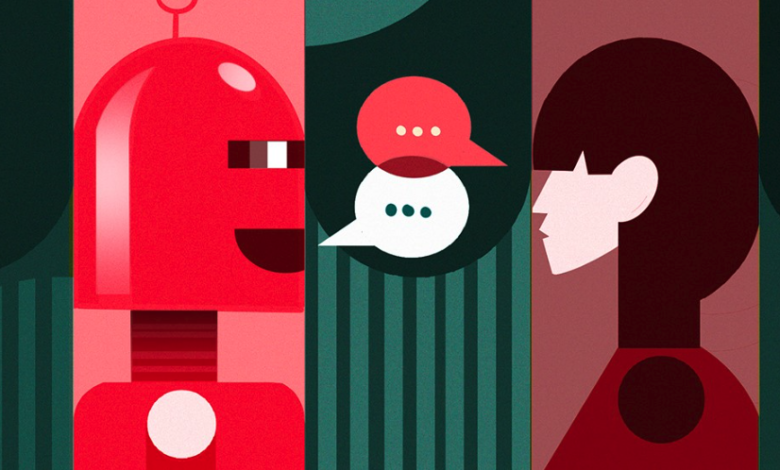
AI has entered every facet of our lives and is changing how people use technologies, from the internet and virtual assistants to self-driving cars. Generative models, lower entry barriers, and quantum data have offered countless capabilities in the digital landscape, opening up new avenues. However, these limitless advancements are cut both ways, enabling cyber fraudsters to deceive and exploit people and organisations.
The depths of AI-driven fraud
Cyber fraudsters craft AI-powered scams, scale them vastly through automation, and capitalise on them. Malicious actors use AI algorithms to deceive others by creating synthetic fake identities and conducting social engineering attacks through chatbots, voice cloning and deepfakes to extract confidential data from people without their knowledge or consent.
Fraud as a Service (FaaS) is an unsettling trend where cybercriminals provide different fraudulent services or tools using websites on the internet or dark web, mirroring the SaaS model and providing access to malicious actors. And, AI-powered FaaS offers a broad spectrum of tools, like phishing and malware kits, synthetic identity theft packages, and botnets.
AI has made automated spear phishing and whaling attacks increasingly common, allowing imposters to access sensitive information. According to the Cyber Security Breaches Survey 2023, “across all UK businesses, there were approximately 2.39 million instances of cybercrime and approximately 49,000 instances of fraud as a result of cybercrime in the last 12 months.”
Phishing occurs not just with emails but also through websites. Every day, people visit harmful sites that retrieve their data and worryingly, the domains of these phishing sites change often, so it is hard to keep a manual blocklist of them. In addition to common phishing attacks, there is also the risk of zero-day attacks where hackers exploit newly discovered vulnerabilities.
The growing challenge is that large language models (LLMs) and machine learning (ML) algorithms learn extensively from public databases and breached datasets from past cyber incidents. AI uses this scraped data in personalised phishing attacks, exploiting users’ trust and familiarity with certain brands by creating phishing sites that resemble the legitimate websites of those brands.
AI-assisted content generation has ushered in both promise and peril. The language models can provide startlingly plausible content for fake product reviews using stealthy tactics mimicking genuine users. These counterfeit reviews entice or dissuade, impacting decision-making and undermining the authenticity and credibility of the product.
The digital revolution has also brought a new wave of serious financial crimes that are more sophisticated than ever before.
Innovations in combating sophisticated fraud
In the face of growing risks, AI fraud detection cannot be overlooked, indeed, AI to combat AI is a growing reality.
Anomaly detection
Datasets have patterns, and any sudden changes that occur in these patterns and data points require supervision. Through anomaly detection, such unusual behaviour can be identified and flagged. Anomaly detection is used in several industries, from healthcare to finance.
For example, in healthcare, it is used to find anomalous readings in a patient’s report and unusual health conditions. Here, AI not only detects anomalies but also offers explanations of why they are anomalies. In finance, banks use anomaly detection to find suspicious transactions and curb fraud. Electricity providers implement it to monitor the consumption of electricity, detect irregularities, and prevent outages.
Natural language processing
Natural language processing (NLP) is used to generate content and understand the user behaviour of target audiences. In the field of banking and insurance, NLP has proven to be quite useful in detecting fraudulent insurance claims.
According to the Association of British Insurers, “in 2021, insurers detected 72,600 dishonest insurance claims valued at £1.1 billion. It is estimated that a similar amount of fraud goes undetected each year. This is why insurers invest at least £200 million each year to identify fraud.”
Banks operate in various regions and are required to abide by the rules about those regions. This can make the detection of fraudulent activities using just documents challenging. Banks have information about their customers through the Know Your Customer framework and its customer due diligence requirements. NLP can use predictive models and text mining on this data to identify a customer’s fraud risk score in real-time.
Identifying deep fake videos
Deepfakes are becoming too realistic, making it difficult to identify fake images, audio, and video. To mitigate this, Intel introduced a deepfake detector called FakeCatcher, which can detect fake videos with a 96% accuracy rate.
FakeCatcher identifies the “‘blood flow’ in the pixels of a video. When our hearts pump blood, our veins change colour. These blood flow signals are collected from all over the face, and algorithms translate these signals into spatiotemporal maps. Then, using deep learning, we can instantly detect whether a video is real or fake.”
Keystroke dynamics
Cyberattacks are prevalent across industries. According to Statista, the global cost of cybercrime is estimated to increase by USD 5.7 trillion (+69.94%) by 2028.
Two-factor authentication and CAPTCHA are some of the most common ways to verify the authenticity of a user, and now AI is integrated into them via biometric verification. In keystroke dynamics, a unique biometric template of an individual is obtained by analysing the pace at which they type. This data is collected and integrated with a neural network, which “achieves an accuracy of 99% and offers a promising hybrid security layer against password vulnerability.”
To wrap it up
In the past, fraud prevention was based on following traditional rules, but AI learns from past data to predict future trends, helping businesses improve the accuracy of their fraud detection. AI can also discover and mitigate risks in real time rather than in hours or weeks. AI used to be considered a black box, given how most of its processes were difficult to understand, but now it offers insights and explanations of its decisions, thereby improving accountability.
On the flip side, as the ways to mitigate fraud are growing, so are the ways to commit fraud. Training an AI model with high-quality data and enabling it to identify patterns in fraudulent activities can help prevent fraud. Businesses also need to have multilayered security approaches, remain vigilant, and make employees aware of new types of attacks.