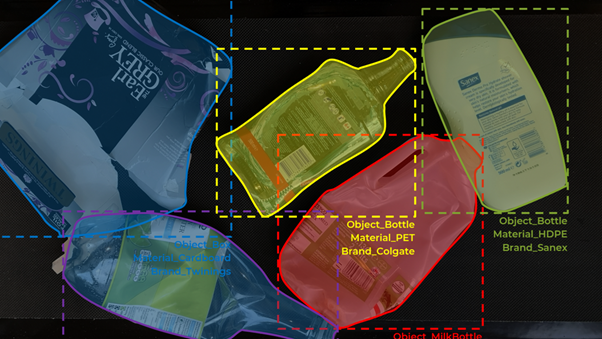
Over the course of human history, technology has proved again and again to be one of the most powerful tools that we have to ensure our survival. From primitive hunting tools to vaccines to the internet, technology has been humanity’s greatest ally. It has enabled us to live for longer, travel the world, and access vast deposits of human history in just seconds. Challenges which once seemed insurmountable, such as curing cancer, exploring Mars, and ending world hunger, are now well within the realms of possibility with the technology we already have or soon will.
In the modern world, one of the greatest challenges we face is the self-imposed threat of climate change. Due to the heavy demands of modern human consumption, environmental diversity is gradually being stifled as the broad array of life forms that we rely on for survival are being over-harvested, poisoned, or losing their habitats.
AI’s impact on the environment is double-edged. On the one hand, the analytical capabilities of AI promise to foster innovative new ways of tracking our impact on the environment and reversing the damage. For example, through a machine learning model, scientists were able to create a new variant of an enzyme, FAST-PETase, that can break down plastics into smaller, recyclable parts, in just hours. This speeds up the natural process of decomposition, which otherwise takes centuries. Predictive AI models are also being used to analyse historical data and provide greater accuracy in forecasting the impact of climate change on ecosystems and biodiversity.
On the other hand, AI is a big consumer of energy, with data centres already accounting for 1-1.5% of global electricity consumption, according to the International Energy Agency. And this rate will double, triple, and quadruple in the coming years, just as the technology itself advances exponentially. OpenAI and Microsoft, for example, are building a $100 billion data centre and supercomputer, which is expected to require an annual supply of 5GW in electrical power. To put this into perspective, this is more than some countries, such as the Netherlands, use in a year!
Ultimately though, there is no reversing AI. Its energy consumption, while undeniably huge, will simply be added to the global demand for energy which is constantly on the rise. Nevertheless, it will put more pressure on net-zero targets that many countries have made, and continue to commit to (and albeit extend their deadlines for) at the annual COP negotiations. For many countries though, this is a sacrifice they are willing to make, given the crucial role that AI already plays in accelerating environmental cleanup efforts.
Overall, the energy costs required to power AI makes its applications in the environmental sector some of the most fundamental to its own sustainability. Fortunately, many innovators in tech are similarly minded, and AI is already being used in a range of creative ways to address environmental issues. One of the biggest of these issues is waste.
The problem of waste
Humans generate over 2 billion tonnes of waste per year – about half of this ends up in the landfill. Despite the implementation of environmentally conscious measures such as compacting the waste, covering it over with soil, and putting in drainage systems to reduce groundwater contamination, landfills nevertheless continue to destroy natural habitats, filling the earth with trash that will take hundreds of years to naturally decompose. Furthermore, landfills are one of the greatest accelerators of global warming due to the large amounts of methane they produce as a biproduct of decomposition.
But even for the minority out there who are not concerned by climate change, the fact remains that we will eventually run out of space for our waste. Based on research conducted in 2015 by Dr Bryan Staley, CEO of the Environmental Research and Education Foundation, RoadRunner Recycling estimates that the states of America have an average of just 62 years until all of their current landfill facilities are filled to the brim.
The finite reality of available landfill space is not a new issue for governments, but remains one for which an ethical and sustainable solution is yet to be found. For many years, several western countries including the UK and USA have been relying on the irresponsible practice of exporting their waste to other countries in exchange for money. But these countries too will eventually run out of space, regardless of how big they are. China, for example, stopped accepting plastic waste from America in 2018, claiming that it no longer wanted to be the “world’s garbage dump”.
In light of this, efforts which utilize AI technology to optimize recycling efforts and promote a circular economy are more important than ever. Below, we look at various environmental use-cases for AI including robotics & computer vision, ML, and predictive algorithms.
Optimizing recycling and waste sorting efforts
Recycling is one of the simplest and most effective ways to reduce the amount of waste that is put into landfills. Industrial recycling is not a perfect environmental waste solution due to the energy required to sort, clean, process, and redistribute the materials. Nevertheless, it remains a preferable option to dumping all waste in landfills, and will play a crucial role in promoting the sustainability of current human living standards by reducing the amount of raw material we need to harvest directly from the earth. Furthermore, as many energy suppliers move increasingly towards using renewable sources of energy, industrial recycling has the potential to become a fully green solution for waste management.
Most of us are now used to making the effort to recycle within our homes because waste sorting is not just promoted but required by most councils and waste collection services. Nevertheless, despite these efforts, the current rate of recycling is much lower than it could be in many countries. In the UK, approximately 45% of waste is recycled even though analysis shows that up to 80% of waste could be recycled. In the US, this rate is even less at just 32% or lower. But this problem goes beyond our individual efforts to recycle. A 2022 report by OECD indicates that the global rate of recycling is actually just 9% despite up to 40% of waste being collected as recycling.
Optimizing the waste sorting process at recycling plants therefore plays a key role in improving actual recycling rates, and ensuring that the recycling efforts people make at home pay off. Waste solutions providers such as Recycleye, Greyparrot, and AMP Robotics are helping do this by installing AI-powered equipment in MRFs (materials recovery facilities) to increase waste intelligence and enhance the accuracy of material detection.
Recycleye offers a comprehensive solution, combining AI analytics with robotics. Its scalable waste-sorting robots, which are fitted with computer vision software, are placed over the conveyer belts which carry streams of already sorted materials. The robots then detect and pick out any impurities/recyclables from these streams. Zoe Cook, Technical Sales Manager for the UK at Recycleye, explains in more detail how the process works below.
“A camera is attached over the top of the conveyor belt, identifying different visual features of each item like the human eye would, e.g. lid shape, transparency, colour etc. The machine learning algorithms, which have been trained on a dataset of 1 billion waste images, then rate how confident they are that they have seen each feature and combine the results to determine the item’s categorisation. This means the computer vision can categorise items based on material and object (e.g. aluminium aerosol) and be trained to recognise infinite new waste classes to unprecedented detail and granularity (e.g. sort transparent PET water bottles from very light blue PET water bottles).”
Zoe Cook, Technical Sales Manager for the UK at Recycleye
So far, Recycleye’s solution has produced promising results for increasing recycling rates, demonstrating the transformative power of using integrated AI technology to counter a widely experienced problem within the waste management sector. At a MRF in Reading, UK, for example, Recycleye managed to increase the recycling rate of the targeted material by 12%. But this is not all. Recycleye’s use of AI technology also increases the cost efficiency of MRFs and provides more accurate data about incoming and outgoing waste materials, which can then be used to inform future waste management strategies and evaluate the efficiency of current solutions.
“Using robotics automates the manual job of quality control in MRFs, which lowers the cost of waste sorting and increases the consistency of picking over a shift, with our robots reporting 98% availability. Usually one robot can replace one manual picker. As many facilities operate on multiple shifts, the robot operates 24/7, which may have previously required 3 FTEs. AI-powered robots also allow sorting into more granular categories that was possible with existing machinery and manual picking, such as food grade v non food grade plastic sorting; multi-materials like beverage cartons; black plastic. Hence, new, purer waste streams are being forged, which increases the resale value of bales for a MRF. Computer vision also allows the plant manager to scan and gather data on 100% of their material, rather than conducting random manual sampling as was the case previously.”
Zoe Cook, Technical Sales Manager for the UK at Recycleye
In light of this automated solution for material waste sorting, we might be wondering how necessary it is to keep recycling at home. But for the near future at least, it remains very important to recycle at home. This is because Recycleye’s robots aim to purify already filtered waste streams, rather than sort mixed/general waste from scratch
“The robots are used to automate the quality control at the back-end of the plant, taking already very pure material streams and making them purer, or recovering missed recyclables from the residual line heading to landfill. Since the technology is a ‘last check’ for mistakes, it is still very important that people sort their waste accurately at home.”
Zoe Cook, Technical Sales Manager for the UK at Recycleye
The use of AI technology to optimize the waste sorting process at MRFs demonstrates the potential of AI to automate many of the mundane and repetitive processes that are crucial to global sustainability efforts. The meticulous, accurate, and tireless nature of AI-powered technology makes it an optimal candidate for tasks which require constant concentration and attention to detail, but which are not in themselves particularly complex. Waste sorting is one such task, and its AI-enabled automation sets a good example of how AI can address real world problems and enhance our efforts to create a more circular economy.
Furthermore, the versatility of AI technology means that it can carry out similar functions in locations where it is more difficult for humans to work, such as the oceans and rivers which are facing severe environmental consequences due to irresponsible waste disposal. Ocean Vision AI, for example, has created a machine learning platform specialized in the recognition and processing of underwater objects, helping to identify how much waste there currently is in the oceans, whereabouts it is, and its impact on sea life.
Enhancing the accuracy of supply and demand chains
Another key way that AI can be used to address the problem of waste is by reducing the amount of stuff we throw away to begin with. Indeed, while the consumerism of the Western world means that there is now even a surplus of resources to go around (at least in theory), this has had an unintended consequence of creating huge amounts of waste, both at a domestic and an industrial level. Primarily, this is a problem within the food sector, given the limited shelf life of fresh food. Nevertheless, other industries such as electronics and fashion are also large producers of waste given their rapidly changing trends.
Primarily, this is a preventable issue that has already been addressed to some extent at a societal level by raising environmental awareness and creating eco-friendly trends. For example, sustainability initiatives such as Too Good To Go and H&M’s garment collecting programme aim to redistribute redundant resources at minimal cost to the environment, and tackle the throwaway cultures of the hospitality and fast fashion industries.
But implementing these initiatives on a broad scale is challenging given the complexity of redistribution, which requires companies to have a holistic and thorough understanding of their supply and demand chains, and be able to navigate large networks of interdependent vendors and businesses. For this reason, several companies promoting these types of sustainable initiatives are utilizing AI so they can more easily predict where resource surplus will end up, and gain real-time insight into fluctuating resource demands to better inform their redistribution strategy.
Too Good To Go, for instance, provides an AI-enabled software solution for food suppliers that enables them to easily track and redistribute surplus food. In the energy sector, software company Green.ai is utilizing predictive algorithms and forecasting software to more efficiently manage the complex supply and demand channels for electrical power.
Similarly, electronics supplier Lenovo has recently launched a new initiative, Certified Refurbished, to promote sustainability, cost-efficiency, and circular practice within the tech industry. Combining this initiative with its AI-solutions platform, Lenovo TruScale, the company will provide its customers with new options to customize their devices and IT mix according to their unique business needs through its DaaS (device as a service) package. This aims to optimize and elongate the lifespan of devices by utilizing AI to analyse and forecast business-specific IT requirements. Claudia Contreras, Executive Director of Global Sustainability Services for Lenovo, explains more about the initiative in a press release sent to the AI Journal.
“Environmental sustainability is a leading concern for businesses of every size, in every sector. Lenovo Certified Refurbished extends the lifecycle of our technology to improve ROI and help reduce waste, offering a solution that provides customers a purchase option to help support a more sustainable future. Certified Refurbished will help reduce e-waste, give devices new life, and positively contribute to the circular economy.”
Claudia Contreras, Executive Director of Global Sustainability Services for Lenovo
Jim Chappell, Global Head of AI at AVEVA, a leading industrial software provider, sees a key role for predictive analytics in industrial contexts. Industrial centres such as factories can waste huge amounts of natural resources through equipment failures, operational inefficiency, and inevitable maintenance checks and safety procedures.
“In the journey toward a sustainable future, resources must not be wasted, and surpluses must be kept to a minimum. Predictive analytics allows companies to optimize the allocation of resources and perform maintenance only when needed so that assets and operations are not over or under maintained. Factory shutdowns for maintenance use a great deal of excess resources, including significant additional power and natural resource consumption. Through predictive analytics, the timing of maintenance can be optimized in order to minimize unnecessary shutdowns, reducing the amount of maintenance material required while also minimizing waste and scrap loss. Underperforming assets can be identified very early before they become a major impact on efficiency loss. By integrating predictive analytics, root cause can be determined so that operations can quickly be brought back to full efficiency, minimizing wasted resources.”
Jim Chappell, Global Head of AI at AVEVA
Jim illustrates the tangible benefits of utilizing predictive analytics in industrial contexts, giving the example of a US power producer that managed to avoid wasting 100,000 gallons of water and producing 2000 metric tons of CO2 per week by using predictive analytics. The technology was able identify a simple operational error in the reconfiguration of one of its generating units that had been taken offline due to a leakage. “Since the issue would not likely have been detected for several weeks, a huge resource impact was avoided through AI”, he explains.
Overall, with speedy and accurate abilities to process and analyse huge amounts of data, AI-powered analytics present a great opportunity for businesses of all kinds to become more efficient and eco-friendly, optimizing their supply and demand chains and maintenance operations to reduce waste whilst ensuring that the required resources get to the right places at the right time.
Removing waste from space
One last key application for AI in waste management that we look at in this article is how it can be used to help combat the growing problem of space debris. The human tendency to leave their waste for someone (or something) else to deal with is unfortunately not just an earthly habit.
Space exploration using satellites and drones has left more than 55,000 pieces of defunct equipment orbiting the earth. And this number represents only the pieces of debris we have been able to track. Due to the Kessler syndrome, in which collisions split equipment into an exponentially growing stream of more pieces, the Federation of American Scientists estimates the actual number to be more like 170 million. And this is not just a mildly claustrophobic thought, it is a growing problem that is disrupting space exploration, weather forecasting, global communications, and even national security.
The International Space Station, for example, has had to perform manual manoeuvres just to avoid its equipment colliding with debris. Indeed, the problem of space debris has now reached a level of severity that requires us to take more action than just making sure that we don’t add to the existing streams of debris. As Congressman John Hickenlooper says, “because of the threats from debris already in orbit, simply preventing more debris in the future is not enough.”
This problem has led several authorities including NASA and the UK government to send out aircraft into space with the specific mission of capturing waste and bringing it back to earth to be recycled. Existing solutions that are already being used or are being proposed include: robotic capture, laser ablation, and harpoons & tethers.
- Robotic capture involves using robotic arms to capture and de-orbit debris.
- Laser ablation works by using lasers (based either on Earth or in space) to alter the course of debris and push it towards atmospheric entry where it then burns up.
- Harpoons & tethers, similar to robotic capture, these aim to snag the debris, then slow it down and ultimately de-orbit the debris using drag tethers.
Due to the remote nature of the mission, all of these solutions will utilize automation, and the power of predictive analytics to ensure their safety and success. Sylvester Kaczmarek, CTO at OrbiSky systems and expert in the integration of AI and robotics in aerospace applications, highlights the key benefits that AI brings to these missions.
Enhanced Orbit Determination and Prediction
Machine learning algorithms are able to increase the accuracy of tracking and predicting the orbits of space debris. In particular, Deep Learning paradigms, which use neural representations to process more complex data that contains multiple layers of information within a network, are being used to process large, multi-modal datasets that come from a variety of sensors including radar, optical, and infrared telescopes. This allows for the algorithms to account for more complex variables such as solar radiation pressure and atmospheric that can exert significant force on the debris orbiting Earth.
“Enhanced predictive capabilities allow for comprehensive risk assessments, spotting potential collision events well before they occur. Such foresight enables operators to take proactive steps, such as maneuvering spacecraft out of harm’s way or deploying additional protective measures. Improving the accuracy of these predictions is vital for scheduling debris removal missions more effectively, ensuring higher success rates and minimizing the risks of unintended collisions with active satellites.”
Sylvester Kaczmarek, CTO at OrbiSky Systems
Autonomous decision-making for Robotic Removal Systems
Existing Robotic Removal Systems face the challenge of dealing with unexpected developments encountered in space during the missions themselves, such as a collision or unexpected placement of the targeted debris. Traditionally, these situations are handled by humans who can manoeuvre spacecraft remotely from space stations. But this method can be hindered by communication delays and the limitations of ex-situ intelligence. Integrating autonomous AI systems that can adjust to new information and emerging events as they unfold gives robotic removal systems an unprecedented level of versatility, enabling them to function more effectively, safely, and quickly.
“AI systems can dynamically adjust their strategies during a mission, relying on sensor feedback and pre-established safety protocols to optimize debris capture and handling, thereby reducing the need for direct human control and decreasing response times.”
Sylvester Kaczmarek, CTO at OrbiSky Systems
Mission Planning and Optimization
Similar to how ML algorithms are being used to track and predict the movements of space debris, its analytical capabilities are also extremely useful for planning space missions. For example, it can analyse variables such as potential debris paths, fuel requirements, and mission logistics to reduce the time it takes to organize debris removal missions. It also streamlines the complexity of juggling all these variables at once, where it is easy for humans to make errors that can have unintended but disastrous consequences.
“Using simulations and predictive modelling, AI algorithms can suggest the most effective routes for debris removal crafts, optimizing the use of resources and increasing the number of debris cleared per mission. This comprehensive planning tool helps streamline operations and enhances the overall efficacy of debris removal efforts.”
Sylvester Kaczmarek, CTO at OrbiSky Systems
Overall, AI plays a key role in enhancing the safety and efficiency of space debris removal missions, ensuring that they are able to collect as much debris as possible and can target objects deemed to be a greater risk to active space missions. Furthermore, the vast analytical and predictive capabilities of AI provide us with accurate real-time information about the speed, composition, and proximity of space debris, enabling us to better understand the current and future risks they pose to our planet, and formulate more targeted strategies to counter these risks.
What’s next for AI in waste management?
As AI technology continues to evolve, it will bring increased utility to the applications outlined above, and no doubt find new applications within the waste management industry.
Firstly, within the recycling and waste sorting sector, AI-powered robotics have scope to be used on at a smaller, site-specific level, enabling circular micro-economies that reduce the energy needed to transport and sort the waste at an external facility.
“There is definitely scope for ‘mini-MRF’ type solutions to be installed at airports or shopping centres in the future, so that they can manage their waste on site rather than using energy to transport waste to sorting facilities and then on to be recycled”
Zoe Cook, Technical Sales Manager for the UK at Recycleye
Furthermore, in an interview with Sarah Crespi on Science Podcast, PhD student Zheng Xuan Hoy from Xiamen University proposes that AI can mitigate the detrimental impact of waste on the environment in 4 key ways.
- Retrofitting landfills with biogas capture systems
- Diverting organic waste for composting
- Diverting organic waste for anaerobic digestion
- Reducing waste generation by 50% (relative to 2020 levels)
This proposal reflects the potential of AI to optimize existing waste management practices, accelerate the development of environmentally-friendly practices that will help us achieve net-zero targets, and ultimately, ensure the sustainability of our own existence.
Secondly, the role of predictive analytics will find increasing applications at a larger scale as net zero targets loom ever closer. By providing comprehensive and accurate analyses of current practices, AI will be able to help both individual businesses, and at a larger scale, governments and international alliances, prepare better for future/hypothetical scenarios. Indeed, as the Earth’s climate becomes more volatile and unpredictable, implementing safeguarding practices informed by accurate, data-led predictions will become increasingly important and fundamental to ensuring our survival on a fragile and changing planet.
“Predictive analytics continues to evolve and become more sophisticated. By combining prescriptive and prognostics with predictive, resources can be optimally allocated, and waste minimized, resulting in significant savings. Beyond that, predictive is now being combined with physics-based simulation software to take maintenance to the next level by addressing risks, assessing what-if scenarios, and offering guidance on how to optimize both operations and maintenance, resulting in even more efficient use of resources while minimizing waste.”
Jim Chappell, Global Head of AI at AVEVA
Thirdly, the increasingly capable autonomous abilities of AI will enhance the viability of space debris removal efforts. One of the key challenges for these missions is the harsh environmental conditions which require extremely durable hardware alongside advanced AI software which has advanced fault-tolerance, self-repair capacity, and vast data processing abilities. Systems with this level of resilience are still under development, but hold exciting promise for what we can achieve in space in the future.
“Future advancements may see AI-powered robots performing on-orbit servicing tasks, such as inspections, repairs, and refuelling of active satellites. These operations could significantly extend the operational lifespans of satellites, reducing the need for replacements and, consequently, the generation of additional space debris.”
Sylvester Kaczmarek, CTO at OrbiSky Systems