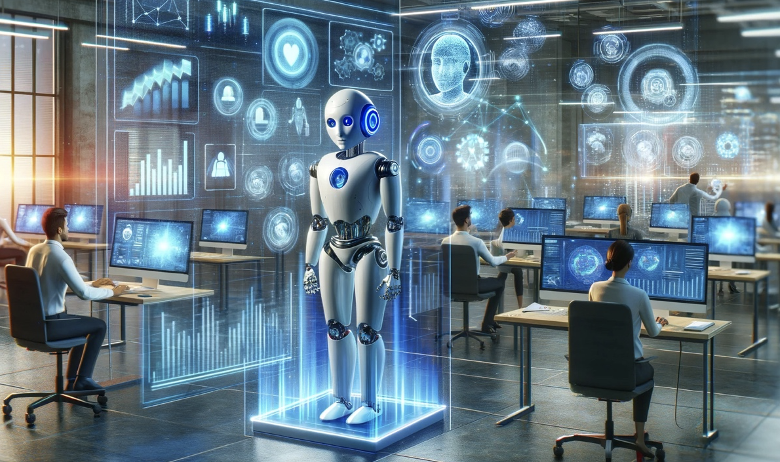
In a recent study, researchers from the University of Chicago have demonstrated that OpenAI’s GPT-4 Turbo, an advanced large language model (LLM), surpasses human analysts and specialized machine learning models in predicting earnings changes from anonymized financial statements. The study’s results and PwC’s recently announced partnership and integration of OpenAI’s technology underscore AI’s significant impact on financial analysis and the broader industry.
The study, titled “Financial Statement Analysis with Large Language Models,” aimed to investigate the potential of LLMs in performing complex financial analysis tasks traditionally carried out by human analysts. The researchers provided GPT-4 Turbo with standardized and anonymous financial statements and instructed it to predict the direction of future earnings.
To ensure the integrity of the results, the researchers employed a “Chain-of-Thought” (CoT) prompting technique, mimicking the step-by-step reasoning of financial analysts. This approach enabled the model to generate more accurate and contextually relevant outputs, achieving higher accuracy and F1 scores than human analysts, especially when analyzing small or loss-making companies.
The study’s findings have significant economic implications. Trading strategies based on GPT-4’s predictions yielded higher Sharpe ratios and alphas compared to other models, indicating improved investment performance and risk-adjusted returns.
The LLM also demonstrated the ability to generate useful narrative insights about a company’s future performance, providing investors with a more comprehensive understanding of a company’s prospects.
Implications for the Financial Industry
The adoption of AI-driven financial analysis has far-reaching implications for stakeholders across the industry – from investors and analysts to companies and markets as a whole.
For investors, LLMs have the potential to provide more accurate and timely insights, enabling better-informed decisions. This capability has already shown promise in enhancing investment strategies and helping managers increase risk-adjusted returns.
The study highlights a significant shift in the role of financial analysts. As AI models like GPT-4 Turbo handle routine analysis tasks, analysts who want to stay relevant must develop new skills in data science and AI literacy to collaborate effectively with these tools. Rather than replacing human judgment, AI augments decision-making by providing comprehensive and timely information. This symbiotic relationship between AI and human expertise allows for more nuanced and insightful analyses.
For example, AI can quickly process large volumes of data and identify trends that might not be immediately apparent to human analysts, enabling them to focus on strategic and interpretative tasks. Both parties complement and enhance each other in this interaction, leading to more comprehensive, nuanced, and valuable insights than either could achieve independently.
For companies, the benefits of AI are substantial. By leveraging LLMs, companies could gain deeper insights into their financial health and market positioning. AI can analyze financial statements, market trends, and even social media posts to identify potential supply chain disruptions or market opportunities. This proactive approach allows companies to mitigate risks and capitalize on opportunities more effectively. Additionally, the automation of routine financial tasks through AI can result in significant cost savings and operational efficiencies, freeing up human resources for more strategic initiatives.
AI adoption in finance also has profound implications for market efficiency. The ability of AI to rapidly process and analyze data means that information can be incorporated into asset prices more quickly than ever before. This acceleration can lead to more efficient markets, where prices reflect available information almost instantaneously. However, this increased speed also raises concerns about market volatility and the potential for flash crashes, as seen in past instances of algorithm-driven trading. Therefore, robust risk management strategies and regulatory frameworks are essential to ensure that the benefits of AI do not come at the cost of market stability.
As AI becomes more prevalent in financial decision-making, developing robust risk management strategies and regulations to ensure market stability will be crucial.
The findings of this study signal a new era of AI-driven finance, where the combination of human expertise and advanced language models like GPT-4 will likely transform the financial industry, leading to more efficient, accurate, and insightful decision-making processes. As more applications emerge, the successful integration of AI into the world of finance will require collaboration between stakeholders to maximize benefits while mitigating potential risks and unintended consequences.
Further Optimization through Fine-Tuning
One approach to enhancing the performance of AI in financial analysis is through fine-tuning large language models (LLMs) for domain-specific tasks. The potential benefits of fine-tuning LLMs for financial expertise are significant, as demonstrated by proprietary models like BloombergGPT, which outperforms similarly sized open models on financial natural language processing (NLP) tasks by significant margins.
BloombergGPT is a prime example of the power of fine-tuning a model for a specific domain. This 50-billion parameter model was trained using a mixed dataset approach, combining domain-specific financial data with general-purpose datasets. The financial data, referred to as “FinPile,” includes a comprehensive collection of English financial documents such as news, filings, press releases, and web-scraped financial documents from Bloomberg’s extensive archives. This blend ensures that BloombergGPT excels in financial tasks while maintaining strong performance on general-purpose benchmarks.
BloombergGPT enhances existing financial NLP tasks such as sentiment analysis, named entity recognition, news classification, and question-answering. It can efficiently process and analyze the vast quantities of data available on the Bloomberg Terminal, providing users with advanced insights and facilitating more informed decision-making. The model’s ability to outperform similarly sized open models on financial tasks demonstrates its robustness and reliability in handling specialized financial tasks while maintaining strong performance on general LLM benchmarks.
As foundational models like ChatGPT, Gemini, and Claude continue to improve, the potential impact of fine-tuning these increasingly powerful models for specific domains could be even more profound. Fine-tuning a more powerful base model would leverage the enhanced capabilities of the foundational model, allowing for even greater accuracy, relevance, and efficiency in domain-specific tasks.
Fine-tuning a more powerful base model would likely result in even higher accuracy and more nuanced insights. For instance, a fine-tuned GPT-4o could provide a deeper and more precise analysis of financial statements, identifying subtler patterns and trends that might be missed by less powerful models. This would further enhance the ability of financial analysts to make well-informed decisions based on comprehensive and accurate data.
The implications of fine-tuning powerful base models extend beyond traditional financial analysis. These models could be used for a wide range of applications, from risk assessment and fraud detection to automated reporting and strategic planning. By leveraging the full capabilities of advanced LLMs, organizations could gain unprecedented insights and efficiencies across various financial operations.
Fine-tuning more powerful models also enhances scalability and adaptability. As financial markets evolve and new challenges emerge, fine-tuned models can be quickly updated with new data or even constantly updated in real-time, ensuring that they remain relevant and effective. This adaptability is crucial for maintaining a competitive edge in the fast-paced world of finance.
As the power of these models rapidly increases, more innovative, tech-forward companies are embracing the technology, recognizing AI-driven solutions’ transformative potential to enhance their operations and service offerings.
PwC’s Partnership with OpenAI: The New Paradigm
The recent partnership between OpenAI and PwC exemplifies the growing industry-wide acceptance of AI in finance. OpenAI is an artificial intelligence research organization focused on developing and advancing AI technologies, known for creating sophisticated language models like GPT-4, while PwC (PricewaterhouseCoopers) is one of the world’s largest professional services firms, providing audit, assurance, consulting, and tax services to clients globally.
The recent partnership between OpenAI and PwC exemplifies the growing industry-wide acceptance of AI in finance. OpenAI is an artificial intelligence research organization focused on developing and advancing AI technologies, known for creating sophisticated language models like GPT-4, while PwC (PricewaterhouseCoopers) is one of the world’s largest professional services firms, providing audit, assurance, consulting, and tax services to clients globally.
PwC’s integration of OpenAI’s Enterprise tier into its operations demonstrates the firm’s recognition of AI’s transformative potential. This collaboration not only underscores the importance of AI in modern financial analysis but also sets a precedent for other firms to follow.
By deeply integrating AI into its operations, PwC acknowledges the significant role that advanced language models can play in transforming financial analysis. Using AI tools like ChatGPT enhances PwC’s analytical capabilities, allowing for more accurate and comprehensive insights. This integration sets a powerful example for other firms, illustrating the benefits of adopting AI-driven solutions in financial workflows.
Leveraging AI allows firms to process vast amounts of data quickly and accurately, identifying patterns and trends that might be missed by human analysts alone. AI tools can handle routine tasks such as data collection, initial analysis, and reporting, freeing up human analysts to focus on more strategic and complex evaluations. This enhancement in analytical capabilities leads to better-informed decision-making and improved financial outcomes.
AI integration streamlines financial operations by automating repetitive tasks, reducing the time and effort required for data analysis. This automation not only increases efficiency but also minimizes the risk of human error. For example, AI can automate the process of reviewing financial statements, detecting anomalies, and generating reports, which significantly speeds up the workflow and ensures accuracy.
Firms that adopt AI-driven solutions can deliver greater value to their clients through more precise and actionable insights. AI’s ability to analyze large datasets and generate detailed reports allows financial professionals to provide clients with a deeper understanding of their financial health and market positioning. This added value can enhance client satisfaction and strengthen client relationships.
The collaboration between OpenAI and PwC reflects a broader zeitgeist around AI adoption, where the combination of human expertise and advanced AI tools is becoming the norm. In today’s rapidly evolving financial landscape, understanding and integrating AI into workflows is no longer optional but essential for staying competitive. Financial professionals must embrace AI to keep pace with technological advancements and maintain their relevance in the industry.
As AI becomes more prevalent, financial professionals need to develop new skills in data science and AI literacy. This knowledge enables them to collaborate effectively with AI tools, enhancing their analytical capabilities and decision-making processes. By acquiring these skills, financial professionals can harness the full potential of AI, driving innovation and efficiency in financial analysis.
The results of this recent study, along with the growing adoption of AI-driven tools by industry leaders like PwC and Bloomberg, underscore the transformative potential of AI in finance. As AI continues to evolve and likely even further outperforms human analysts and specialized machine learning models, the financial industry must adapt by embracing collaboration, developing new skills, and implementing robust risk management strategies.
The future of finance lies in the successful integration of human expertise and advanced AI technologies, which will unlock unprecedented levels of insight, accuracy, and efficiency, ultimately leading to better outcomes for investors, companies, and markets as a whole.