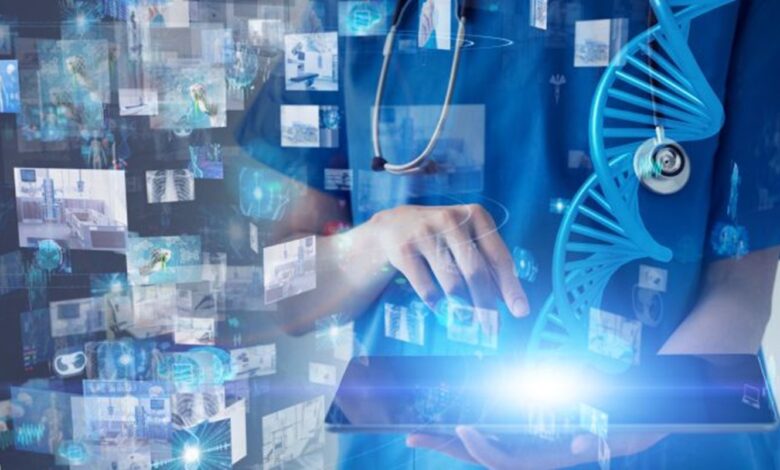
“AI has the potential to transform health on a planetary scale” ~ Karen DeSalvo, Google’s chief health officer, speaking at an annual company event in March, as reported in a Bloomberg article.
“Healthcare is probably the most impactful utility of generative AI that there will be” ~ Kimberly Powell, VP of healthcare at Nvidia, speaking at Nvidia’s latest AI Summit in Taipei, as quoted in an Al Jazeera article.
“Stanford Health Care’s deployment of DAX Copilot [will leverage] state-of-the-art AI from Nuance and Microsoft to empower its clinicians, expand care access for its diverse patient population, and train new physicians to deliver high-quality, personalized precision care.” ~Robert Dahdah, CVP of Global Healthcare and Life Sciences at Microsoft, commenting on the deployment of a new Generative AI Copilot, DAX, developed for healthcare by its subsidiary company, Nuance.
“Amazon aims to democratise AI capabilities for the healthcare industry – and ultimately make it easier for customers and patients to get and stay healthy. We believe we can make the healthcare experience easier for patients and providers, and we know generative AI will play a critical role in that.” ~ Sunita Mishra, Chief Medical Officer & Head of AI Clinical Innovation at Amazon Health Services, and Dan Sheeran, General Manager for Health Care and Life Sciences, at AWS, summarizing Amazon’s vision for AI in healthcare in a comment sent to Healthcare Digital.
“Our Telepathy cybernetic brain implant…allows you to control your phone and computer just by thinking.” ~ Elon Musk, CEO and founder of Neuralink, sharing the next steps for the company in an X post, following the successful implant of a brain chip in the first patient.
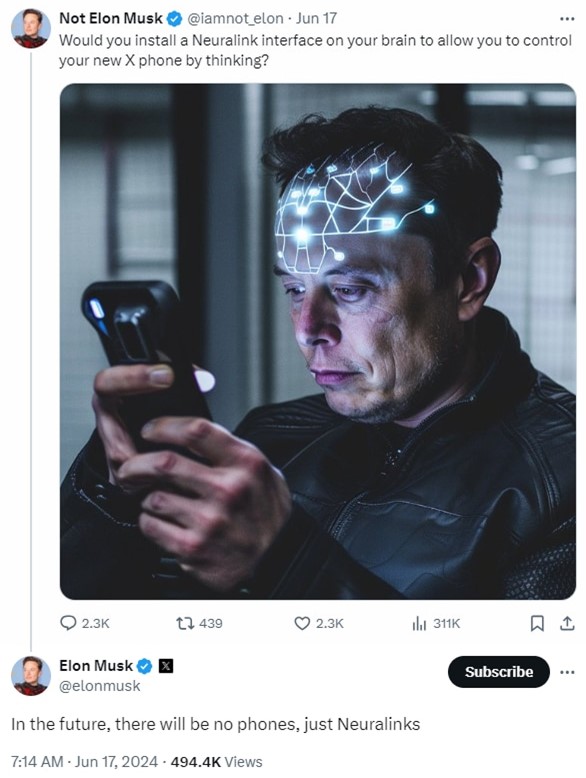
Above: Musk responds to a parody of himself on X, confirming that his ambitious vision for Neuralink’s AI-enabled Brain Chip Technology stretches beyond the vast reaches of traditional healthcare, to revolutionize the interface of human-technology interactions.
These comments highlight just some of the lofty ambitions harboured by big tech companies and tech tycoons when it comes to healthcare and biotech.
However, it remains to be seen whether these ambitions can realistically be pursued in the highly regulated sector of healthcare, where the stakes are as high as life or death and the commitment to ‘putting the patient first’ still reigns supreme.
Indeed, the track record of AI in healthcare is pretty rocky. While most of us have probably heard the major success stories, including Musk’s pioneering brain chip implant technology at Neuralink, and the ongoing innovation with AlphaFold technology at Google DeepMind, unfortunately these breakthroughs are few and far between.
For example, according to my own calculations based on publicly available information, the rate of success for AI healthcare projects at Google’s parent company, Alphabet Inc, would be approximately just 54% – and that’s counting projects still in development as successes.
This is no doubt why the company and its subsidiaries have dabbled in so many different areas of healthcare, as reporters Davey Alba & Ike Swetlitz savvily point out in a Bloomberg article, where they also list the company’s record of healthcare projects.
Nevertheless, as of 2023 data collected for an academic study published in mHealth Journal, Google (including its subsidiaries & parent company, Alphabet) is the tech giant that has shown the most interest in healthcare, leading with 12 collaborations with healthcare partners, followed by Microsoft with 9, Amazon/AWS with 6, IBM with 3, Nvidia with 2, and finally Apple and Samsung with just 1 each.
Of these 34 collaborations, 27 involved some use of AI, illustrating the high hopes for this powerful technology in the healthcare sector. Given that healthcare is also a trillion dollar industry, worth $4 trillion in the US alone, it is no wonder that several of the bigger tech companies are setting their sights on this industry as a comparatively untapped goldmine.
So given big tech’s clear incentive to invest in AI-driven healthcare, why is the success rate so low?
In a recent AI Journal poll, 50% of respondents voted that the main reason that tech giants struggle with healthcare projects is the complex regulations that rule the industry, followed by 38% who considered privacy concerns to be the main reason.
Andreas Cleve, CEO and Cofounder of AI healthcare Co-Pilot provider, Corti, similarly highlights regulatory compliance and lack of transparency surrounding the training data for Generative AI models as key issues that can hinder the successful deployment of AI in healthcare.
“While big tech’s ambitions in healthcare are grand, the reality of stringent regulatory and safety requirements, coupled with the use of generalized models, can limit the speed at which these innovations scale. Transparent and explainable AI, specifically trained on healthcare data, holds tremendous potential for enhancing clinical reasoning and building trust. Without this approach, progress may be slower, as widespread adoption depends heavily on establishing and maintaining trust within the healthcare community.” ~ Andreas Cleve, CEO and Co-founder of Corti
Interestingly, 13% of our poll respondents also voted that there was a perceived mismatch between AI technology and healthcare culture, but no respondents voted for the option that tech giants had misaligned incentives that would put them at odds with healthcare providers.
This highlights that while tech companies have commendable goals and aspirations for the healthcare industry, they may face difficulties in integrating with healthcare providers due to the different principles and working cultures that they each have. Indeed, while the tech industry tends to prioritize agility, innovation, and automation (particularly with Generative AI), the healthcare industry is typically characterized by more standardized procedures, a more cautious approach to innovation, and an emphasis on human touch and connection.
So when these two quite different industries come together to collaborate on AI-driven healthcare projects, it is perhaps no surprise that these differences could present a challenge to the real-life deployment of the tech industry’s ambitions for healthcare. Nevertheless, this is arguably where the greatest potential for transformation lies – in that sweet spot that balances tradition with innovation, caution with risk, and automation with human connection.
To find out more about what this sweet spot looks like, we spoke to representatives from two successful tech companies in healthcare, rehabilitation software provider, MiiCare, and Deep Medical, a leading UK company expanding healthcare capacity through the power of AI, to pick their brains on what the challenges of AI in healthcare are, and how they can be overcome.
We also feature exclusive insights from Andreas Cleve, CEO and Cofounder of AI healthcare Co-Pilot provider, Corti, and Dr Ameera Patel, MD PhD, and CEO at respiratory technology developer, Tidalsense, who share their thoughts on why healthcare has proven to be such an elusive area of success for big tech companies, and what some realistic ambitions for AI in healthcare could look like.
Failures & flops for AI in healthcare
- IBM’s Watson Oncology Expert Advisor project that aimed to provide diagnostic guidance in cancer care. Following the victory of IBM’s Watson supercomputer on TV game show Jeopardy! playing against two human competitors in 2011, the company decided to leverage its capabilities in the lucrative healthcare industry. In 2013, narrowing in on cancer, a particularly high-profile disease, the company formed collaborations with a number of healthcare partners, including Memorial Sloan Kettering, MD Anderson Cancer Center and the University of North Carolina School of Medicine. However, the actual deployment of the system was marred by a huge mismatch between ambition and suitable training data, leading to generic and limited treatment suggestions that were no real aid to physicians. So after just two years and $62 million in spending, MD Anderson terminated its partnership with IBM Watson, and many of its other healthcare partners have since followed suit.
- Google Health’s AI system for Diabetic Retinopathy in India and Thailand, where the number of eye specialists is limited. This project aimed to develop a digital screening process for the condition, which can lead to blindness if left untreated. However, due to problems with accessing quality data in real-life hospitals with sub-par resources and usability challenges for local health workers, the project failed to meet the anticipated results.
- Epic Systems’ Sepsis Prediction Model, which aimed to predict the development of Sepsis, a life-threatening disease, in already hospitalized patients. Medical reviews found that the accuracy of the model was too low to be trusted, and was in fact just propagating the some of the expectations of doctors, rather than providing unbiased prediction based on objective and reliable data. Falling trust in the model was also furthered by its lack of transparency and explainability, which was partly a result of its proprietary ownership.
- DeepMind Stream’s App, which aimed to help doctors and nurses monitor patients with acute kidney injury. However, despite some limited success in the actual monitoring, the project is widely regarded as an example of what not to do because it was later ruled to have violated data privacy regulations by the Information Commissioner’s Office, with the NHS sharing patient data without proper consent or sufficient transparency. This gave rise to significant backlash over privacy concerns, eroding trust in the app, the NHS, and the use of AI in healthcare more generally.
Why is there a high chance of failure for AI in healthcare?
In the cases of the projects mentioned above, the failure of the AI systems in healthcare essentially boils down to three core issues:
- Inaccuracy/insufficiency of data used to train AI models
- Potential incompatibility of AI with existing healthcare systems
- Lack of transparent and secure data collection
None of these issues are particularly unique to healthcare, but are instead considered key flaws of Generative AI models more generally.
Nevertheless, they present an especially significant challenge in healthcare due to the stringent regulations that are in place to protect patient data, and the human-centric and often multi-faceted nature of existing healthcare operations.
Below, we explore these core issues that seem to be at the heart of big tech’s failure to harness the power of AI in healthcare, and discuss whether (and how) they could best be mitigated.
Inaccuracy/insufficiency of data used to train AI models
In today’s world, predictive diagnostic tools for health conditions are in high demand, particularly as the notion of holistic health and wellbeing has become increasingly popularized.
In fact, scarcely a day goes by for many of us without some reminder of the impact of our lifestyle on our risk of developing this or that health issue later on in life – and we may even find ourselves consuming articles about lifestyle rituals associated with various health benefits are a consumed with almost the same gusto as the foods we are reminded to avoid.
But this newfound interest in holistic health and wellbeing also highlights one of the key challenges for AI systems in healthcare – this is their ability to ingest and process data on a more holistic level.
As the case of IBM’s Watson Oncology Advisor demonstrates, inaccurate or insufficient data is a significant challenge for AI in healthcare. This is particularly true of diagnostic applications of AI in healthcare, where the utility of the AI model is contingent on the accuracy and comprehensiveness of the data.
Indeed, it is extremely difficult to train AI models to comprehensively account for the complex interplay of genetic, environmental, and social factors that underlie many health conditions. Nevertheless, just like a good physician will sit down with a patient and try to really understand their problem within the greater context of their lifestyle and background, holistic data about the lifestyle of an individual is crucial to the accuracy of predictive AI models.
Without holistic data about an individual’s genetic make-up, diet, mental wellbeing, exercise levels, and daily routines, these models have far less accuracy in predicting the likelihood of the individual developing a particular condition, or the chances of success for a particular course of treatment.
Nevertheless, this type of more holistic data not only demands more time and energy-consuming model training, but it is also challenging to acquire in the first place.
In an interview with the AI Journal, George Kowalski, VP of Business Development at MiiCare, highlighted the issue of consent for data collection as one of the greatest challenges for AI in healthcare, pointing out, for example, the natural discomfort and vulnerability that patients experience under video surveillance and other similarly intrusive data collection methods.
“So in response to your question ‘what’s the biggest challenge for AI in healthcare’, I would say that even if you’ve got the best ever solution in the world (which we think we do because it saves lives) – even if it’s that good, you still have the massive barrier of consent that’s going to prevent your solution reaching its full theoretical potential. For example, there’s always going to be someone who thinks “Oh no! The AI has identified that I went to the toilet four times last night, and I know why it’s doing it – it’s because I’ve got a urinary tract infection. And even though the doctors and nurses and carers might need to know that, I don’t want them to know.” So even though the patient might’ve agreed to the data policy of the AI system, and even though they understand why it’s being recorded, suddenly it’s paranoia again. It’s like, whoa, hold on a minute. I don’t like people knowing, for example, that I’m going to the toilet four times a night.” ~ George Kowalski, VP of Business Development at MiiCare
Thus, the acquisition of holistic patient data is a significant, though inevitable, challenge for AI in healthcare. But this is not all. Even if you manage to acquire access to healthcare data, this does not guarantee that it will be in a clean and model-ready format.
As Hans Kaspersetz from Relevate Health Group commented in a MM+M article, the complex, multi-faceted, and potentially siloed nature of the data required to accurately predict risk for health conditions can significantly hinder the efficiency of AI systems such as IBM’s Watson Oncology Advisor.
“IBM didn’t quite understand the complexity of health records, especially the fact that the majority of them are on paper, and that they’re all recorded differently. They had to apply an enormous amount of human power to apply hygiene to the data, then to label it and get it into the database so that the machine learning algorithms could start to make sense out of it.” ~ Hans Kaspersetz, Chief Innovation Officer at Relevate Health Group
This highlights the complexity of deploying AI models in the real world, where humans are used to operating and working in sup-optimal conditions with messy data and complex requirements. Indeed, a significant challenge for utilizing AI in healthcare is just the often less glamorous groundwork of preparing existing systems for integration with AI models – this includes both the standardization of clinical workflows for healthcare workers, and the development of transparent and comprehensive data collection pipelines.
Indeed, while increasing digitization of medical systems over the past decade (and particularly during the Covid-19 pandemic) now means that the majority of health records are stored digitally rather than on paper, there is still a long way to go before AI models have access to enough clean and high quality data to truly transform healthcare on both an industry-wide and individual level.
However, according to Cleve, while the complexity of healthcare data is still a significant challenge for AI healthcare projects today, this issue often stems from insufficient collaboration between tech companies/software developers and their healthcare partners.
“Failures often stem from overestimating the maturity of AI models and underestimating the complexity of healthcare data. Additionally, insufficient collaboration with healthcare professionals plays a significant role. These setbacks underscore the necessity of setting realistic expectations, rigorous validation, and deep integration with existing healthcare workflows to ensure the successful deployment of AI in the healthcare market.” ~ Andreas Cleve, CEO and Co-founder of Corti
Collaboration is indeed key to successful implementation of AI more generally. This is particularly the case when expanding the reach of AI’s potential into more countries and industries, as we explore in more detail below.
Potential incompatibility of AI with existing healthcare systems
As one of the most fundamental industries to human society, healthcare spans just about the whole range of cultures, communities, and geographies that form the backbone of human civilisations across the globe. For this reason, it is one of the industries in which AI could fulfil vast potential and transform human experience on a truly international scale.
However, on the flipside of this potential is the complexity that comes from integrating AI systems with established healthcare systems across different cultures and communities, where resources, expectations, and traditions vary greatly.
A clear example demonstrating the potential pitfalls of dismissing this challenge comes from Google Health’s attempt to implement an AI system for Diabetic Retinopathy in Thailand and India (see above). The failure of this project is widely attributed to the lack of high quality resources in these countries, even though ironically this was a big incentive for the development of this AI system in the first place.
Of course, this is probably one of the more extreme examples highlighting the impact of cultural, geographical and historical factors on the successful implementation of AI in local healthcare systems.
Nevertheless, Google Health’s failure to account for the well-documented difference in resources between the healthcare systems of the East and West raises the question of how well AI systems developed in America and the first-world countries of Western Europe can be implemented in third world countries. Even if the difference in resources could be accounted for, there are also significant cultural differences that would need thoughtful consideration.
For example, many Eastern countries have a unique and rich history of medicinal that are still very much in practice today. India, for example, has a rich tradition of Ayurvedic medicine. Meanwhile, traditional Chinese medicine typically involves herbal remedies and acupuncture based on the philosophical principle of balancing the yin and yang, and understanding the body in terms of energy (or chi).
While several these practices are known about and practiced to some extent in the Western world, they are often labelled as ‘alternative medicine’, and typically have a minority status compared to modern Western medicine.
Implementing Western-developed AI healthcare systems in non-Western cultures thus runs a high risk of cultural bias, which could not only aggravate local communities by undermining and clashing with their cultural traditions, but also reduce the diversity of medicinal practices across the globe.
This highlights collaboration between tech companies and local healthcare providers as a crucial way to streamline the integration of AI into local communities, avoid misunderstandings over the purpose/capability of the AI systems, prevent any potential misuse of the system, and help more generally to mitigate the risk of globalisation, which is particular risk for big tech companies which tend to be primarily based in the U.S.
Additionally, as pointed out by Deep Medical Founders and Co-CEOs, Dr Benyamin Deldar & David Hanbury, successful AI healthcare systems require holistic input from not just software developers and medical professionals, but also the patients, families, employees, and businesses/organisations that form the healthcare community of a local area.
In particular, they point out the importance of understanding the sentiment towards AI amongst the people who will be using and interacting with the technology, and being prepared to accept that the best interests of the tech company may not always align with the best interests of the customers they aim to serve, even if the company has the best intentions in the world.
“Healthcare is multifaceted. You know, it’s a really big umbrella term, so I think you need to understand the politics that are at play in healthcare just as much as you need to identify a problem and a solution. This is especially true when it comes to AI because of the distrust and fear around the emerging technology. For example, I used to be in the space of doing radiology scans and building the AI models to help with them, but every week we would talk about whether the technology is going to replace us or not, or whether it was really going to work. So you really saw that kind of reluctance towards the technology playing out. But I think that what this really highlights is that what we might think is best initially for an individual or a patient, may not necessarily be the business that grows in this environment. And so the politics of healthcare is really about considering how to deliver AI solutions.” ~ Dr Benyamin Deldar & David Hanbury, Co-CEOs and Founders of Deep Medical
Furthermore, Deldar and Hanbury argue that factors such as geography, culture, and even private versus public healthcare should form some of the most fundamental and initial considerations in the development of any AI system. This, rather than the potential of the technology itself, should form the crux of the business model for any company developing AI solutions for healthcare.
“Something that is really important is being able to take a bird’s eye view into what people are saying and doing, and then to really ideate about where and how to find the right areas for the application of AI. Because I think that changes as well depending on where you’re choosing to set up your company. So do you want to work in a private healthcare setting, because they’re more orientated around profit and loss? Or do you want to work in a more social care setting where you can be more focused the benefits the patient and the system? Or even, do you want to go somewhere where there are very little provisions of healthcare, in places where you can really, truly challenge some of those status quos in healthcare – because this is far more difficult to do in places like the UK, America and Europe where the standard of care is higher. So I would say, make sure that you respect or understand the sort of differences in how healthcare is delivered, how multifaceted it is, and how that changes across different geographies, and then go into the solution around that.” ~ Dr Benyamin Deldar & David Hanbury, Co-CEOs and Founders of Deep Medical
Furthermore, Kowalski points out that cultural differences between different regions can have a significant impact on the success of an AI healthcare project in different places. For example, many countries are far more wary towards data collection and public surveillance than other countries, and regulation surrounding these issues also varies from place to place.
“So at MiiCare, we signed an agreement with a care home
which said to us, yes, we’d like to use your product. However, privacy concerns
were quite prevalent in the region where the home is located, and there was a
notable reluctance from residents when it came to being assessed and monitored.
When we told the patients about all the great things we could do with
monitoring (particularly remote monitoring) to make sure that they stayed
healthy and well, some of them were still thinking, ‘well, I don’t want it. I
don’t want someone watching me.’ So that’s kind of an unavoidable problem. Even
if your solution is one of the best in the world, you’re always going to get
someone who says, no, not for me thanks.” ~ George Kowalski, VP of Business
Development at MiiCare
This brings us to the crucial issue of data collection and usage, which is arguably the greatest challenge of AI in healthcare. In the next section, we consider the ethical issues surrounding data collection in healthcare, and the importance of fostering trust in AI systems through secure and transparent data management pipelines.
Lack of transparent and secure data collection
It is no surprise that one of the key provisions of the now in-force EU AI Act is that companies must provide greater transparency regarding the data used to train AI models.
With the proliferation of Generative AI models and tools over the last 18 months, two major areas of concern have emerged: data privacy and data security.
These issues have stirred up particular agitation in the creative industries, where existing copyright protection has failed to protect creatives against the use of their work to train AI models.
Healthcare is another industry where the data used to train models has raised significant ethical concerns – and according to Dr Ameera Patel, MD PhD and CEO of Tidalsense, these concerns are not unfounded.
“I am genuinely scared by the risks and security issues with more complex AI models swimming around the internet, and it will be a long time before any of these ultra-high complexity models like LLMs make it into mainstream clinical practice, purely because, as of today, they are unsafe.” ~ Dr Ameera Patel, MD PhD, Chief Executive Officer at Tidalsense
In her view, many of even the big tech companies hoping to manufacture medical devices have got a long way to go in terms of compliance and regulation before they will be able to provide the sufficient level of transparency and security required to collect and use patient data.
“Big tech companies are great at coming up with reasonably high complexity AI solutions that have potential to solve amazing problems. But the level of performance, and cybersecurity scrutiny of medical devices means that some of these algorithms haven’t got a chance of being regulated until we solve some more research problems first.” ~ Dr Ameera Patel, MD PhD, and CEO at Tidalsense
As Patel mentions here, cybersecurity is a key issue which plays into the viability of AI solutions in real-life healthcare applications. Even without AI systems, healthcare systems are already vulnerable targets for cyberattacks. Earlier this year (June 2024), for example, Synnovis, a pathology lab which processes NHS data, was hacked in a ransomware attack orchestrated a by Russian cybercrime group, which led to the exposure of personal and sensitive patient data.
When it comes to implementing AI systems, which typically require vast quantities of sensitive data, this vulnerability only increases.
Furthermore, Kowalski discloses that many organisations planning to implement AI systems in healthcare are still lacking in compliance with the regulatory and standards in healthcare for security.
“It really is imperative that the correct level of security is in the actual system itself. There are so many ideas on the market at this moment in time which don’t have the necessary regulative information security levels, accreditation, or the other specifics required to deal with any data, let alone patients’ medical data. One of the main requirements is GDPR, which obviously is really important even when people are just looking at a website. Essentially, anything that involves entering your data is covered by GDPR, and that’s vitally important. On top of that, you’ve also got specifics like cyber security and other security methodologies that are in place that you should sign up to and have in place prior to working with any organization. And that’s whether it’s the NHS or whether it’s a private organization, whether it’s an insurance company or anybody at all. And I can tell you right now there are a lot of organizations out there who either don’t have the relevant security in place or say they do because they’re working towards it, or basically haven’t even started working towards it yet.” ~ George Kowalski, VP of Business Development at MiiCare
This highlights secure data management as key challenge for AI in healthcare. Yet in this industry, perhaps more than any other, having robust security for patient data will be fundamental to gaining trust from the public, and getting patients on board with piloting AI systems.
“Trust in healthcare AI is crucial as it directly impacts patient outcomes and the adoption of life-saving technologies. Without trust, patients and providers may hesitate to use AI-driven tools, obstructing the path to safer, higher-quality care. Concerns over patient data usage are prevalent, making it essential to ensure robust data privacy and security through encryption, access controls, and de-identification techniques. Compliance with regulations like HIPAA and GDPR, along with ethical guidelines, is vital for building trust. Additionally, standardizing data, maintaining high data quality, and implementing strong governance practices are equally important. As we integrate AI into healthcare, we must ensure it operates with the highest standards of accuracy and ethics—because in healthcare, trust isn’t just a virtue; it’s a necessity.” ~ Andreas Cleve, CEO and Co-founder of Corti
Realistic ambitions for AI in healthcare
Given the challenges we have discussed above for AI in healthcare, you could argue that some of big tech’s ambitions for AI in healthcare verge on the unattainable, at least in the not-so-distant future.
According to Alex John London, director of Carnegie Mellon’s Center for Ethics and Policy, the healthcare industry is still a long way from being fully AI-ready.
Speaking at an AI & Medicine event at the University of Seattle in June, as reviewed in a Geekwire article, he argued that healthcare requires a fundamental, structural revamp in order for AI to reach its full potential in this industry.
“The structural problems [of the healthcare industry] are not going to be changed by doing fancy work on your dataset. To really make use of AI and get all the value out of AI in healthcare, we have to change health systems, the data that we generate, our ability to learn, the way we deliver health care, and who’s included in our systems. Until we do that, it’s going to be incredibly hard to get the value that we want out of artificial intelligence.” ~ Alex John London, Director of Carnegie Mellon’s Center for Ethics and Policy
Nevertheless, healthcare remains one of the most exciting areas for transformation through AI, even if this transformation takes place primarily through the less ‘glamorous’ applications of AI such as administration.
Patel points out that this transformation is being driven not just by the ambitions of big tech companies, but also equally by smaller companies and startups whose agility in the fast-moving tech industry gives them vast potential to innovate at the very cutting-edge of AI and healthcare.
“I think it’s great to be ambitious – we need ambition to move forwards. And I don’t think it’s just big tech companies that are ambitious – there are plenty of small companies (the future big tech companies) driving incredible innovations.” ~ Dr Ameera Patel, MD PhD, Chief Executive Officer at Tidalsense
Additionally, Cleve sees vast potential for AI in the more scientific and research-focused area of biotech, where AI can be used to accelerate scientific discovery in molecular biology and heuristics. Given that these applications are more lab-based and do not involve such direct interaction with public healthcare systems and vulnerable patients, they have the advantage of evading some of the more stringent legislation related to user privacy and the alleviating some of the complexity that arises when integrating AI systems into local communities.
“An exciting opportunity for AI healthcare to leap forward is emerging in molecular biology, where machine learning tools are unlocking and understanding the complexities of DNA. Companies like Isomorphic Labs are prime examples of how AI can accelerate drug discovery, providing immediate applications compared to more futuristic projects like Neuralink.” ~ Andreas Cleve, CEO and Co-founder of Corti