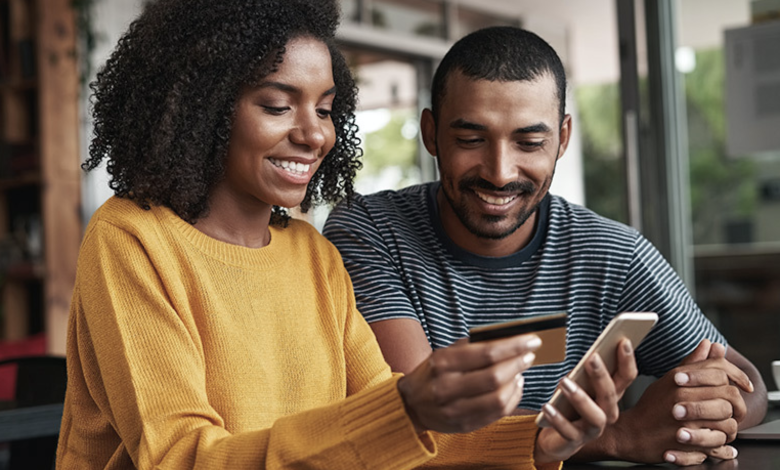
This article was co-authored by Riaz Raihan, President of Products and Manuel Zahariev, Vice President of Engineering (AI & Analytics) at Alida.
Artificial Intelligence (AI) has a rich history of optimizing business outcomes. Over the past decade, airlines worldwide have used AI to set dynamic pricing for airline tickets. We have also seen the introduction of user-driven AI recommendation engines to improve the customer experience (CX). For example, in 2009, Netflix awarded a $1 million prize in a competition for the best algorithm to improve the accuracy of predictions about how much a user would enjoy a movie based on their preferences.
As seen in the scenarios above, AI was first available to consumer-facing behemoths who could afford to fund fundamental research in Machine Learning (ML). AI today is being broadened to cover more rich, flexible and fluid use cases. It is also being democratized by extending its reach to smaller, more dynamic organizations. All brands can now use AI-driven systems and solutions to empower the customer experience and provide the best value for their customers. Leveraging AI in your Customer Experience Management (CXM) system may feel complex, but using predictive analytics has now become essential to drive customer loyalty and increase revenue.
Here are three steps to use predictive models to build a greater customer experience:
1. Gather Data from Multiple Sources
AI traditionally has been used for modelling transactional data, such as predicting what the customer will buy next or how much they are willing to pay for an airline ticket. Today, with customer needs constantly evolving, transactional data is no longer enough. To build the foundation for a great CX program, brands first need to gather data from various sources and listen to their customers on a regular basis.
AI works by learning models of individual or group behaviour from transactional data (history of business interactions with the brand), behavioural data (other interactions with the brand or other brands), research data (profiles, opinions, expressed or implied desires), and even from the online or social presence of consumers (social postings, reviews, customer service messages). Combining data from all these sources will increase the accuracy of the predictive models.
As a rule of thumb, the richer customer insights you have, the better the potential of the predictive model. Here is how AI can be used with different data to predict outcomes and recommend actions:
- Quantitative data can be used to drive pricing and packaging of products. For instance, you know how much of your product Ann has purchased over the course of the year, and how much money she has spent in different categories. Using predictive analytics, you are able to predict what Ann is going to buy next year and are able to package better offers for her. If you know that Ann bought a coat in November 2020, you may be able to suggest a fall wardrobe package for her in 2021.
- Time series data can be used to optimize the timing of interactions with the customer. For example, data shows you that Jane does her Christmas shopping in November. So the best time to present her with offers would be late October. In another instance, you are aware that Mark complains about price increases. So if you have a price increase for a recurring service at a certain point, you know that’s exactly the time to reach out to Mark with an offer or a client service.
- Categorical data (customer profiles and preferences) can be used to create broad-to-micro-customer segmentation for brand communication. AI enables you to micro-target customers based on their profiles (such as age, gender, income) and the preferences they have expressed. For instance, using AI predictive models, you know that John prefers scuba diving on vacations rather than surfing. So you can now target John specifically with new scuba gear before the next vacation season.
When you combine quantitative, time sensitive and categorical data and use predictive models to understand your customers, the resulting model will be more powerful than the sum of its parts.
2. Apply AI Across Customer Journey Maps
At the core of the next-generation CXM systems, modern AI moulds to much more complex workflows than its venerable predecessors. Applying AI across customer journey maps will enable you to predict and orchestrate the next best experience for your customers. For example, you are aware that Mary has been going over her mobile phone data usage limit for the past couple of months. Your customer service center may be able to offer Mary an increase in her data monthly allowance before she even makes a support call to complain about her bill.
Predicting failure points in a customer’s experience can trigger marketing or business process automation that may guide the customer to a happier path of their experience. For instance, you are able to predict that Tim is looking for a pair of boots. But you also know that your stock is depleted due to unexpected demand. You can preempt Tim’s negative experience by guiding him to an alternative offer even before he begins his search on your site.
Machine learning models don’t need to be static. They can continuously improve through additional touch points along the customer journey, such as A/B tests or short surveys integrated into the customer experience. To keep up with evolving customer needs, using CXM solutions will provide the tools required to allow these dynamic models to keep learning and predict better outcomes.
AI can also be used to enhance combinations of transactional aggregate metrics, such as customer acquisition cost and revenue. It can also include softer metrics, yet strong predictors for future outcomes, such as satisfaction, brand sentiment or engagement. Optimizing these will lead to positive outcomes, such as increasing Customer Lifetime Value (CLV) and reducing churn.
3. Trigger Actions to Improve Customer Experience
Once you’ve gathered data and given it a context with customer journeys, AI enables your CXM system to take actions that impact outcomes. For example, let’s take identifying parts of the journey that contribute to churn. An AI-powered CXM system can not only predict customers who may be at risk of churn, but can also deliver insights into the best method to retain them as customers and can micro-target that at an individual level with personalized action items. It could be a combination of getting the right people or systems in the company connected with them, hearing their needs and finding a solution to meet their needs.
In today’s era, CX leaders should move past creating the best solutions for customers based on what we think they need. Instead, focus on providing them with the best value and experience. For a business to thrive today, it is fundamental for it to drive value and customer health by predicting what customers will do next across their journeys. Using an AI-powered CXM system to first listen to your customers to understand their needs and then act on those needs, will improve value realization, both for customers and for your company.