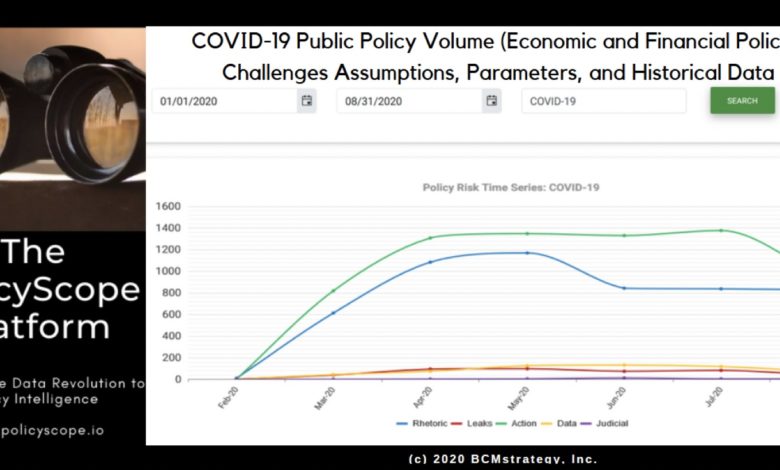
Machine learning and artificial intelligence enhance considerably the ability to assess correlations and covariances across a broader range of data sets to identify more precisely the probability of default for any given set of exposures. Because machine learning does not require rule-based programming to draw conclusions concerning relationships across data sets, the process provides the potential for better insights into embedded risk relationships which enables bankers to make smarter decisions about potential default rates.
But offloading default probability calculations to AI systems is not a shortcut. Because the automated analysis operates thousands if not millions of iterations in a cycle and because many of the analytical processes occur in a hidden layer, successfully deploying AI into default probability assessments requires great care.
Enthusiasts eager to evangelize often gloss over the most important step in any machine learning project: carefully choosing the data sets used to train the AI systems.
Credit Risk 101
At its core, the lending business is all about extending credit with the minimum risk of loss to the lender. Bankers have devised multiple ways to constrain credit risk over the centuries.
Accepting collateral, varying the time horizon for exposures, and varying the permitted amount of interest rate fluctuation (fixed versus floating) have endured even as the process for identifying the probability of default at the portfolio level has become a numbers game. Historical loss rates for specific instruments and portfolios are now paired with a growing amount of alternative data culled from various digital sources.
It is a complicated process to get right under the best of circumstances. The pandemic requires risk managers to up their game urgently regarding default probability assessments. As discussed below, the pandemic particularly requires risk managers to incorporate more public policy considerations and alternative data points concerning public policy.
Credit Data 101
Many in the machine learning and artificial intelligence field chase large data sets to train their models. They believe that bigger is better. The more data the model has on which to train, the better chance of spotting hidden correlations faster.
Sadly, bigger is not better. In credit particularly, curation is a key component of the risk estimation process. Even if the system identifies correlated risk characteristics occurring naturally within the data sets, the correlation may not signal a causation link which is crucial to understanding the shape of default probability.
The harder questions, which even today usually only humans can answer, are whether the data has been labeled properly and whether it has been accurately transitioned into the format to be used for training AI systems. For example: if a data set does not systematically and correctly identify which borrowers in the past defaulted, the automated analysis conducted within the AI system will start on a faulty foundation. Incorrect estimates are inevitable.
For example, one would not choose to use aircraft leasing data sets to estimate default probabilities on retail credit card portfolios. Nor would one choose to use automobile route data to estimate interest rate swap counterparty default rates. Default rates and behavior might both change during a financial crisis, for example, but the similarity in risk profiles across these data sets would shed no light on the probability that a portfolio would experience abnormally high default rates in the absence of a crisis.
Finally, the underlying components of each data set require careful examination before feeding it as training data to an AI-based system. Changes in regulatory standards can impact loan performance patterns. Examples include:
- Changes in the definition of default (60 days past due? 90 days past due? Other?)
- Changes in accounting standards for loss recognition
- Changes in collateral collection/foreclosure standards
If the training data do not line up with current regulatory standards, then AI-powered processes will generate incorrect correlation and risk exposure estimates because they have been using outdated asset performance patterns.
Which brings us to 2020 and the Pandemic Problem. The pandemic amplifies further the potential for misleading analysis regarding default probabilities. Both humans and automated systems are at risk for misestimating default probabilities not just now but also in the future.
The Pandemic Problem – Credit Risk Data
Consider our crazy 2020 COVID-19 spring. Central banks, financial regulators, and finance ministries spent 8-10 weeks in a frantic scramble to keep the economy and financial system functioning amid the first (and hopefully only) simultaneously shuttering of all G7 economies. It looked like this at the monthly level:
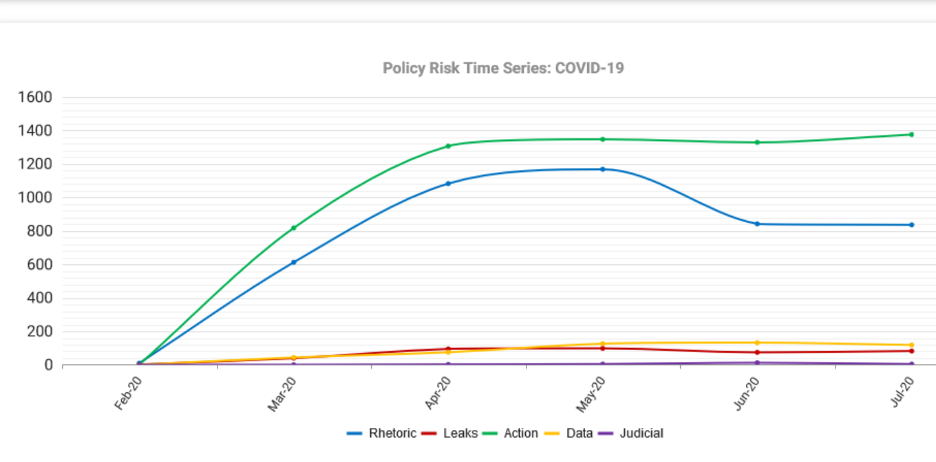
The aggregate year-to-date time series shows a rapid, steep effort to address the crisis….and a sustained high level of activity for every month since the spring. In fact, the data show a slight increase in activity in July. The uptick is consistent with policymakers pivoting to start addressing proactively the nascent indicators of a potential second wave of infections as mobility restrictions were lifted:
Policymakers literally threw the kitchen sink at the pandemic.
- They created massive government guarantee programs that substituted G7 sovereign credit risk for private credit risk.
- They committed central bank programs to purchase a broad range of assets in the open market (government bonds, corporate bonds, corporate equities.
- Moratoria were issued, creating debt service holidays for certain types of assets in some countries.
- Financial regulators publicly encouraged banks to be lenient and flexible when determining if a loan was performing.
- Regulatory capital and loss recognition standards were relaxed.
- Fiscal authorities delivered salary subsidies and additional cash payments to individuals.
- Regulatory reporting and audited account deadlines were relaxed, extended, or suspended temporarily.
By stepping in to prevent systemic collapse, policymakers have also effectively invalidated historical data regarding default probabilities for private sector assets.
Efforts to estimate default probabilities using AI-powered scenario analysis, as well as more traditional extrapolation methods, cannot generate meaningful risk measurements without addressing this break in the time series. In addition, assessing exposure to the probability of default requires far more explicit assessments of exposure to public policy risks.
Default probability parameterization must now also expressly incorporate both sovereign risk and regulatory rule changes implemented in the spring. The policy shifts implemented in the spring of 2020 were designed to be temporary. The policies that expired in June have already been renewed to year-end. Policies expiring in September will also likely be renewed for the most part to year-end.
The credit data issue caused by the pandemic includes a more subtle challenge for those seeking training data for their AI systems: the pandemic creates data deficits just when financial firms require more data to estimate credit risk. Relaxed or suspended regulatory reporting requirements deliver fewer data to governments for aggregation, which in turn delivers less complete data sets to markets. This is a short-run situation, but if machine learning methods are being used without adjustment to shifts in data quality for routine components, model error risks increase silently.
The question then becomes whether — or how — pandemic era policies will be extended through 2021 and beyond as the economy adapts not only to the pandemic but also (hopefully) its end. For the next 18 months, public policy will play an abnormally large role in insulating whether, how, and which borrowers default….and which do not.
Conclusion
AI-powered credit risk systems can accelerate risk estimation processes considerably. Alternative data sets can amplify the accuracy of those systems. But for AI-powered credit risk estimation to generate reliable predictive analytics, it must be trained on data that best approximates the current credit situation.
Merely throwing a random amount of large data into an AI system is no better than throwing spaghetti at a wall. Considerable human judgment and engagement devoted to data curation will increase substantially the accuracy and reliability of automated default risk estimation processes. Given the unusually high levels of sovereign engagement and support for the credit process at present, this means credit risk models must also incorporate data reflecting public policy risks.