the data point of someone’s race, it is implicitly picked up,” Chowdhury said.
Biases of the past vs AI models of the present
AI models can also exhibit biases based on age, gender, or geographic location. This can lead to unfair outcomes, such as discriminatory lending practices or unequal employment opportunities.
For instance, women have historically entered the labor market later than men, particularly for STEM roles, meaning that AI models trained on older data may not adequately represent female candidates.
A classic example is the case of Amazon‘s AI recruiting tool, which relied on 10 year data prior to 2014. During that period, the number of female candidates applying for tech jobs was significantly lower than today.
As a result, the AI model, having been trained on this data, developed a bias toward male candidates, often selecting them as better fits for roles simply because the input data suggested so. This is a reminder that historical data can embed the societal biases of the past into the AI models of the present.
To mitigate this risk, it’s essential to ensure that training datasets are diverse and representative of the current population. This requires a concerted effort to include equal proportions of different groups, such as males and females, and to focus on the relevant skills and attributes that truly determine an individual’s suitability for a role or financial product.
The demand for high-quality training datasets is skyrocketing. According to a recent report from The Brainy Insights, the global AI training dataset market is set to expand from $1.64 billion in 2023 to a staggering $14.42 billion by 2033, boasting a compound annual growth rate (CAGR) of 24.25%.
Training your AI dragon: Critical concerns to address
In financial institutions, models are frequently used for regulatory and risk management purposes. They are also leveraged for credit decisioning and credit scoring, specifically in the lending markets. AI, at its core, is a model, and needs to be managed for model risk among other risks.
When evaluating AI models in banking, we focus on several key areas:
- Data Quality: The quality of training data is assessed. Are there missing data points? Is the data relevant and appropriate for the portfolio in question?
- Methodology: The model’s methodology is examined to ensure whether it is standard and widely accepted in the industry. This includes reviewing whether the model uses linear regression, machine learning, or qualitative approaches, and determining if these methods are appropriate for the intended purpose.
- Model Design: Variable and feature selection is critically assessed. Do they make sense from both a business and quantitative perspective? Are they accurately predicting the outcomes they are supposed to?
- Model Stability: Model’s stability and performance metrics are evaluated. Does the model break through different periods of time? Are there any discrepancies in how the model predicts outcomes in different scenarios?
- AI specific risks: Specific tailored testing needs to be conducted to test AI/genAI applications. Tests should ensure the AI is robust, reliable, safe, secure, fair, private, explainable and involves human accountability.
Data privacy, fairness, and legal risks in lending
With regulations in place to ensure fair lending practices, any bias in AI models could lead to significant legal and ethical issues. Therefore, it’s crucial to conduct specific analyses to ensure that lending decisions are based on factors that truly indicate creditworthiness, rather than on demographic attributes like ethnicity, gender, or age.
Data privacy is another significant concern in AI applications, not just in banking but across industries. Right now, 18 states, including California, Virginia, and Colorado, have comprehensive data privacy laws in place. Six more states are in the process of passing similar legislation.
Banks handle sensitive information, such as Social Security numbers and financial records, making them prime targets for cybersecurity attacks. To mitigate these risks, some small-to-mid-sized banks are banning the use of sensitive information in AI training or tuning processes and are implementing strict policies to protect customer data.
A recent survey by Arizent, the publisher of American Banker, found that 30% of banks ban the use of generative AI tools. The research, which included 127 professionals from financial institutions of varying asset sizes, explored the evolving role of AI in the industry, examining its applications, risks, workforce impact, and more.
In the wake of recent reports on discrimination, IP infringement, and data privacy issues related to use of AI based technology, banks and other financial services institutions have to be educated on best practices for AI risk management and governance.
While many banks believe they are taking necessary precautions, the reality is that the rapid pace of AI adoption can outstrip their ability to manage risks effectively. Big banks are ahead of the curve in exploring generative AI, but they also recognize the need to establish strong policies to manage these technologies responsibly.
Addressing specific AI risks like fairness and bias requires a combination of rigorous testing, diverse data, and ongoing monitoring. By taking proactive steps to ensure that AI models are fair, accurate and reliable, banks can harness the power of technology while safeguarding against its potential pitfalls.